Squarm-Sgd: Communication-Efficient Momentum Sgd For Decentralized Optimization
2021 IEEE INTERNATIONAL SYMPOSIUM ON INFORMATION THEORY (ISIT)(2021)
摘要
In this paper, we propose and analyze SQuARM-SGD, a communication-efficient algorithm for decentralized training of large-scale machine learning models over a network. In SQuARM-SGD, each node performs a fixed number of local SGD steps using Nesterov's momentum and then sends sparsified and quantized updates to its neighbors regulated by a locally computable triggering criterion. We provide convergence guarantees of our algorithm for general smooth objectives, which, to the best of our knowledge, is the first theoretical analysis for compressed decentralized SGD with momentum updates. We show that SQuARM-SGD converges at rate O(1/root nT), matching that of vanilla distributed SGD. We empirically show that SQuARM-SGD saves significantly in total communicated bits over state-of-the-art without sacrificing much on accuracy.
更多查看译文
关键词
Convergence,Training,Optimization,Stochastic processes,Quantization (signal),Information theory,Wireless communication
AI 理解论文
溯源树
样例
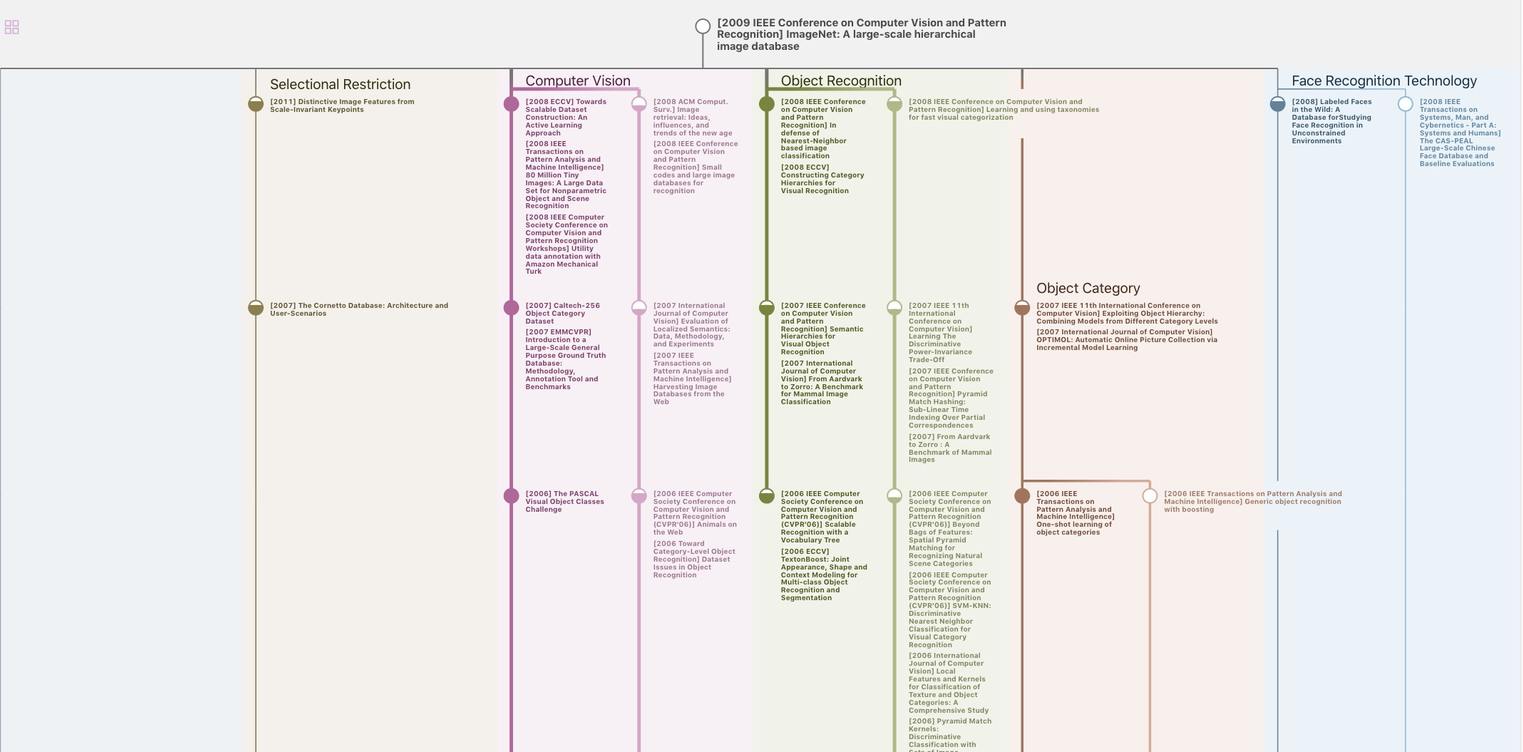
生成溯源树,研究论文发展脉络
Chat Paper
正在生成论文摘要