Instance Segmentation Of Benthic Scale Worms At A Hydrothermal Site
2020 IEEE WINTER CONFERENCE ON APPLICATIONS OF COMPUTER VISION (WACV)(2020)
摘要
Subsea hydrothermal vents, typically existing at water depths below natural light penetration, contain diverse and unique macrofaunal environments. Traditionally, long-term ecological observation has been difficult as the extreme depth, temperature and pressure make in situ video surveys challenging. However, the introduction of subsea cabled arrays has allowed for the long time series collection of high definition imagery from these vents. To study the benthic hydrothermal vent environment, we propose an inference pipeline consisting of a U-Net followed by VGG-16 CNN to perform instance segmentation of scale worms, a specific macrofaunal family. The developed pipeline exhibits an average precision (AP) of 0.671 AP@[0.5], despite the difficult camouflaged imagery and low training data inputs. We further explore full pipeline training data requirements, as the dynamic scene in question requires the pipeline to be re-trained on an approximately monthly basis for effective segmentation. We find that the VGG-16 CNN portion of the pipeline is typically more sensitive to training data variation than the U-Net portion.
更多查看译文
关键词
macrofaunal environments,in situ video surveys,camouflaged imagery,benthic scale worms,training data variation,VGG-16 CNN portion,pipeline training data requirements,low training data inputs,specific macrofaunal family,instance segmentation,inference pipeline,benthic hydrothermal vent environment,high definition imagery,long time series collection,long-term ecological observation,diverse environments,natural light penetration,water depths,subsea hydrothermal vents,hydrothermal site
AI 理解论文
溯源树
样例
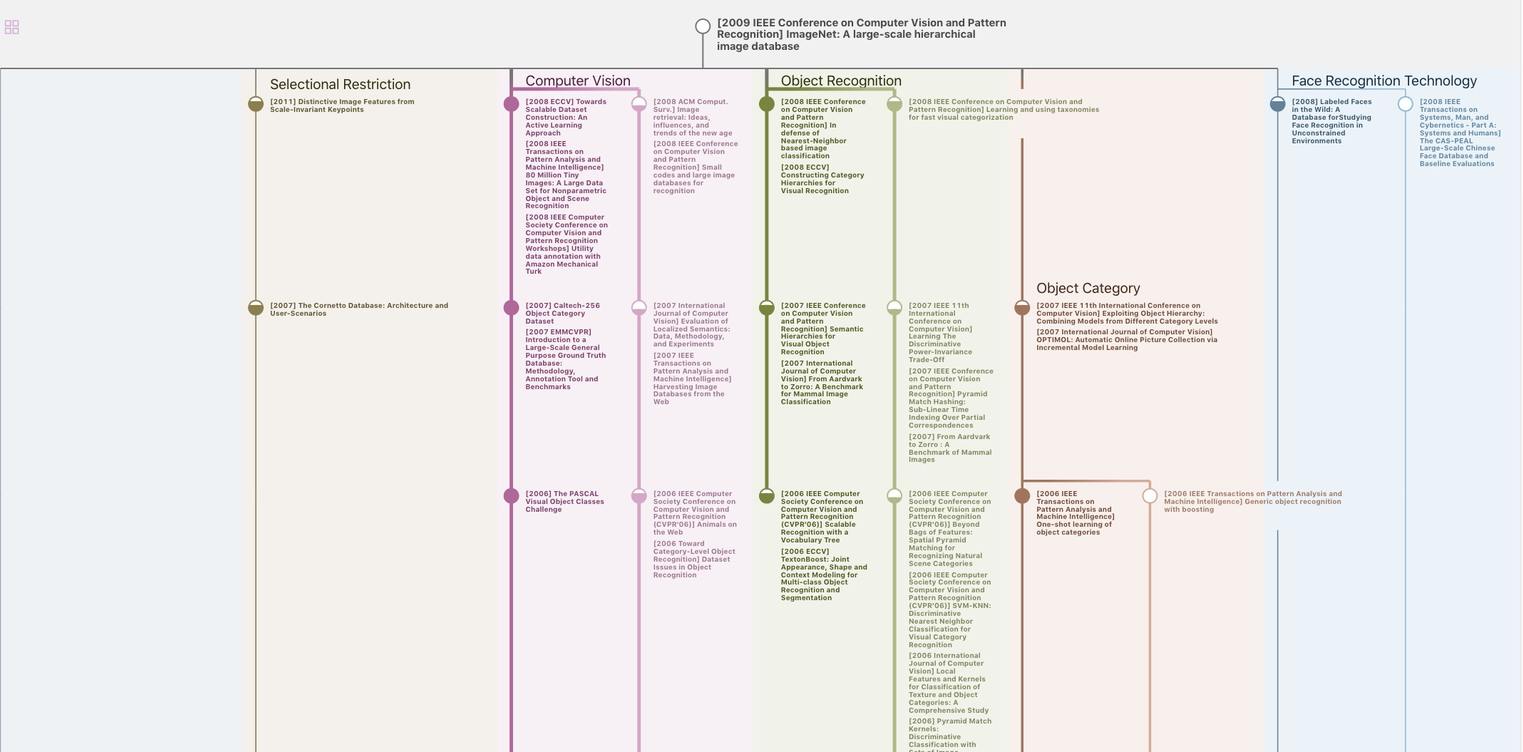
生成溯源树,研究论文发展脉络
Chat Paper
正在生成论文摘要