Dynamic Sampling Strategies For Multi-Task Reading Comprehension
58TH ANNUAL MEETING OF THE ASSOCIATION FOR COMPUTATIONAL LINGUISTICS (ACL 2020)(2020)
摘要
Building general reading comprehension systems, capable of solving multiple datasets at the same time, is a recent aspirational goal in the research community. Prior work has focused on model architectures or generalization to held out datasets, and largely passed over the particulars of the multi-task learning set up. We show that a simple dynamic sampling strategy, selecting instances for training proportional to the multi-task model's current performance on a dataset relative to its single-task performance, gives substantive gains over prior multi-task sampling strategies, mitigating the catastrophic forgetting that is common in multi-task learning. We also demonstrate that allowing instances of different tasks to be interleaved as much as possible between each epoch and batch has a clear benefit in multitask performance over forcing task homogeneity at the epoch or batch level. Our final model shows greatly increased performance over the best model on ORB, a recently-released multitask reading comprehension benchmark.
更多查看译文
AI 理解论文
溯源树
样例
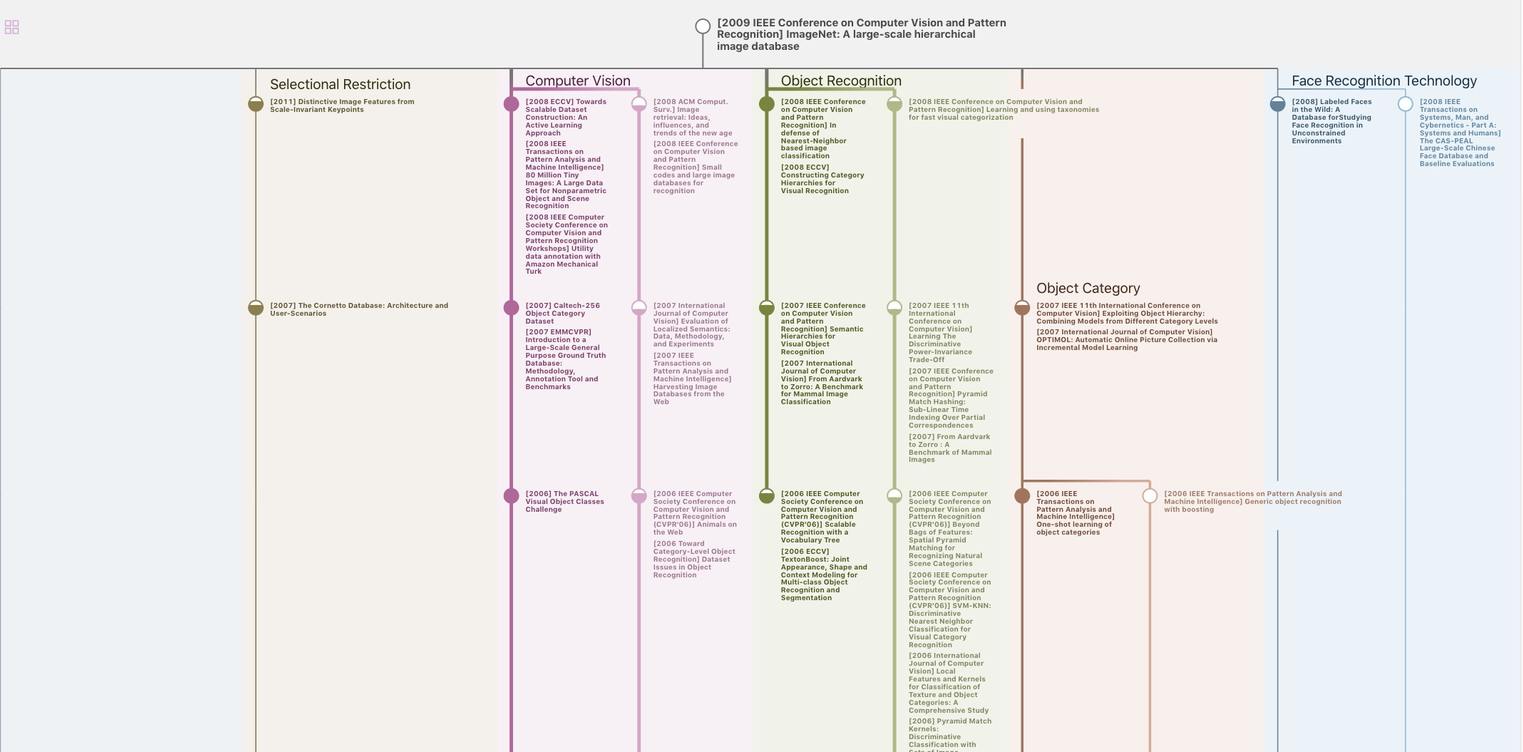
生成溯源树,研究论文发展脉络
Chat Paper
正在生成论文摘要