MULTISTREAM CNN FOR ROBUST ACOUSTIC MODELING
2021 IEEE INTERNATIONAL CONFERENCE ON ACOUSTICS, SPEECH AND SIGNAL PROCESSING (ICASSP 2021)(2021)
摘要
This paper proposes multistream CNN, a novel neural network architecture for robust acoustic modeling in speech recognition tasks. The proposed architecture processes input speech with diverse temporal resolutions by applying different dilation rates to convolutional neural networks across multiple streams to achieve the robustness. The dilation rates are selected from the multiples of a sub-sampling rate of 3 frames. Each stream stacks TDNN-F layers (a variant of 1D CNN), and output embedding vectors from the streams are concatenated then projected to the final layer. We validate the effectiveness of the proposed multistream CNN architecture by showing consistent improvements against Kaldi's best TDNN-F model across various data sets. Multistream CNN improves the WER of the test-other set in the LibriSpeech corpus by 12% (relative). On custom data from ASAPP's production ASR system for a contact center, it records a relative WER improvement of 11% for customer channel audio to prove its robustness to data in the wild. In terms of real-time factor, multistream CNN outperforms the baseline TDNN-F by 15%, which also suggests its practicality on production systems. When combined with self-attentive SRU LM rescoring, multistream CNN contributes for ASAPP to achieve the best WER of 1.75% on test-clean in LibriSpeech.
更多查看译文
关键词
Multistream CNN, robust acoustic modeling, speech recognition
AI 理解论文
溯源树
样例
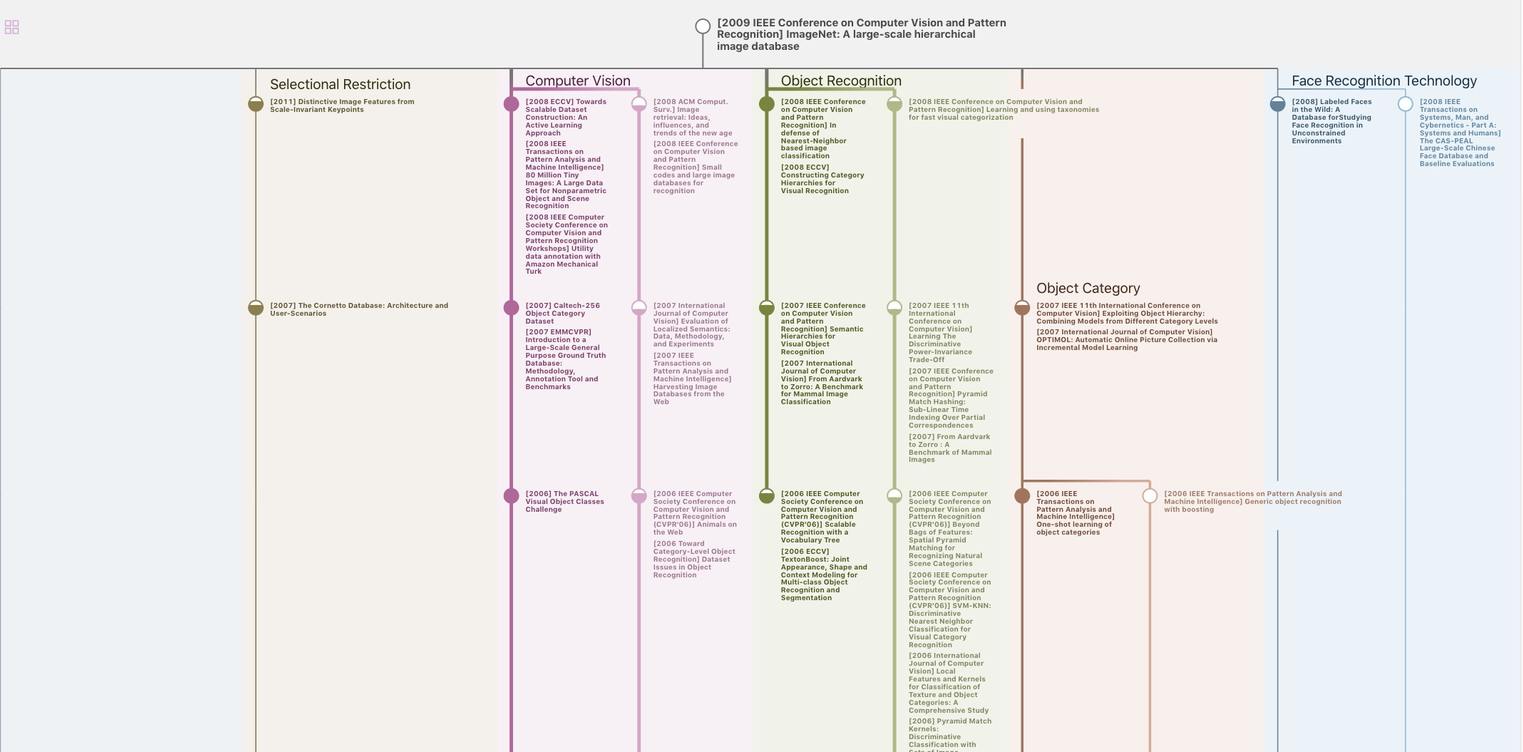
生成溯源树,研究论文发展脉络
Chat Paper
正在生成论文摘要