Learning To Herd Agents Amongst Obstacles: Training Robust Shepherding Behaviors Using Deep Reinforcement Learning
IEEE ROBOTICS AND AUTOMATION LETTERS(2021)
摘要
Robotic shepherding problem considers the control and navigation of a group of coherent agents (e.g., a flock of bird or a fleet of drones) through the motion of an external robot, called shepherd. Machine learning based methods have successfully solved this problem in an environment with no obstacles. Rule-based methods, on the other hand, can handle more complex scenarios in which environments are cluttered with obstacles and allow multiple shepherds to work collaboratively. However, these rule-based methods are fragile due to the difficulty in defining a comprehensive set of behavioral rules. To overcome these limitations, we propose the first known learning-based method that can herd agents amongst obstacles. By using deep reinforcement learning techniques combined with the probabilistic roadmaps, we train a shepherding model using noisy but controlled environmental and behavioral parameters. Our experimental results show that the trained shepherding controller is robust, namely, it is insensitive to the uncertainties originated from either the group behavioral models or from environments with a small of path homotopy classes. Consequently, the proposed method has a higher success rate, shorter completion time and path length than the rule-based behavioral methods have. These advantages are particularly prominent in more challenging scenarios involving more difficult groups and strenuous passages.
更多查看译文
关键词
Motion and path planning, reinforcement learning, task and motion planning
AI 理解论文
溯源树
样例
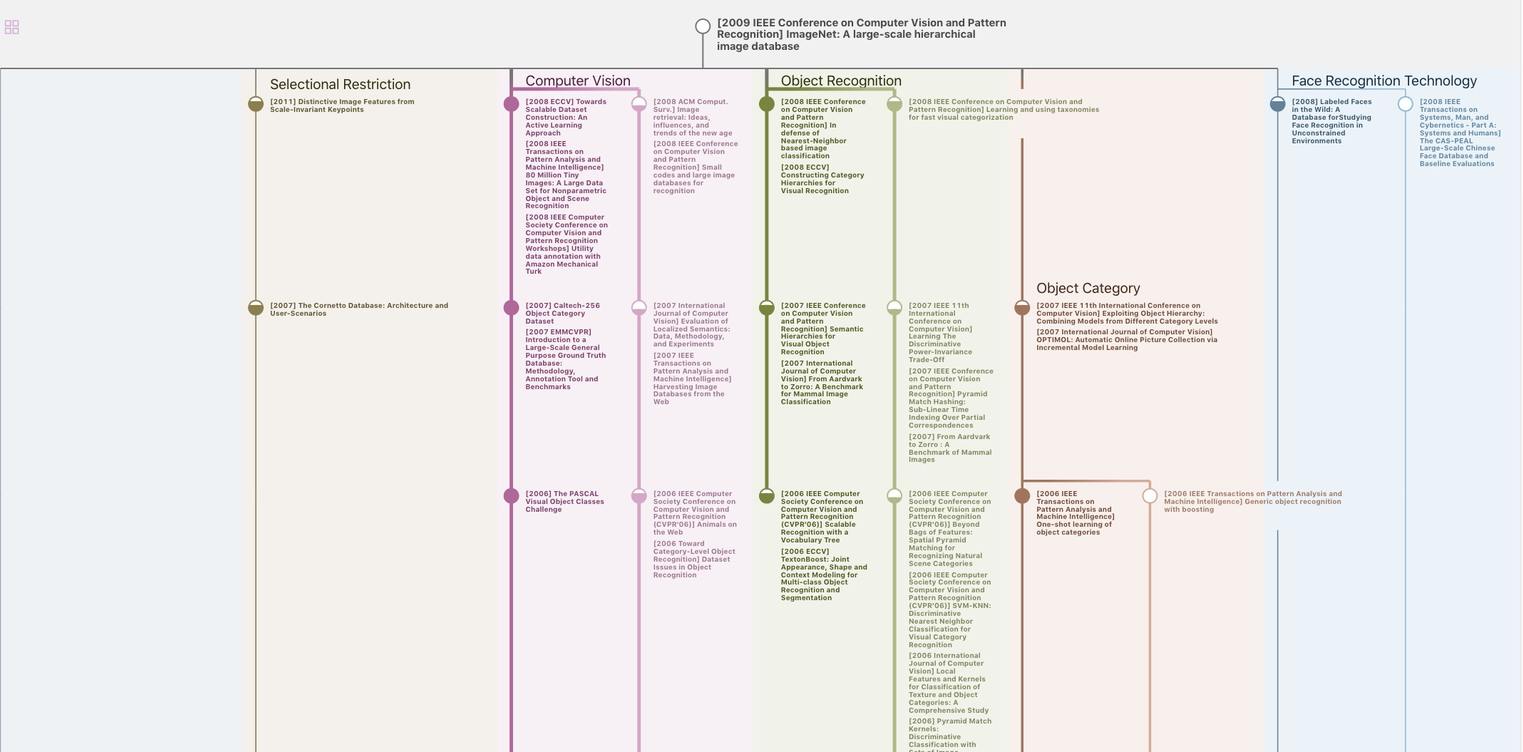
生成溯源树,研究论文发展脉络
Chat Paper
正在生成论文摘要