Next item recommendation with self-attentive metric learning
Thirty-Third AAAI Conference on Artificial Intelligence(2019)
摘要
In this paper, we propose a novel sequence-aware recommendation model. Our model utilizes self-attention mechanism to infer the item-item relationship from user’s historical interactions. With self-attention, it is able to estimate the relative weights of each item in user interaction trajectories to learn better representations for user’s transient interests. The model is finally trained in a metric learning framework, taking both local and global user intentions into consideration. Experiments on a wide range of datasets on different domains demonstrate that our approach outperforms the state-of-theart by a wide margin.
更多查看译文
AI 理解论文
溯源树
样例
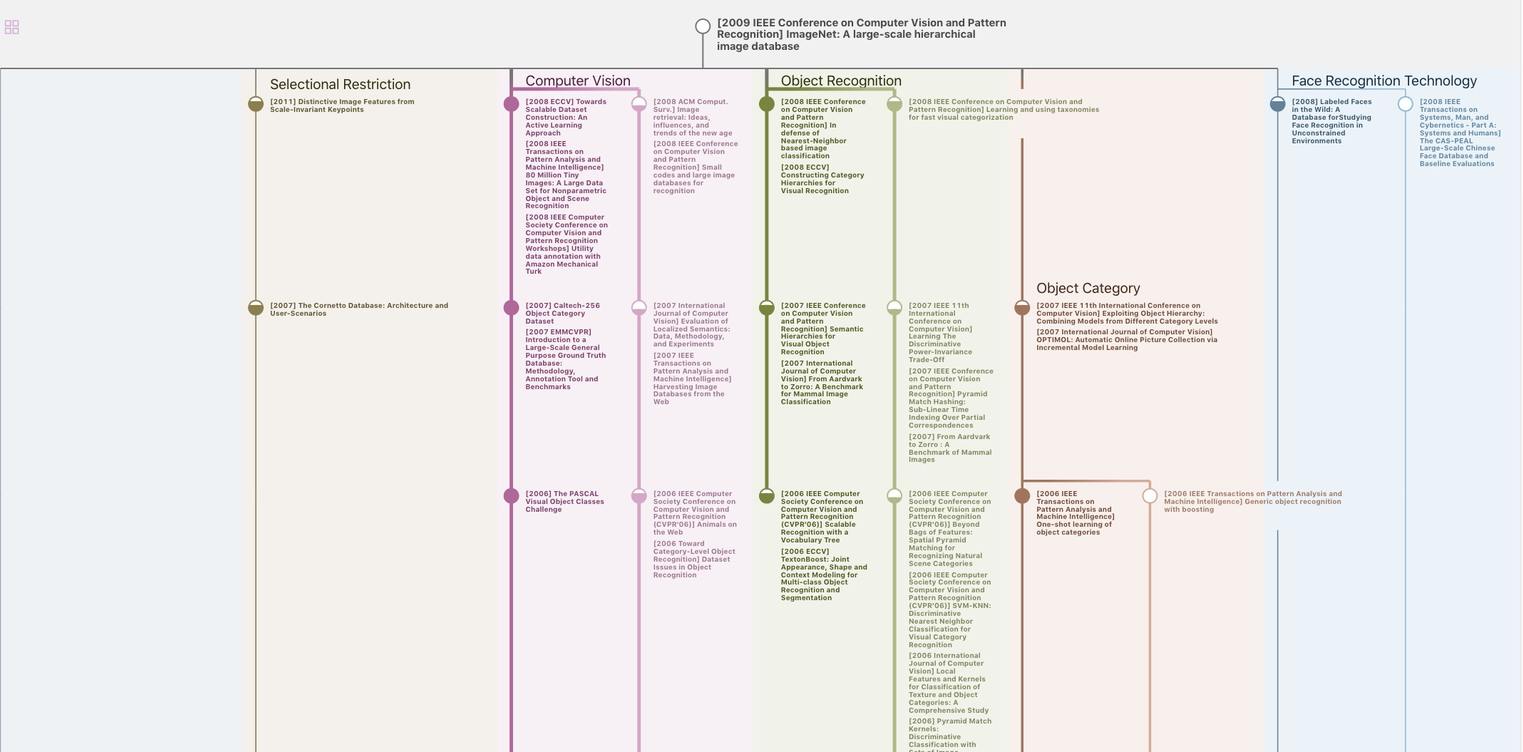
生成溯源树,研究论文发展脉络
Chat Paper
正在生成论文摘要