Hierarchical Temporal Modeling With Mutual Distance Matching for Video Based Person Re-Identification
IEEE Transactions on Circuits and Systems for Video Technology(2021)
摘要
Comparing to image-based person re-identification (re-ID) problems, video-based person re-ID can take advantage of more cues from appearance and temporal information, and therefore receives widespread attention recently. However, due to the different pose, occlusion, misalignment and multi-granularity in video sequences, those consequent inter-sequence variations and intra-sequence variations, inevitably makes the feature learning and matching in videos more difficult. Under this circumstance, it is necessary to design an effective discriminative representation learning mechanism, as well as a matching solution, to tackle these variations in video-based person re-ID. To this end, this paper introduces a multi-granularity temporal convolution network and a mutual distance matching measurement, aiming at alleviating the intra-sequence variation and the inter-sequence variation, respectively. Particularly, in the feature learning stage, we model different temporal granularities by hierarchically stacking temporal convolution blocks with different dilation factors. In the feature matching stage, we propose a clip-level probe-gallery mutual distance measurement and consider the most convincing clip pairs by top-k selection. We validate that our proposed method can achieve state-of-the-art results on three video-based person re-ID benchmarks, more than that, we conduct extensive ablation study to demonstrate conciseness and effectiveness of our method in video re-ID tasks.
更多查看译文
关键词
Video-based person re-identification,temporal modeling,temporal convolutional networks,probe-gallery mutual distance
AI 理解论文
溯源树
样例
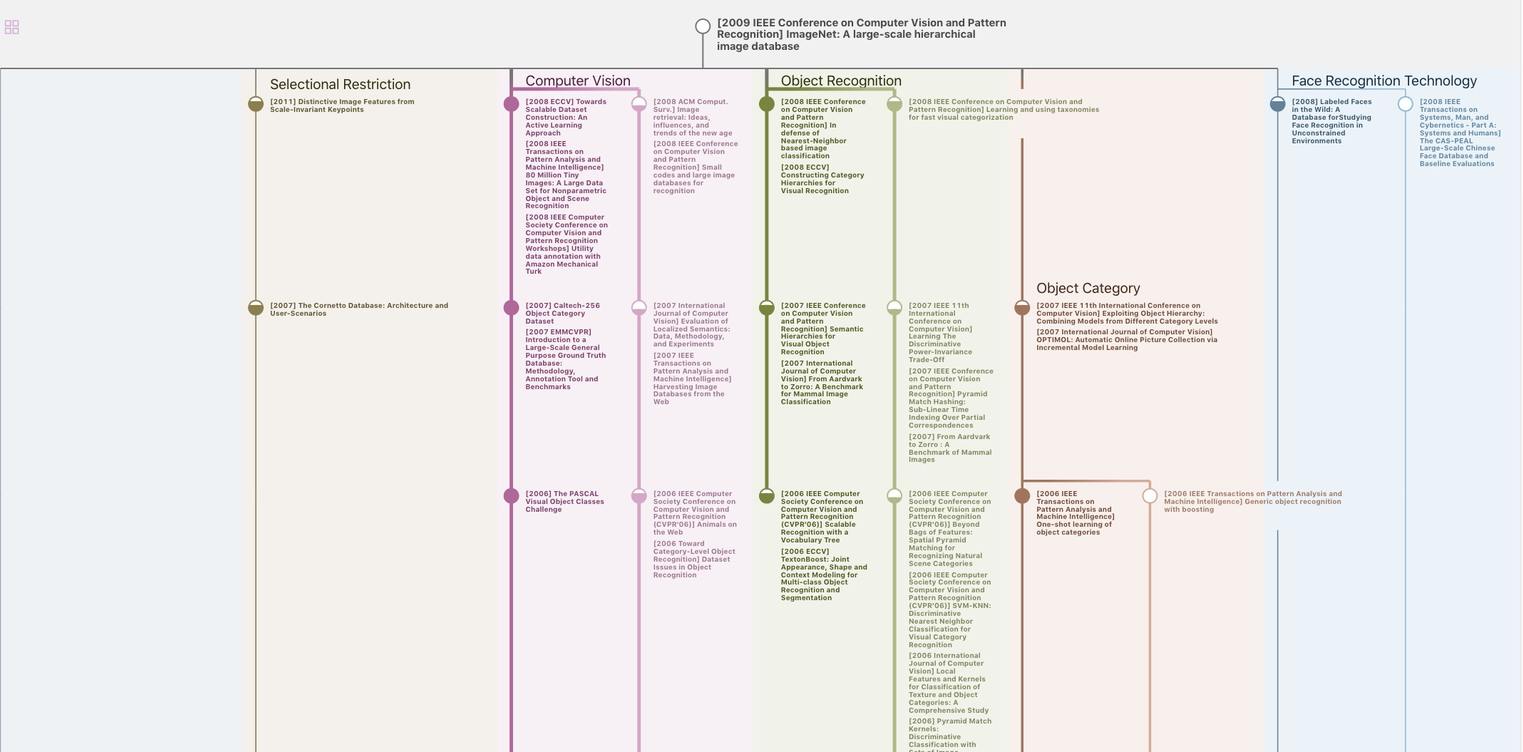
生成溯源树,研究论文发展脉络
Chat Paper
正在生成论文摘要