Query-By-Committee Framework Used for Semi-Automatic Sleep Stages Classification.
UCAmI(2019)
摘要
Active learning is very useful for classification problems where it is hard or time-consuming to acquire classes of data in order to create a subset for training a classifier. The classification of over-night polysomnography records to sleep stages is an example of such application because an expert has to annotate a large number of segments of a record. Active learning methods enable us to iteratively select only the most informative instances for the manual classification so the total expert’s effort is reduced. However, the process is able to be insufficiently initialised because of a large dimensionality of polysomnography (PSG) data, so the fast convergence of active learning is at risk. In order to prevent this threat, we have proposed a variant of the query-by-committee active learning scenario which take into account all features of data so it is not necessary to reduce a feature space, but the process is quickly initialised. The proposed method is compared to random sampling and margin uncertainty sampling which is another well-known active learning method. It was shown that, during crucial first iteration of the process, the provided variant of query-by-committee acquired the best results among other strategies in most cases.
更多查看译文
关键词
active learning,uncertainty sampling,query-by-committee,psg classification,sleep stages classification
AI 理解论文
溯源树
样例
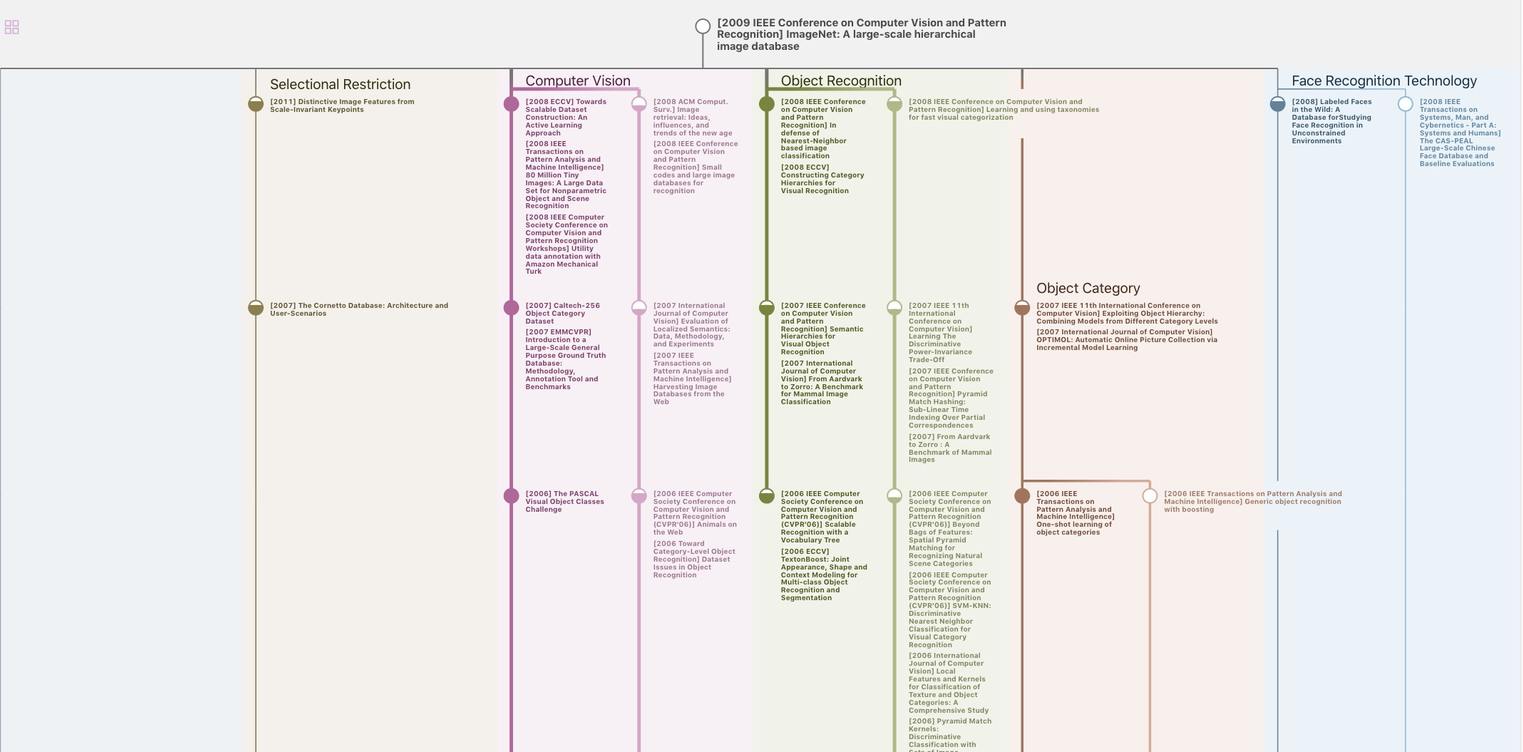
生成溯源树,研究论文发展脉络
Chat Paper
正在生成论文摘要