The Influence of Image Augmentation on Breast Lesion Classification Using Transfer Learning
2020 International Conference on Artificial Intelligence and Signal Processing (AISP)(2020)
摘要
Transfer learning and data augmentation techniques play an essential role in implementing an effective deep learning model when there is a lack of sufficient training samples. Popular pre-trained networks such as AlexNet, VGG, GoogLeNet, and ResNet showed high level of accuracy on many image classification tasks, however these networks were trained on general purpose datasets such as ImageNet which does not contain labeled images related to breast lesions. In this paper, we have fine-tuned the pre-trained VGG-19 network on breast lesions images before and after applying the augmentation methods to differentiate between benign and malignant type of cancers. Image augmentation methods are carefully chosen to further overcome the lack of breast x-ray images, improve the classification accuracy, and reduce over-fitting by generating new training samples based on the existing breast x-ray images modifications. These augmentation methods include rotation, flipping, zooming, brightness, and contrast. The performance of VGG-19 classification is compared with similar pre-trained models used in the literature such as ResNet50 and VGG-16. Our approach surpassed the previous approaches with classification accuracy, sensitivity, specificity, and AUC of 90.4%, 94.01%, 85.9%, and 95.9% respectively.
更多查看译文
关键词
transfer learning,VGG-19,image augmentation,breast lesion classification,mammograms
AI 理解论文
溯源树
样例
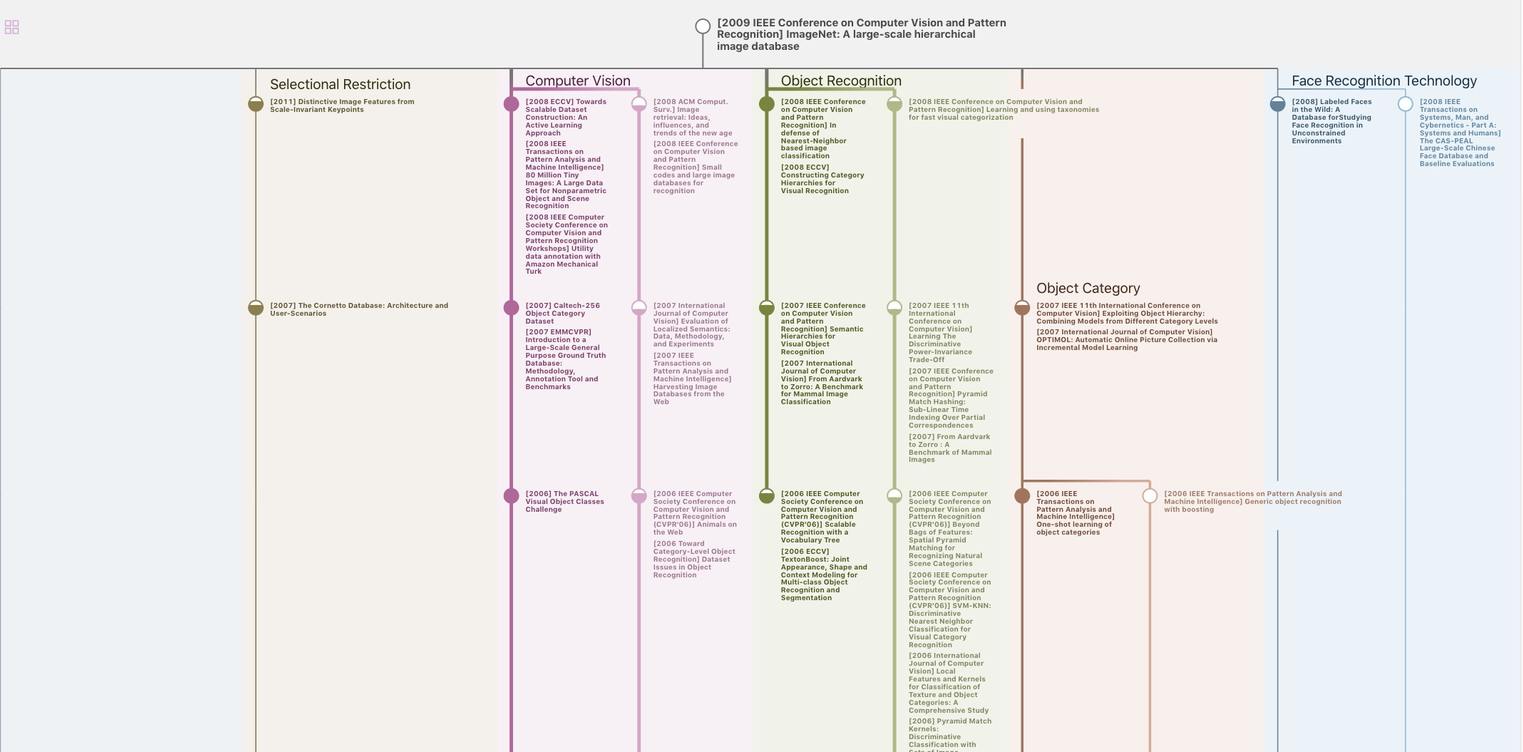
生成溯源树,研究论文发展脉络
Chat Paper
正在生成论文摘要