A semi-automated annotation algorithm based on weakly supervised learning for medical images
Biocybernetics and Biomedical Engineering(2020)
摘要
For medical image recognition, deep learning requires a massive training set, while annotation work is a tedious and time-consuming process because of the high technical threshold. Furthermore, it is difficult to guarantee annotation accuracy due to the knowledge, skills, and status of the annotator. In this research, we propose a semi-automated annotation model based on weakly supervised learning. Moreover, a target-level annotation method is proposed based on weakly supervised learning that is guided by machine learning. The machine learning method is used to screen the regions of interest (RoIs), whose semantic feature vectors are extracted by the deep learning method. Then, the machine learning method is used to cluster them, and the RoIs are finally classified and labeled by a distance comparison. Therefore, this model achieves target-level semi-automated annotation by only using image-level annotations. We applied this method to ultrasound imaging of thyroid papillary carcinoma. The experiments demonstrate the potential of this new methodology to reduce the workload for pathologists and increase the objectivity of diagnoses. We find that 89.8% of papillary thyroid carcinoma regions can be detected automatically, while 82.6% of benign and normal tissue can be excluded without the use of any additional immunohistochemical markers or human intervention.
更多查看译文
关键词
Deep learning,Machine learning,Target-level annotation,Weakly supervised learning,Thyroid carcinoma
AI 理解论文
溯源树
样例
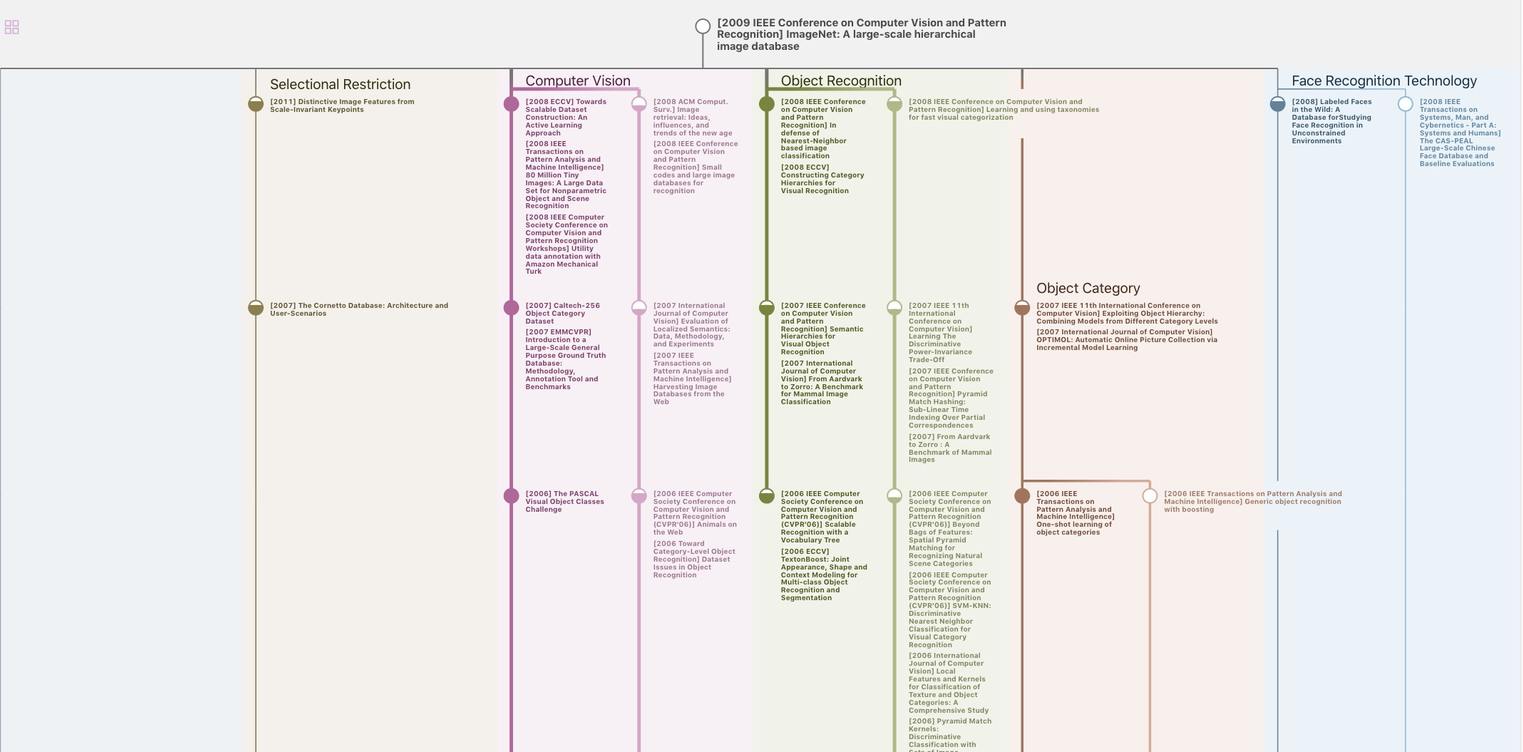
生成溯源树,研究论文发展脉络
Chat Paper
正在生成论文摘要