Compressed Sensing Based Channel Estimation and Open-loop Training Design for Hybrid Analog-digital Massive MIMO Systems
ICASSP(2020)
摘要
Channel estimation in hybrid analog-digital massive MIMO systems is a challenging problem due to the high channel dimension, low signal-to-noise ratio before beamforming, and reduced number of radio-frequency chains. Compressed sensing based algorithms have been adopted to address these challenges by leveraging the sparse nature of millimeter-wave MIMO channels. In compressed sensing-based methods, the training vectors should be designed carefully to guarantee recoverability. Although using random vectors has an overwhelming recoverability guarantee, it has been recently shown that an optimized update, which could be obtained so that the mutual coherence of the resulting sensing matrix is minimized, can improve the recoverability guarantee. In this paper, we propose an open-loop hybrid analog-digital beam-training framework, where a given sensing matrix is decomposed into analog and digital beamformers. The given sensing matrix can be designed efficiently offline to reduce computational complexity. Simulation results show that the proposed training method achieves a lower mutual coherence and an improved channel estimation performance than the other benchmark methods.
更多查看译文
关键词
Hybrid analog-digital,massive MIMO,compressed sensing,open-loop training design,mutual coherence.
AI 理解论文
溯源树
样例
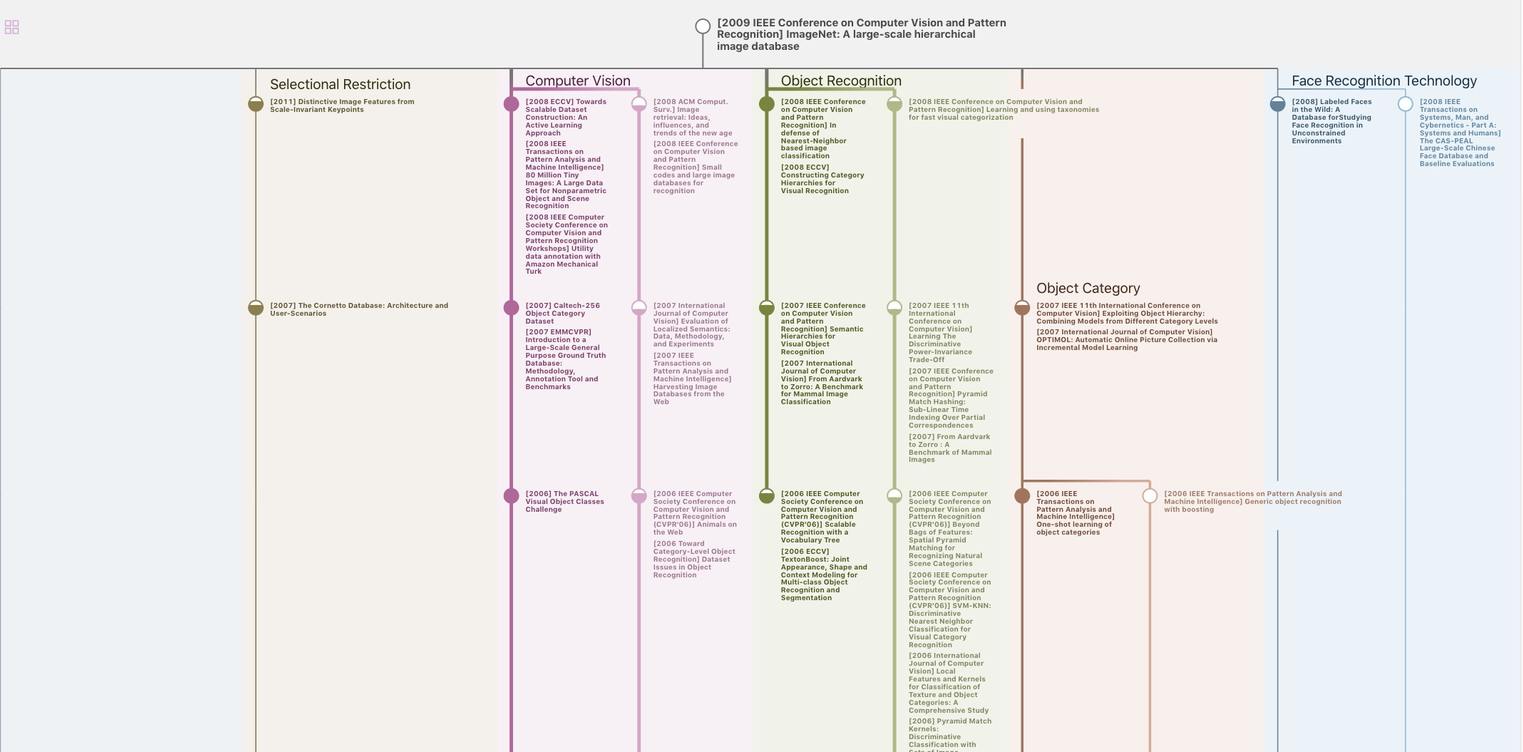
生成溯源树,研究论文发展脉络
Chat Paper
正在生成论文摘要