Variational Neural Machine Translation with Normalizing Flows
ACL(2020)
摘要
Variational Neural Machine Translation (VNMT) is an attractive framework for modeling the generation of target translations, conditioned not only on the source sentence but also on some latent random variables. The latent variable modeling may introduce useful statistical dependencies that can improve translation accuracy. Unfortunately, learning informative latent variables is non-trivial, as the latent space can be prohibitively large, and the latent codes are prone to be ignored by many translation models at training time. Previous works impose strong assumptions on the distribution of the latent code and limit the choice of the NMT architecture. In this paper, we propose to apply the VNMT framework to the state-of-the-art Transformer and introduce a more flexible approximate posterior based on normalizing flows. We demonstrate the efficacy of our proposal under both in-domain and out-of-domain conditions, significantly outperforming strong baselines.
更多查看译文
关键词
normalizing flows,translation
AI 理解论文
溯源树
样例
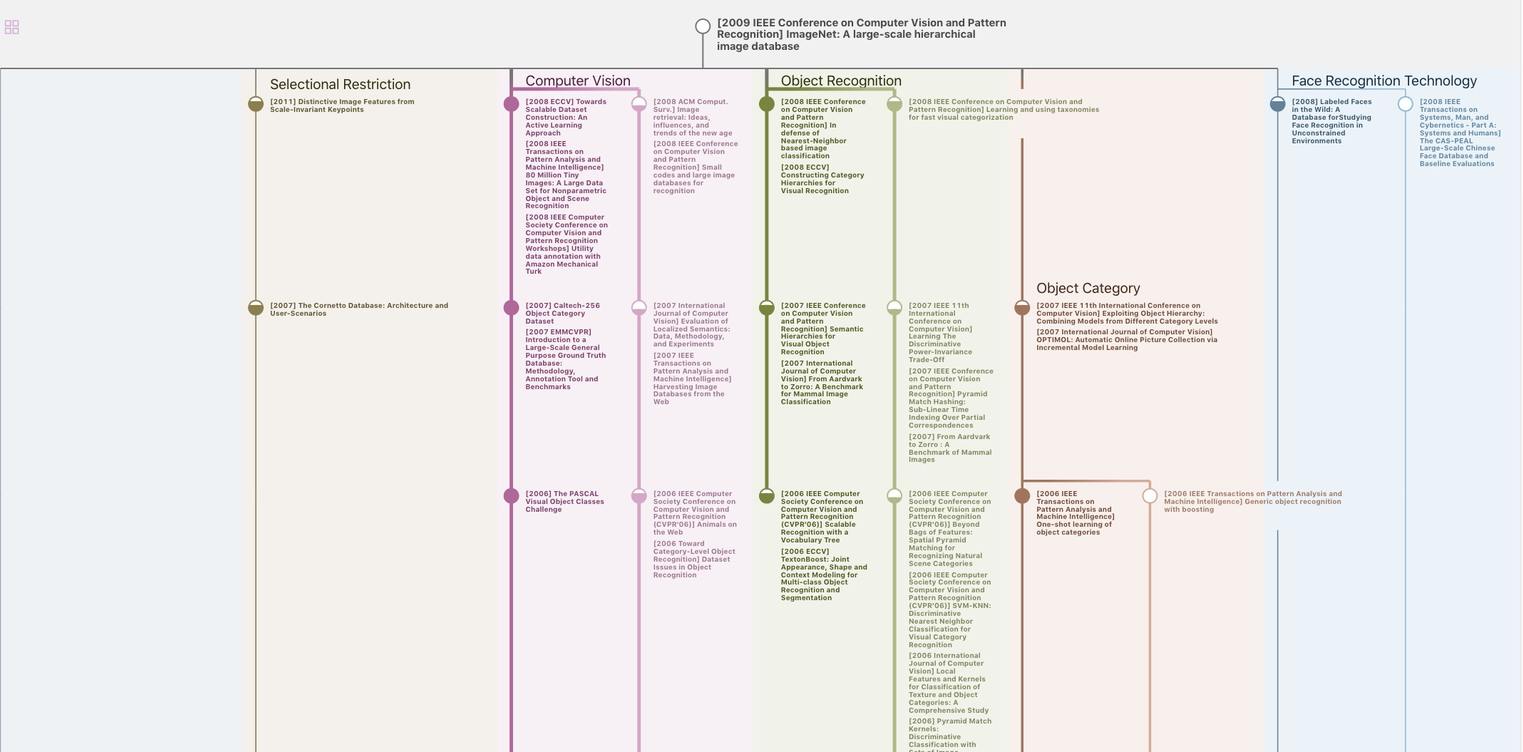
生成溯源树,研究论文发展脉络
Chat Paper
正在生成论文摘要