Combining Local and Global Features into a Siamese Network for Sentence Similarity
IEEE ACCESS(2020)
摘要
Sentence similarity is widely used in various natural language tasks such as natural language inference, paraphrase identification, and question answering. However, a variety of linguistic expressions and ambiguities of words in sentences make it difficult to measure sentence similarity. Many studies show that using local features or global features of a sentence will produce satisfactory sentence representations that can be utilized to measure sentence similarity. Local features reflect the relationships of adjacent words for each sentence and the sequence information of a sentence are usually expressed by global features. However, local features lack abilities to capture sequence information while a small amount of extracted global features is not enough to produce sentence representations with good qualities. In this paper, we propose A Hybrid Model combining Local and Global features into a Siamese Network (HM-LGSN) for sentence similarity calculation. We first propose a new convolution neural network architecture called group convolution neural network to extract the most representative local features (or word semantic features). Then we combine these new features with pre-trained embeddings of words as input to the Bidirectional Gated Recurrent Units to extract global features of sentences. Finally, we select the global features to form sentence representations and calculate sentence similarity through Manhattan distance. The experimental results on SICK, MSRVID, STS-B datasets show that the accuracy of our proposed model is significantly improved by combining local features and global features.
更多查看译文
关键词
Feature extraction,Semantics,Convolution,Neural networks,Information filters,Task analysis,Sentence similarity,semantic representation,siamese network,local feature,global feature
AI 理解论文
溯源树
样例
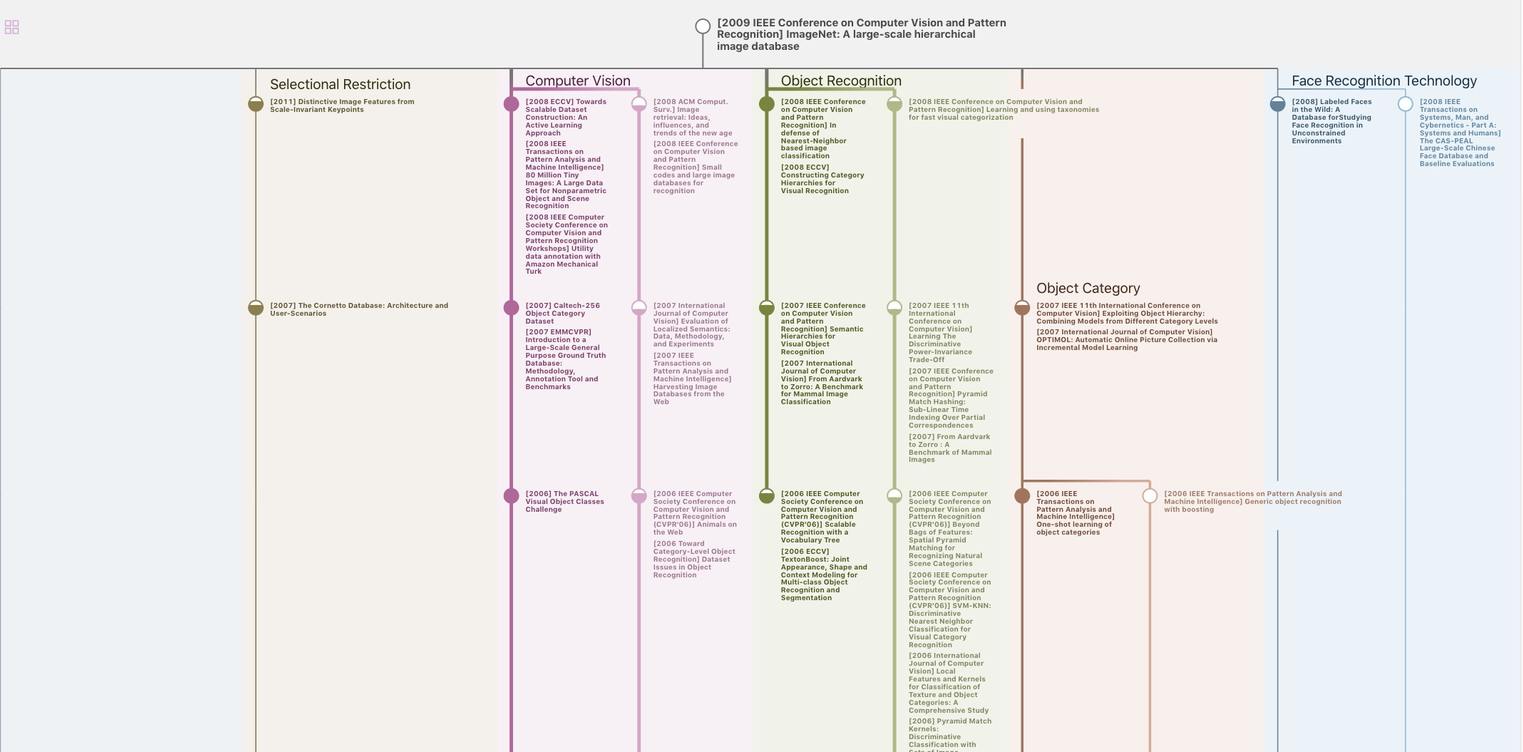
生成溯源树,研究论文发展脉络
Chat Paper
正在生成论文摘要