Neural Network Interatomic Potential for Predicting the Formation of Planar Defect in Nanocrystal
JOURNAL OF PHYSICAL CHEMISTRY C(2020)
摘要
Recent advances in the development of interatomic potential using neural networks have proven that its accuracy reaches that of first-principles calculations but with considerably reduced computational cost. In this study, we successfully implement a neural network to construct the interatomic potential of the ZnSe structure by training its potential energy surface results obtained from density functional theory (DFT) calculations. The developed potential is used for molecular dynamics simulations and its accuracy lies within an error of 6% on average from the DFT results for predicting the total energy on pristine and defective bulk structures, slab, and cluster structures of ZnSe. The prediction accuracy is also demonstrated considering the lattice constant and mechanical properties of the pristine bulk structure. To demonstrate its transferability further, a neural network potential is constructed to predict the formation energy of planar defects (stacking fault and twin boundary) in the slab and the nanocrystal structures, and it precisely reproduces the order of stability for each defect type. These results reveal that the neural-network-based interatomic potential can be used to revolutionize atomistic simulations by significantly saving the computation time while maintaining accuracy comparable to that of the DFT.
更多查看译文
AI 理解论文
溯源树
样例
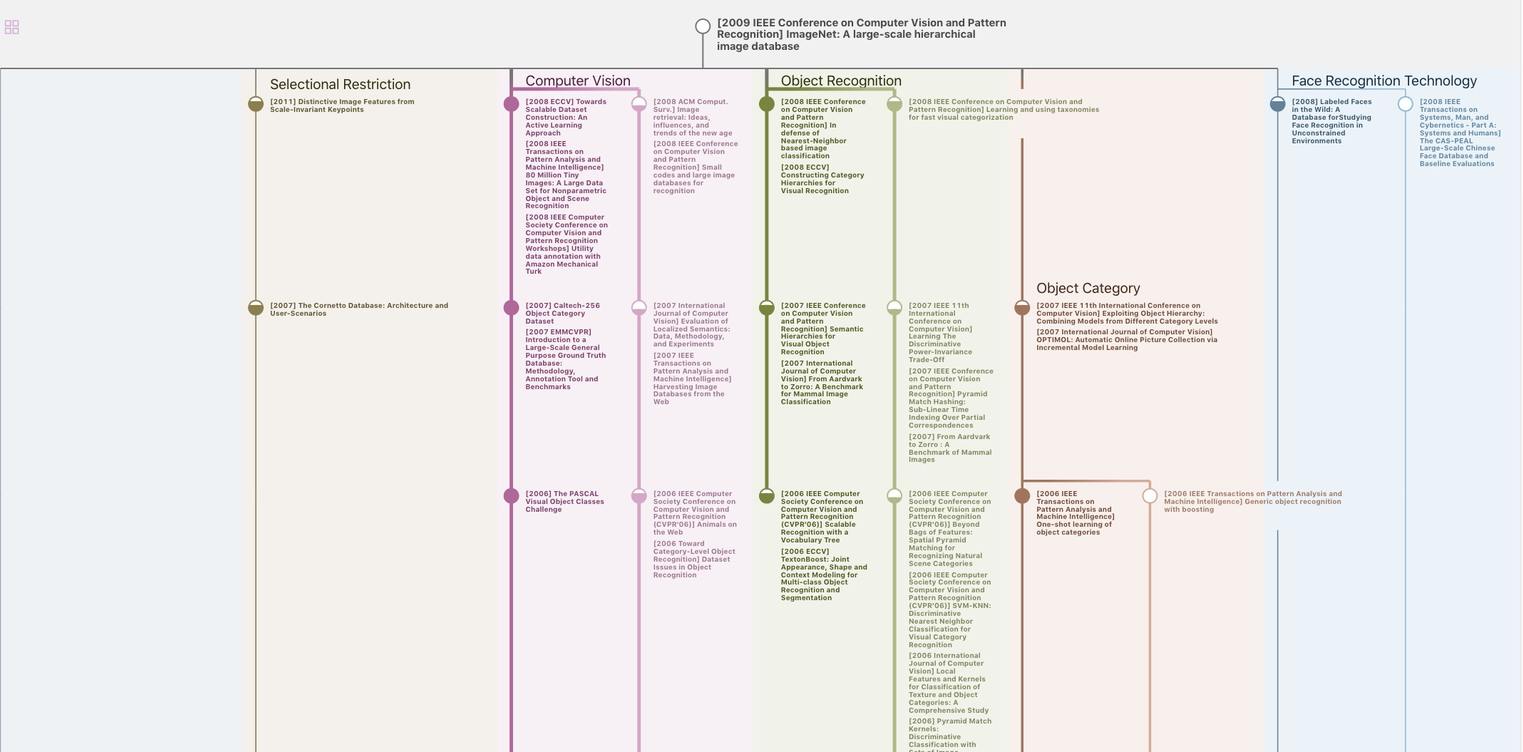
生成溯源树,研究论文发展脉络
Chat Paper
正在生成论文摘要