Zero-Shot Transfer Learning with Synthesized Data for Multi-Domain Dialogue State Tracking
58TH ANNUAL MEETING OF THE ASSOCIATION FOR COMPUTATIONAL LINGUISTICS (ACL 2020)(2020)
摘要
Zero-shot transfer learning for multi-domain dialogue state tracking can allow us to handle new domains without incurring the high cost of data acquisition. This paper proposes new zero-short transfer learning technique for dialogue state tracking where the in-domain training data are all synthesized from an abstract dialogue model and the ontology of the domain. We show that data augmentation through synthesized data can improve the accuracy of zero-shot learning for both the TRADE model and the BERT-based SUMBT model on the MultiWOZ 2.1 dataset. We show training with only synthesized in-domain data on the SUMBT model can reach about 2/3 of the accuracy obtained with the full training dataset. We improve the zero-shot learning state of the art on average across domains by 21%.
更多查看译文
关键词
learning,zero-shot,multi-domain
AI 理解论文
溯源树
样例
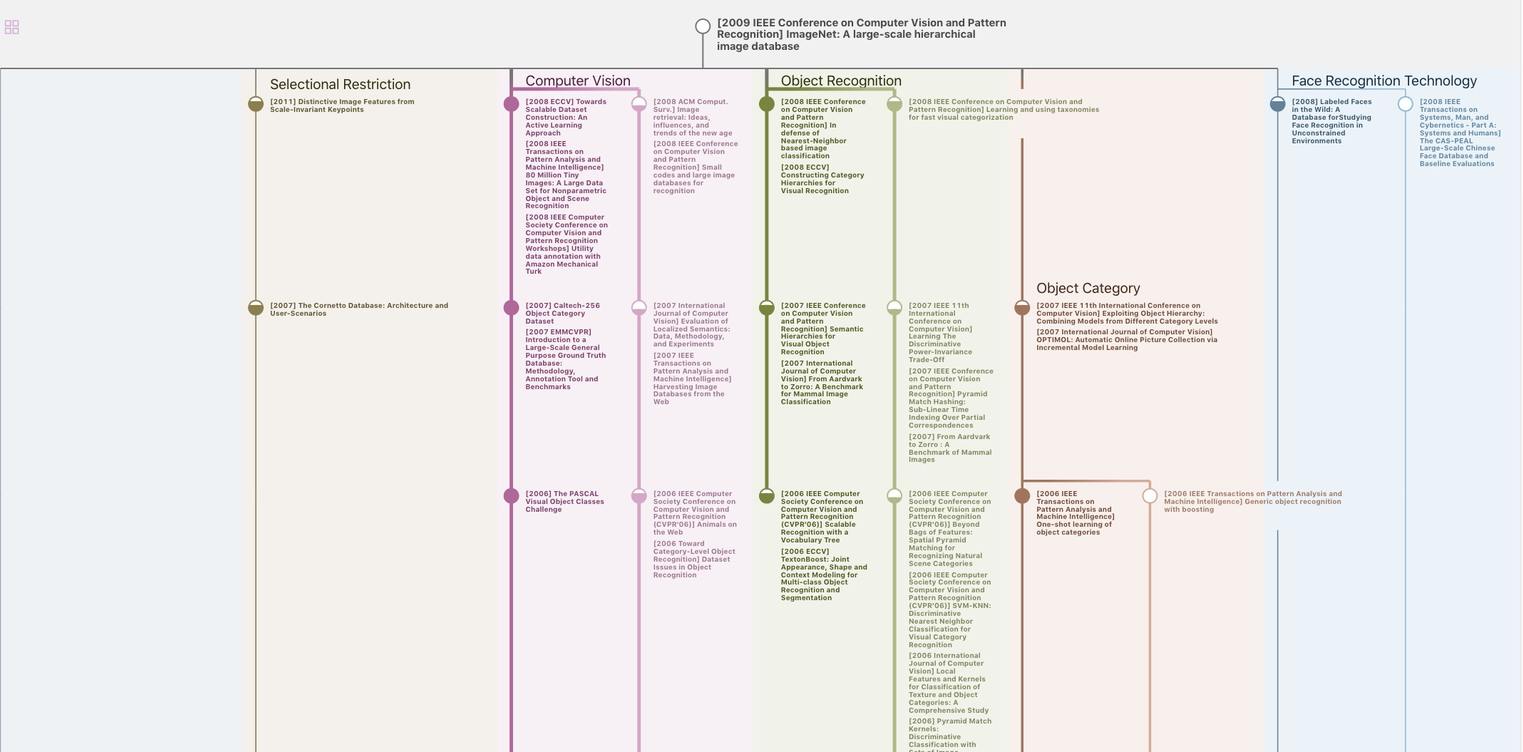
生成溯源树,研究论文发展脉络
Chat Paper
正在生成论文摘要