Improved Algorithms for Learning Equilibria in Simulation-Based Games
AAMAS '19: International Conference on Autonomous Agents and Multiagent Systems Auckland New Zealand May, 2020(2020)
摘要
We tackle a fundamental problem in empirical game-theoretic analysis (EGTA), that of learning equilibria of simulation-based games. Such games cannot be described in analytical form; instead, a black-box simulator can be queried to obtain noisy samples of utilities. Our first theorem establishes that uniform approximations of simulation-based games are equilibrium preserving. We then design algorithms that uniformly approximate simulation-based games with finite-sample guarantees. Our first algorithm, global sampling (GS), extends previous work that constructs confidence intervals assuming bounded utilities with confidence intervals that are sensitive to variance. The second, progressive sample with pruning (PSP), samples progressively, ceasing the sampling process (i.e., pruning strategies) as soon as it determines that the corresponding utilities have been sufficiently well estimated for equilibrium computation. We experiment with our algorithms using both GAMUT, a state-of-the-art game generator, and Gambit, a state-of-the-art game solver. For a broad swath of games, we show that GS using our variance-sensitive bounds outperforms previous work, and that PSP can significantly outperform GS. Here "outperform" means achieving the same guarantees with far fewer samples.
更多查看译文
AI 理解论文
溯源树
样例
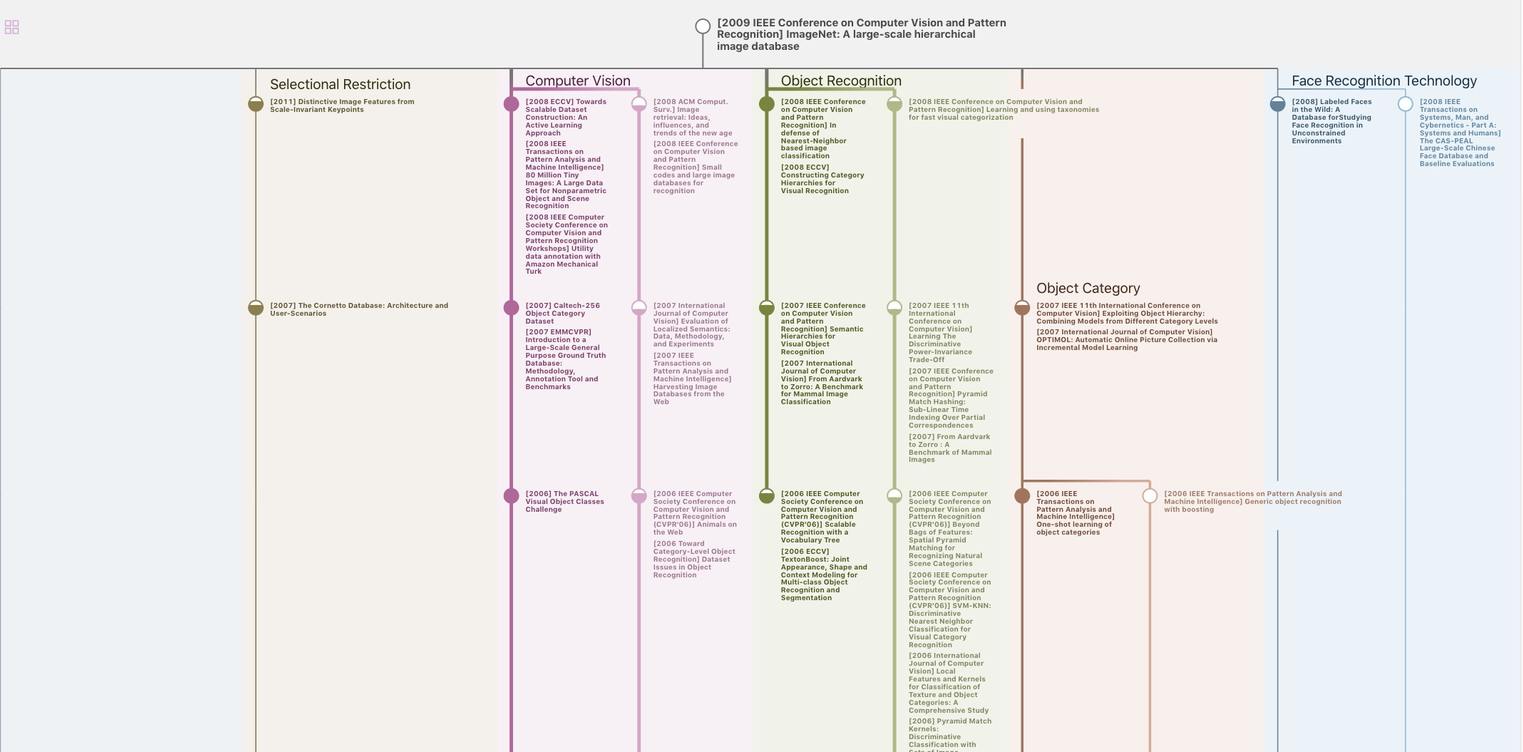
生成溯源树,研究论文发展脉络
Chat Paper
正在生成论文摘要