Sparse Mixture of Local Experts for Efficient Speech Enhancement
INTERSPEECH(2020)
摘要
In this paper, we investigate a deep learning approach for speech denoising through an efficient ensemble of specialist neural networks. By splitting up the speech denoising task into non-overlapping subproblems and introducing a classifier, we are able to improve denoising performance while also reducing computational complexity. More specifically, the proposed model incorporates a gating network which assigns noisy speech signals to an appropriate specialist network based on either speech degradation level or speaker gender. In our experiments, a baseline recurrent network is compared against an ensemble of similarly-designed smaller recurrent networks regulated by the auxiliary gating network. Using stochastically generated batches from a large noisy speech corpus, the proposed model learns to estimate a time-frequency masking matrix based on the magnitude spectrogram of an input mixture signal. Both baseline and specialist networks are trained to estimate the ideal ratio mask, while the gating network is trained to perform subproblem classification. Our findings demonstrate that a fine-tuned ensemble network is able to exceed the speech denoising capabilities of a generalist network, doing so with fewer model parameters.
更多查看译文
关键词
speech enhancement, adaptive mixture of local experts, model selection, neural network compression
AI 理解论文
溯源树
样例
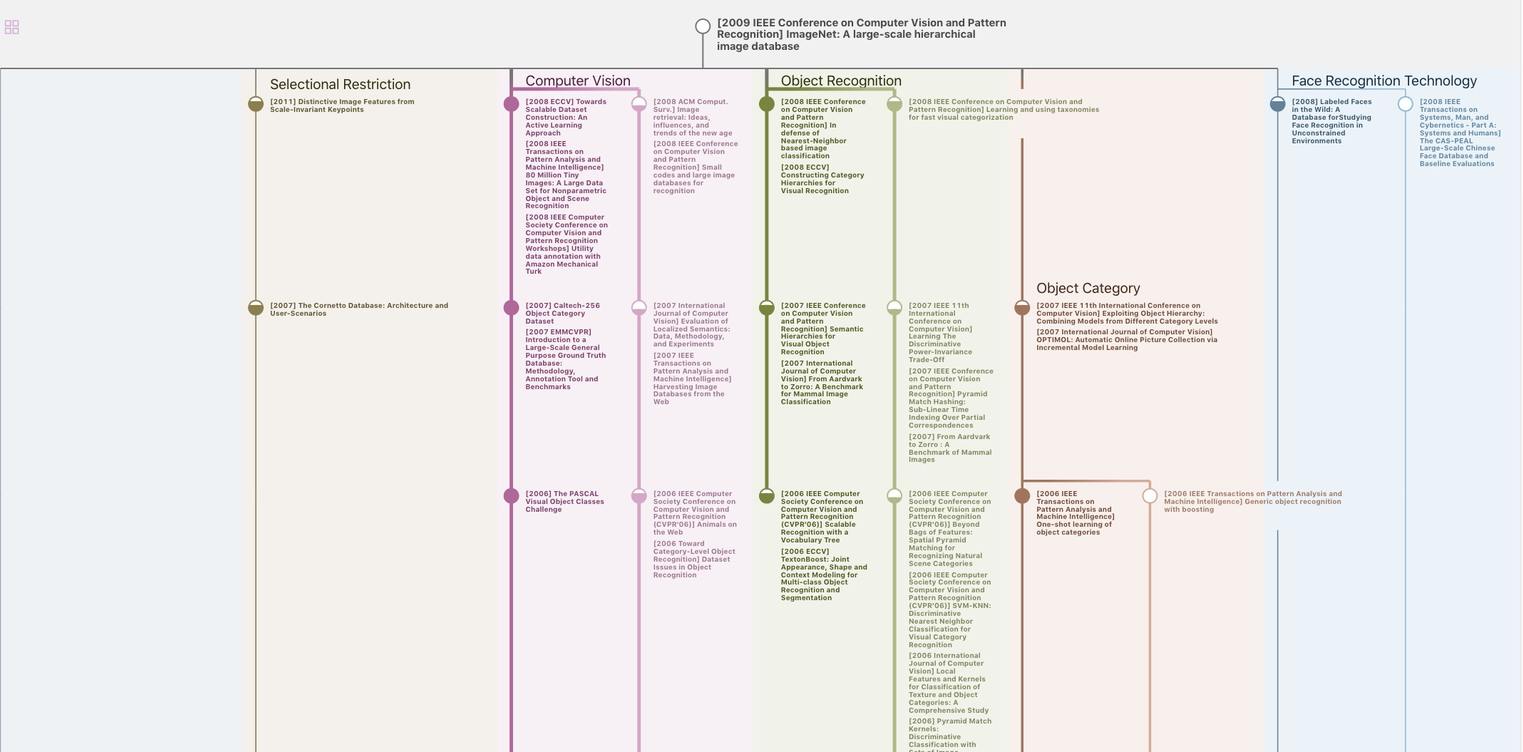
生成溯源树,研究论文发展脉络
Chat Paper
正在生成论文摘要