A Comparative Evaluation of AutoEncoder-Based Unsupervised Anomaly Detection Methods Applied on Space Payload
2019 6th International Conference on Dependable Systems and Their Applications (DSA)(2020)
摘要
The anomaly detection technology is the basis for ensuring the safe and stable operation of the on-rail payload. The traditional threshold-based anomaly detection method has low accuracy and poor flexibility, and cannot detect abnormalities in real time. In addition, due to the lack of abnormal samples, the distribution of positive and negative samples is extremely imbalanced, which increases the difficulty of abnormal detection. Therefore, this paper proposes an unsupervised learning method based on AutoEncoder and its variants, the Basic AutoEncoder, Deep AutoEncoder and Sparse AutoEncoder are used to verify the algorithm on three public datasets. And using the above three algorithms to carry out the case application on the real load dataset. The experiments show whether in the public dataset or the real data of the payload, the three methods of AutoEncoder have achieved good results, proving the AutoEncoder and its variants have a good application in anomaly detection. At the same time, it is verified that the three algorithms have different effects on different datasets, which proves that the AutoEncoder with different characteristics need to be selected in different scenarios.
更多查看译文
关键词
space payload,real-time anomaly detection,AutoEncoder
AI 理解论文
溯源树
样例
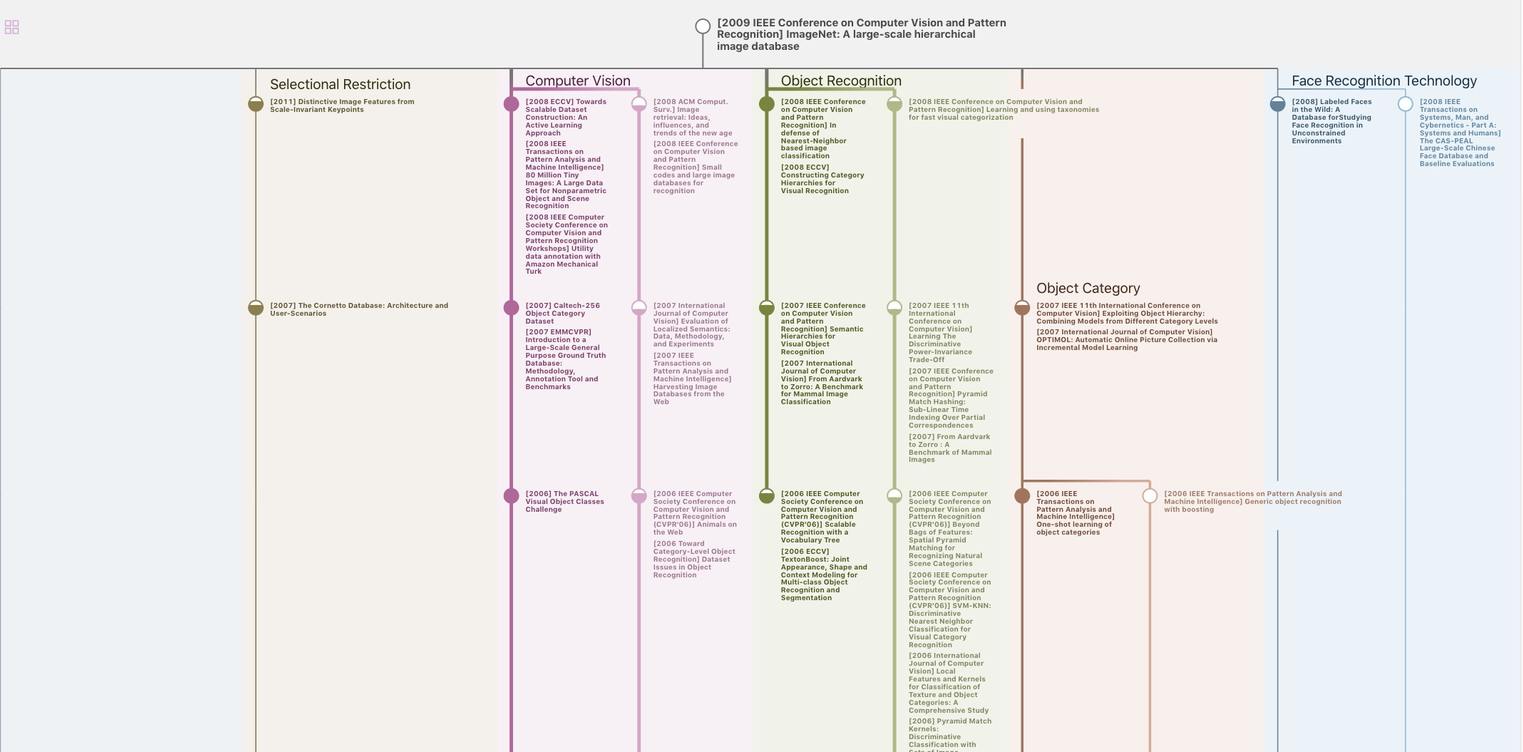
生成溯源树,研究论文发展脉络
Chat Paper
正在生成论文摘要