Evolved explainable classifications for lymph node metastases
Neural Networks(2022)
摘要
A novel evolutionary approach for Explainable Artificial Intelligence is presented: the “Evolved Explanations” model (EvEx). This methodology combines Local Interpretable Model Agnostic Explanations (LIME) with Multi-Objective Genetic Algorithms to allow for automated segmentation parameter tuning in image classification tasks. In this case, the dataset studied is Patch-Camelyon, comprised of patches from pathology whole slide images. A publicly available Convolutional Neural Network (CNN) was trained on this dataset to provide a binary classification for presence/absence of lymph node metastatic tissue. In turn, the classifications are explained by means of evolving segmentations, seeking to optimize three evaluation goals simultaneously. The final explanation is computed as the mean of all explanations generated by Pareto front individuals, evolved by the developed genetic algorithm. To enhance reproducibility and traceability of the explanations, each of them was generated from several different seeds, randomly chosen. The observed results show remarkable agreement between different seeds. Despite the stochastic nature of LIME explanations, regions of high explanation weights proved to have good agreement in the heat maps, as computed by pixel-wise relative standard deviations. The found heat maps coincide with expert medical segmentations, which demonstrates that this methodology can find high quality explanations (according to the evaluation metrics), with the novel advantage of automated parameter fine tuning. These results give additional insight into the inner workings of neural network black box decision making for medical data.
更多查看译文
关键词
Artificial intelligence,Convolutional Neural Networks,Explainable AI,Multi-objective genetic algorithms
AI 理解论文
溯源树
样例
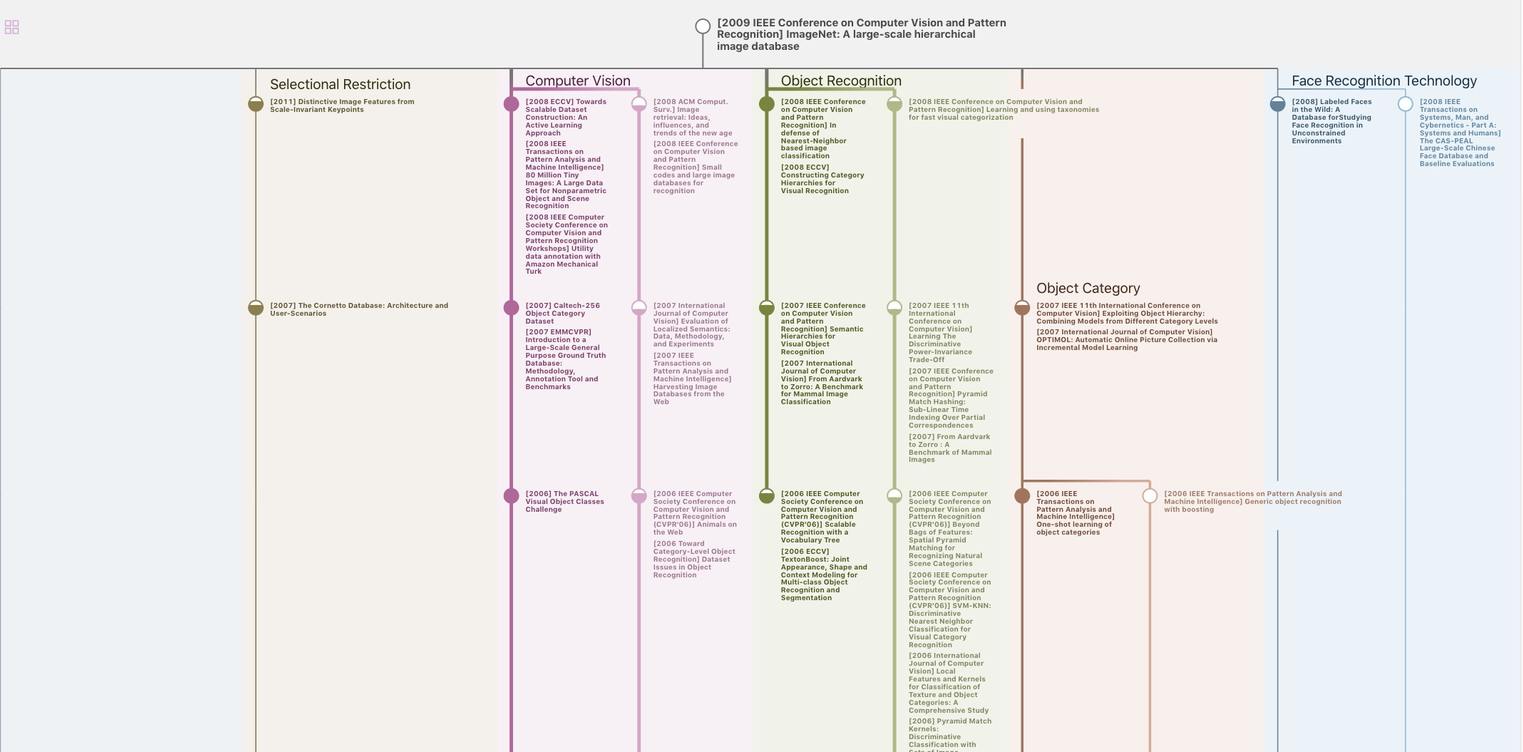
生成溯源树,研究论文发展脉络
Chat Paper
正在生成论文摘要