Diffusion copulas: Identification and estimation
Journal of Econometrics(2021)
摘要
We propose a new semiparametric approach for modelling nonlinear univariate diffusions, where the observed process is a nonparametric transformation of an underlying parametric diffusion (UPD). This modelling strategy yields a general class of semiparametric Markov diffusion models with parametric dynamic copulas and nonparametric marginal distributions. We provide primitive conditions for the identification of the UPD parameters together with the unknown transformations from discrete samples. Likelihood-based estimators of both parametric and nonparametric components are developed and we analyse their asymptotic properties. Kernel-based drift and diffusion estimators are also proposed and shown to be normally distributed in large samples. A simulation study investigates the finite sample performance of our estimators in the context of modelling US short-term interest rates. We also present a simple application of the proposed method for modelling the CBOE volatility index data.
更多查看译文
关键词
C14,C22,C32,C58,G12
AI 理解论文
溯源树
样例
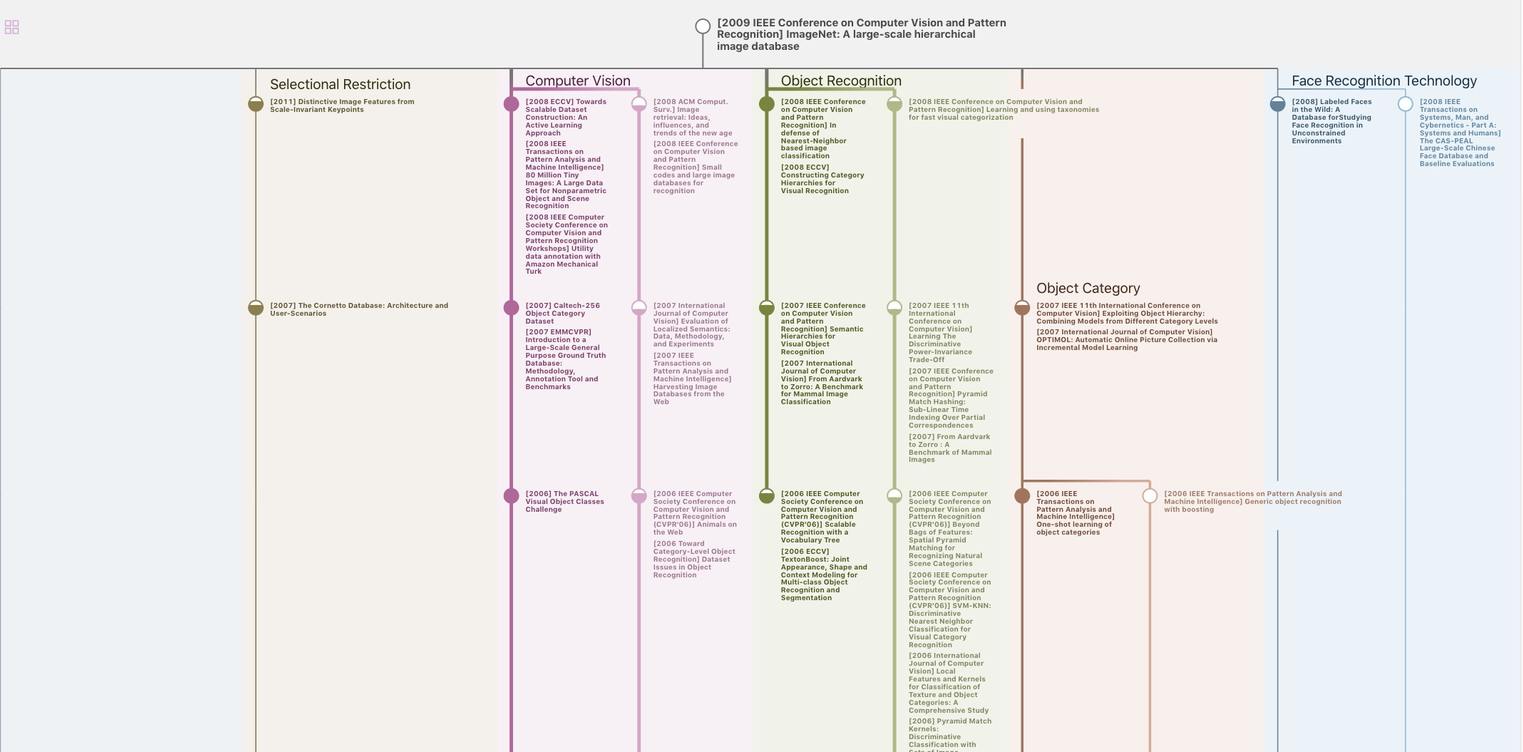
生成溯源树,研究论文发展脉络
Chat Paper
正在生成论文摘要