A 1.15-TOPS 6.57-TOPS/W DNN Processor for Multi-Scale Object Detection
2020 2nd IEEE International Conference on Artificial Intelligence Circuits and Systems (AICAS)(2020)
摘要
We present a 40-nm multi-scale object detection processor with only three operations: 3 × 3 convolution, 1 × 1 convolution, and 4 × 4 deconvolution. The multi-scale object detection at high accuracy is possible by virtue of the deconvolution feature. Input memory for a feature map has 8-bit width as well as a multiplier for the inputs has 8-bit precision. Partial-sum memory, however, has 16-bit width to suppress detection accuracy deterioration in a layer with 5 12 channels or more. By fixed-point bit precision, the external memory bandwidth and internal memory capacity are reduced. Optimized parallelization in input and output channels reduces the external memory bandwidth to 0.50 GB per 1280 × 384 image with internal memory capacity of 400 kB. The detection error is 1.9% of that using single-precision floating point. The maximum operating frequency is 500 MHz at a supply voltage of 1 V. Its peak performance is 1. 15 TOPS. The maximum energy efficiency is 6.57 TOPS/W at 174 MHz and 0.6 V.
更多查看译文
关键词
Self-driving cars,Convolutional neural network,Deconvolution,Multi-scale object detection
AI 理解论文
溯源树
样例
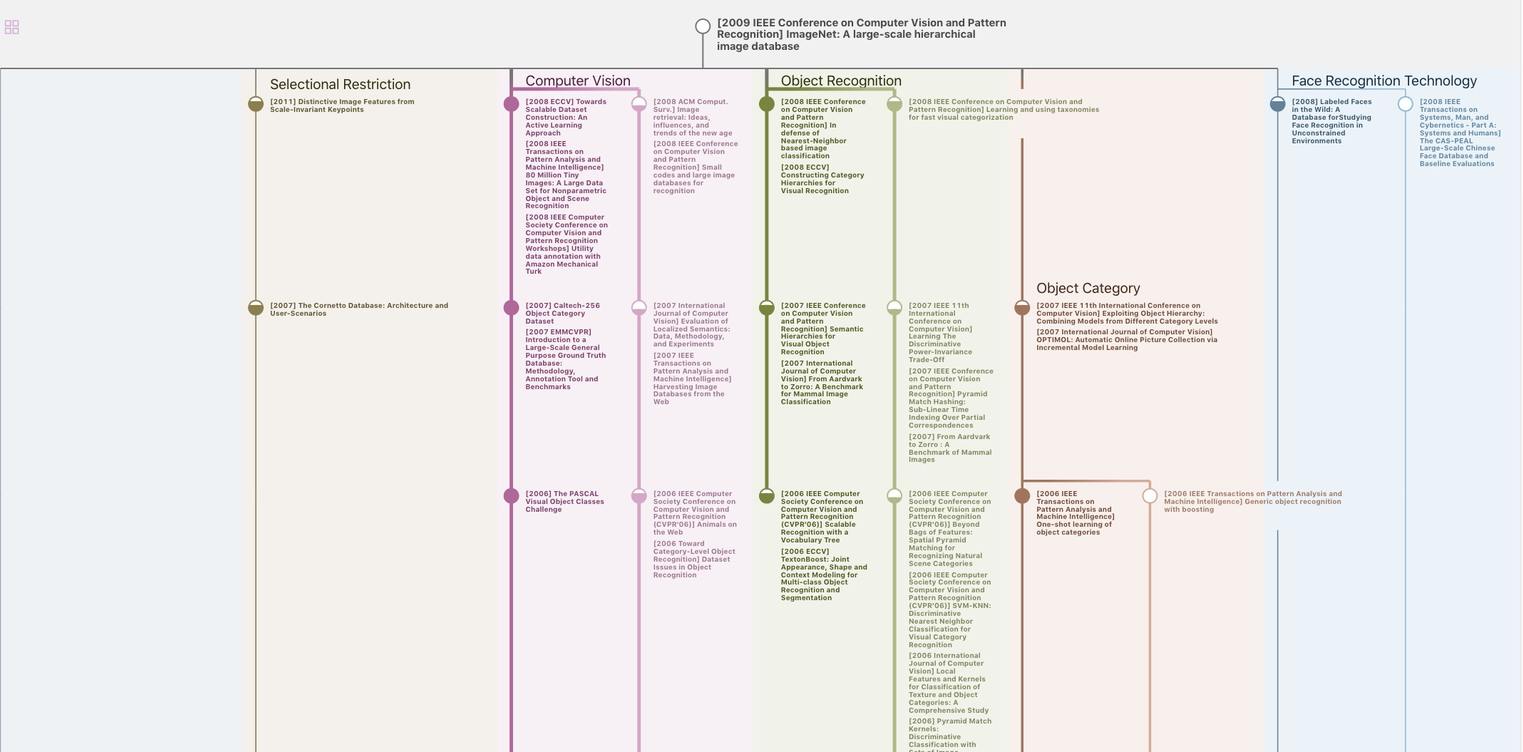
生成溯源树,研究论文发展脉络
Chat Paper
正在生成论文摘要