Bi-Directional Relationship Inferring Network For Referring Image Segmentation
2020 IEEE/CVF CONFERENCE ON COMPUTER VISION AND PATTERN RECOGNITION (CVPR)(2020)
摘要
Most existing methods do not explicitly formulate the mutual guidance between vision and language. In this work, we propose a bi-directional relationship inferring network (BRINet) to model the dependencies of cross-modal information. In detail, the vision-guided linguistic attention is used to learn the adaptive linguistic context corresponding to each visual region. Combining with the language-guided visual attention, a bi-directional cross-modal attention module (BCAM) is built to learn the relationship between multi-modal features. Thus, the ultimate semantic context of the target object and referring expression can be represented accurately and consistently. Moreover; a gated bi-directional fusion module (GBFM) is designed to integrate the multi-level features where a gate function is used to guide the bi-directional flow of multi-level information. Extensive experiments on four benchmark datasets demonstrate that the proposed method outperforms other state-of-the-art methods under different evaluation metrics.
更多查看译文
关键词
cross-modal information,vision-guided linguistic attention,adaptive linguistic context,language-guided visual attention,bi-directional cross-modal attention module,multimodal features,referring expression,gated bi-directional fusion module,bi-directional flow,bidirectional relationship inferring network,image segmentation,mutual guidance,BRINet,ultimate semantic context,multilevel features
AI 理解论文
溯源树
样例
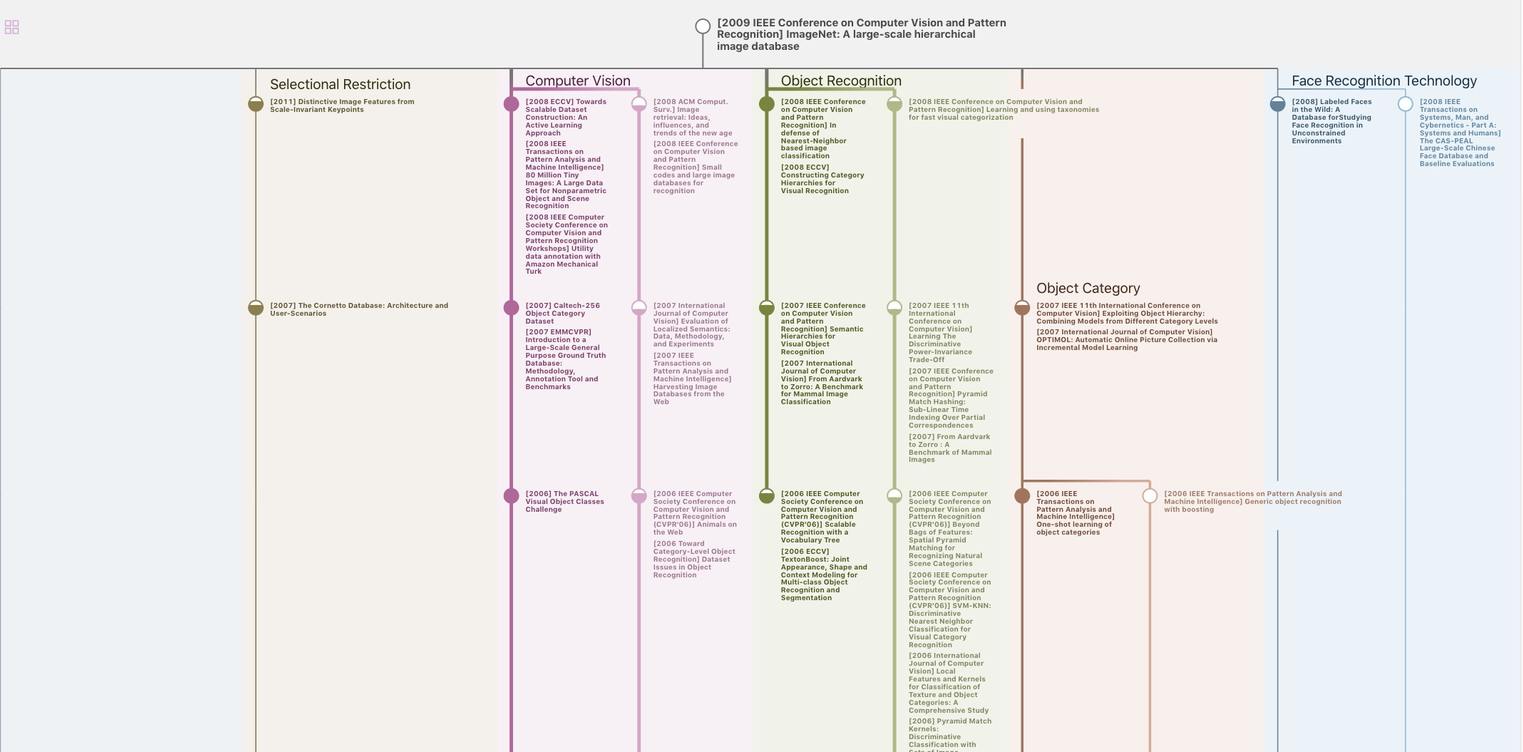
生成溯源树,研究论文发展脉络
Chat Paper
正在生成论文摘要