KGClean: An Embedding Powered Knowledge Graph Cleaning Framework
arxiv(2020)
摘要
The quality assurance of the knowledge graph is a prerequisite for various knowledge-driven applications. We propose KGClean, a novel cleaning framework powered by knowledge graph embedding, to detect and repair the heterogeneous dirty data. In contrast to previous approaches that either focus on filling missing data or clean errors violated limited rules, KGClean enables (i) cleaning both missing data and other erroneous values, and (ii) mining potential rules automatically, which expands the coverage of error detecting. KGClean first learns data representations by TransGAT, an effective knowledge graph embedding model, which gathers the neighborhood information of each data and incorporates the interactions among data for casting data to continuous vector spaces with rich semantics. KGClean integrates an active learning-based classification model, which identifies errors with a small seed of labels. KGClean utilizes an efficient PRO-repair strategy to repair errors using a novel concept of propagation power. Extensive experiments on four typical knowledge graphs demonstrate the effectiveness of KGClean in practice.
更多查看译文
关键词
cleaning,knowledge
AI 理解论文
溯源树
样例
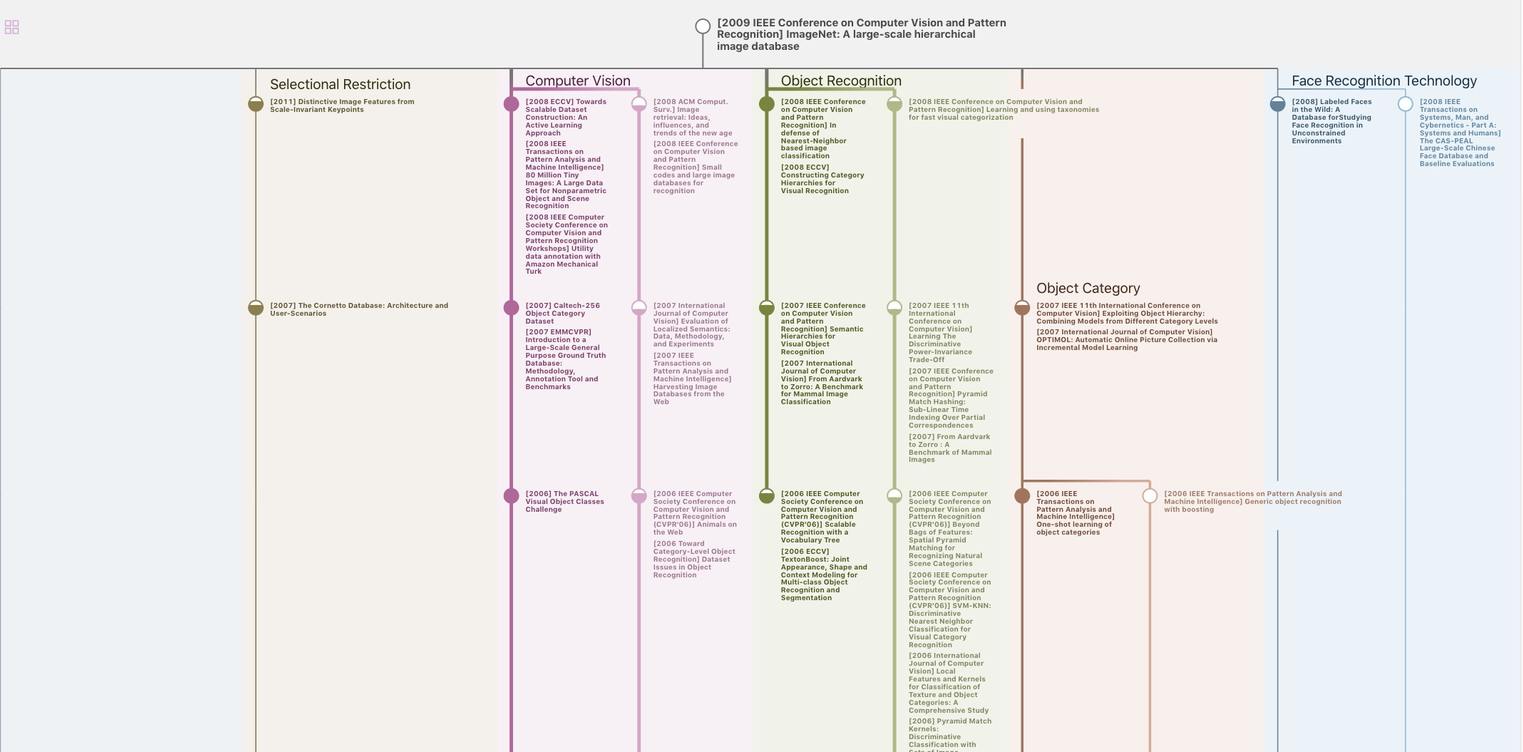
生成溯源树,研究论文发展脉络
Chat Paper
正在生成论文摘要