Lstm-Based One-Pass Decoder For Low-Latency Streaming
2020 IEEE INTERNATIONAL CONFERENCE ON ACOUSTICS, SPEECH, AND SIGNAL PROCESSING(2020)
摘要
Current state-of-the-art models based on Long-Short Term Memory (LSTM) networks have been extensively used in ASR to improve performance. However, using LSTMs under a streaming setup is not straightforward due to real-time constraints. In this paper we present a novel streaming decoder that includes a bidirectional LSTM acoustic model as well as an unidirectional LSTM language model to perform the decoding efficiently while keeping the performance comparable to that of an off-line setup. We perform a one-pass decoding using a sliding window scheme for a bidirectional LSTM acoustic model and an LSTM language model. This has been implemented and assessed under a pure streaming setup, and deployed into our production systems. We report WER and latency figures for the well-known LibriSpeech and TED-LIUM tasks, obtaining competitive WER results with low-latency responses.
更多查看译文
关键词
automatic speech recognition, streaming, decoding, acoustic modeling, language modeling
AI 理解论文
溯源树
样例
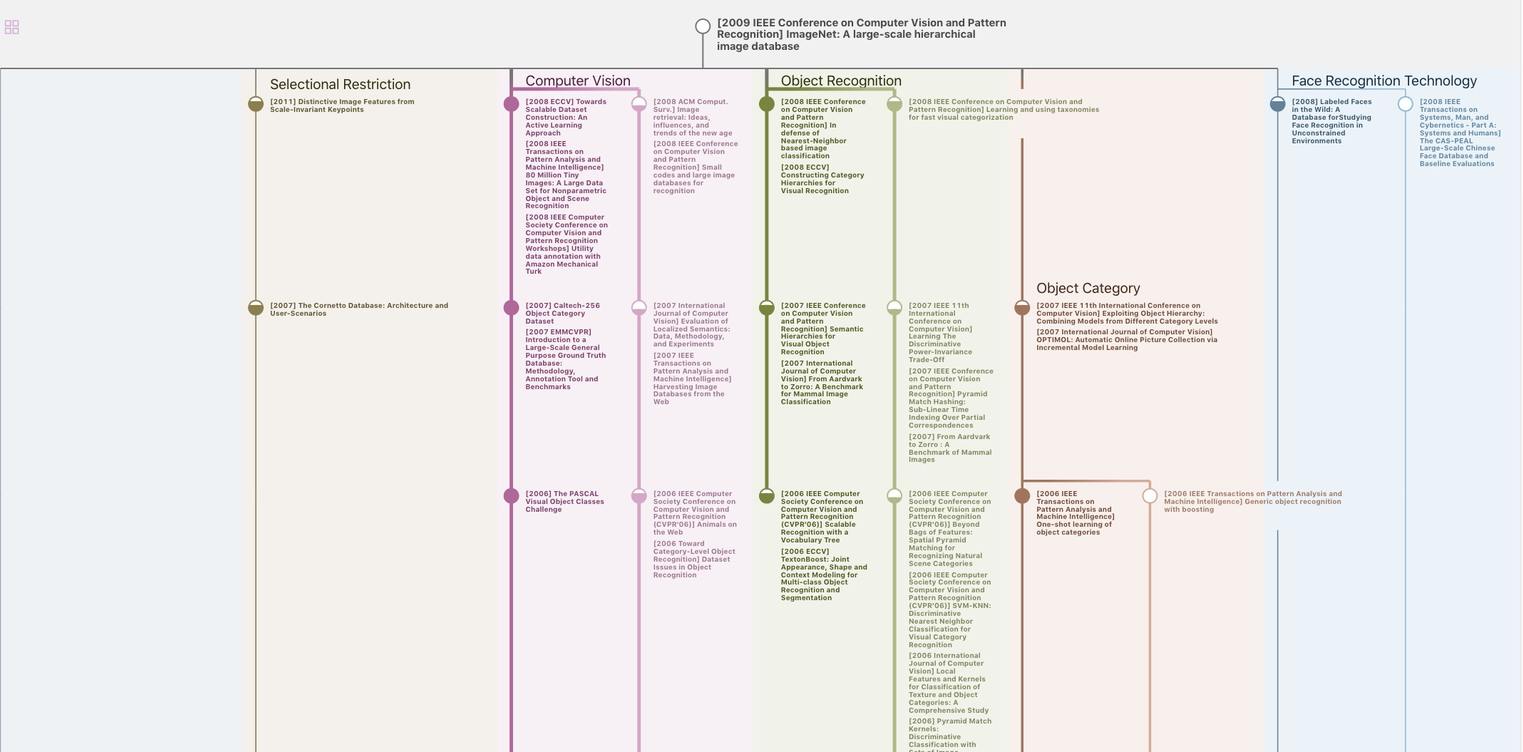
生成溯源树,研究论文发展脉络
Chat Paper
正在生成论文摘要