One Sparse Perturbation to Fool them All, almost Always!
arxiv(2020)
摘要
Constructing adversarial perturbations for deep neural networks is an important direction of research. Crafting image-dependent adversarial perturbations using white-box feedback has hitherto been the norm for such adversarial attacks. However, black-box attacks are much more practical for real-world applications. Universal perturbations applicable across multiple images are gaining popularity due to their innate generalizability. There have also been efforts to restrict the perturbations to a few pixels in the image. This helps to retain visual similarity with the original images making such attacks hard to detect. This paper marks an important step which combines all these directions of research. We propose the DEceit algorithm for constructing effective universal pixel-restricted perturbations using only black-box feedback from the target network. We conduct empirical investigations using the ImageNet validation set on the state-of-the-art deep neural classifiers by varying the number of pixels to be perturbed from a meagre 10 pixels to as high as 1 ar X iv :2 00 4. 13 00 2v 1 [ cs .C R ] 2 4 A pr 2 02 0 10 00 50 00 10 00 0 50 17 6 Number of perturbed pixels 50 60 70 80 Fo ol in g R at e (% ) DEceit on GoogleNet DEceit on VGG16 UAP on VGG16 UAP on GoogleNet (a) 10 00 50 00 10 00 0 50 17 6 Number of perturbed pixels 30 40 50 60 PS N R (d B ) DEceit on GoogleNet DEceit on VGG16 UAP on VGG16 UAP on GoogleNet
更多查看译文
关键词
Adversarial attack,Black-box attack,Convolutional image classifier,Differential evolution,Sparse universal attack
AI 理解论文
溯源树
样例
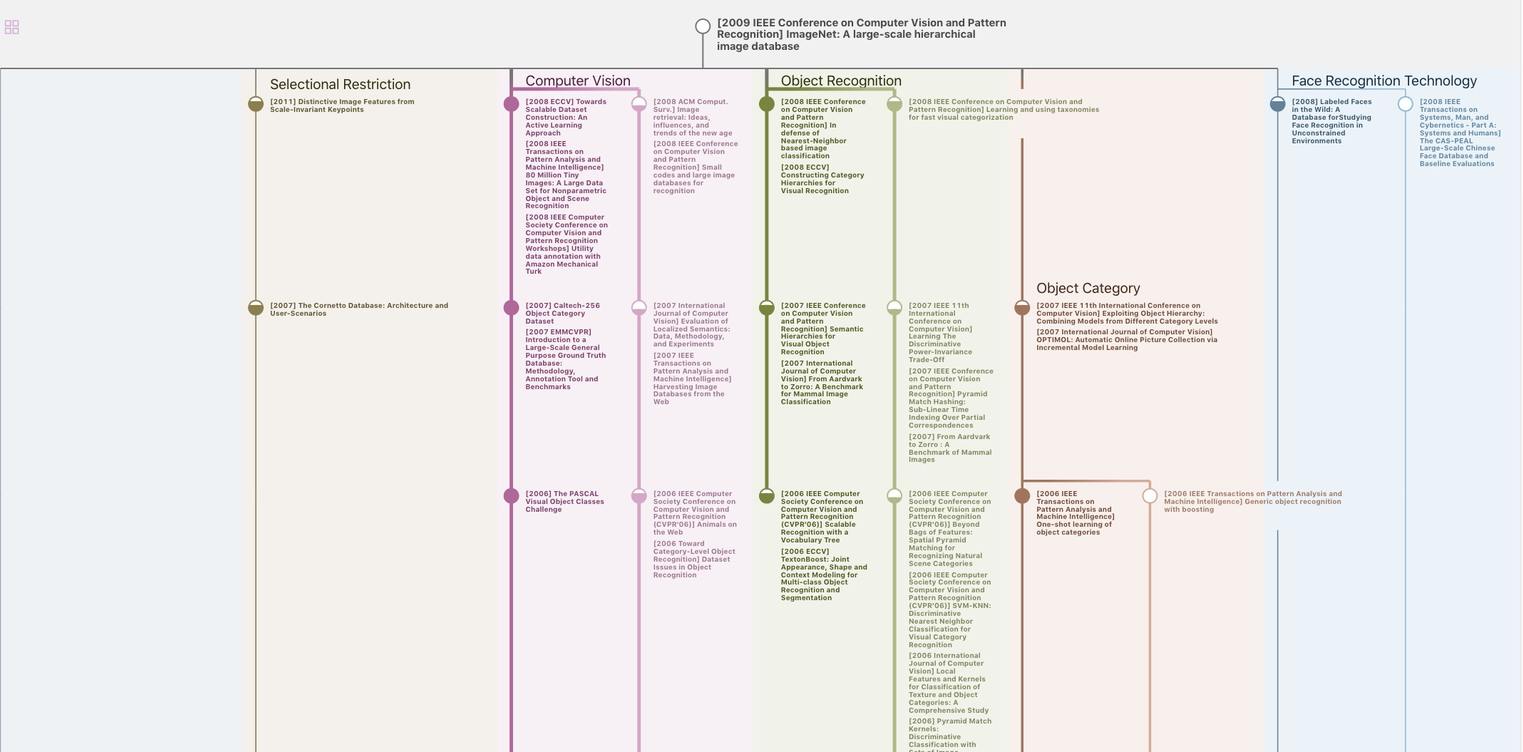
生成溯源树,研究论文发展脉络
Chat Paper
正在生成论文摘要