An Analysis Of The Adaptation Speed Of Causal Models
24TH INTERNATIONAL CONFERENCE ON ARTIFICIAL INTELLIGENCE AND STATISTICS (AISTATS)(2021)
摘要
Consider a collection of datasets generated by unknown interventions on an unknown structural causal model G. Recently, Bengio et al. (2020) conjectured that among all candidate models, G is the fastest to adapt from one dataset to another, along with promising experiments. Indeed, intuitively G has less mechanisms to adapt, but this justification is incomplete. Our contribution is a more thorough analysis of this hypothesis. We investigate the adaptation speed of cause-effect SCMs. Using convergence rates from stochastic optimization, we justify that a relevant proxy for adaptation speed is distance in parameter space after intervention. Applying this proxy to categorical and normal causeeffect models, we show two results. When the intervention is on the cause variable, the SCM with the correct causal direction is advantaged by a large factor. When the intervention is on the effect variable, we characterize the relative adaptation speed. Surprisingly, we find situations where the anticausal model is advantaged, falsifying the initial hypothesis.
更多查看译文
关键词
causal models,adaptation speed
AI 理解论文
溯源树
样例
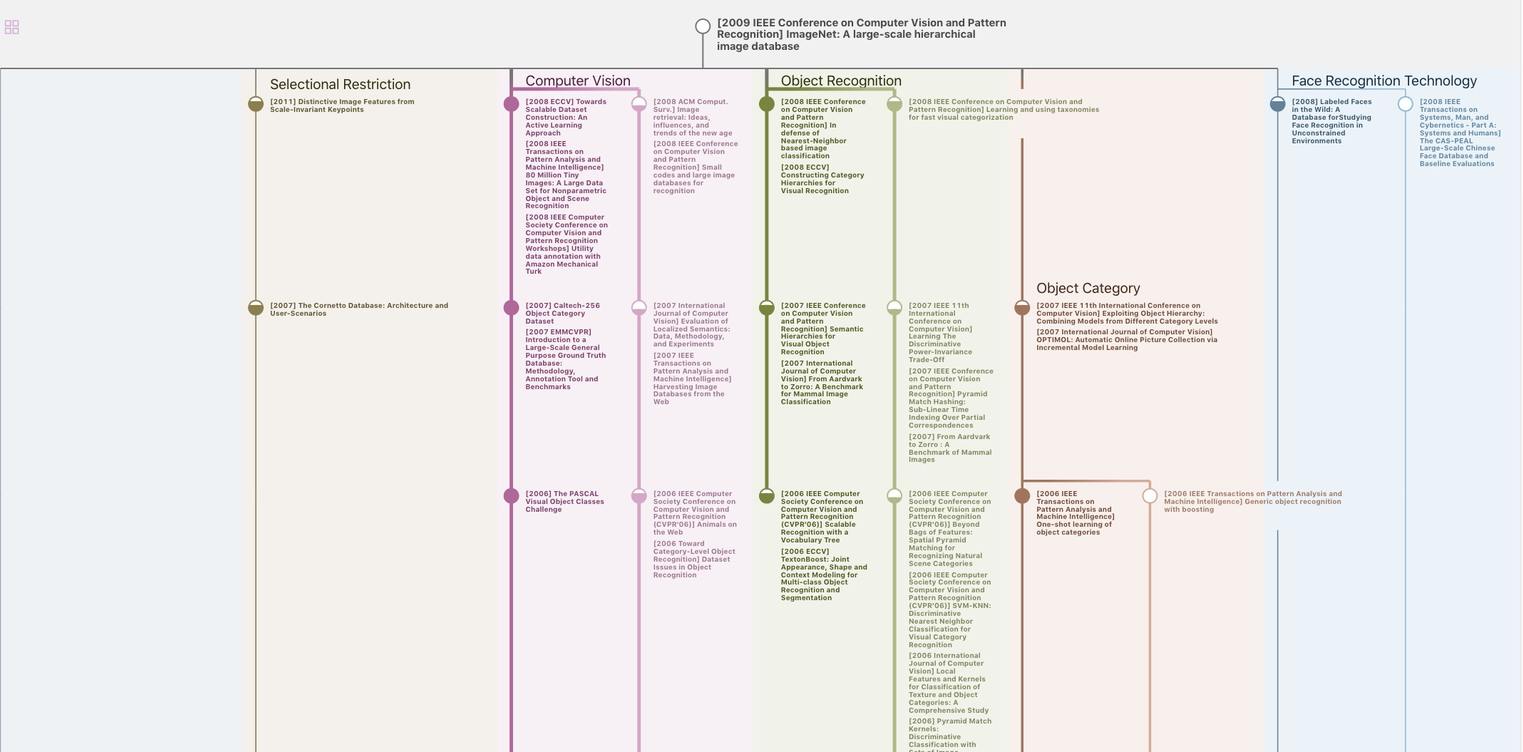
生成溯源树,研究论文发展脉络
Chat Paper
正在生成论文摘要