Difficulty Translation in Histopathology Images
AIME(2020)
摘要
The unique nature of histopathology images opens the door to domain-specific formulations of image translation models. We propose a difficulty translation model that modifies colorectal histopathology images to become more challenging to classify. Our model comprises a scorer, which provides an output confidence to measure the difficulty of images, and an image translator, which learns to translate images from easy-to-classify to hard-to-classify using a training set defined by the scorer. We present three findings. First, generated images were indeed harder to classify for both human pathologists and machine learning classifiers than their corresponding source images. Second, image classifiers trained with generated images as augmented data performed better on both easy and hard images from an independent test set. Finally, human annotator agreement and our model's measure of difficulty correlated strongly, implying that for future work requiring human annotator agreement, the confidence score of a machine learning classifier could be used instead as a proxy.
更多查看译文
关键词
Deep learning, Histopathology images, Generative adversarial networks
AI 理解论文
溯源树
样例
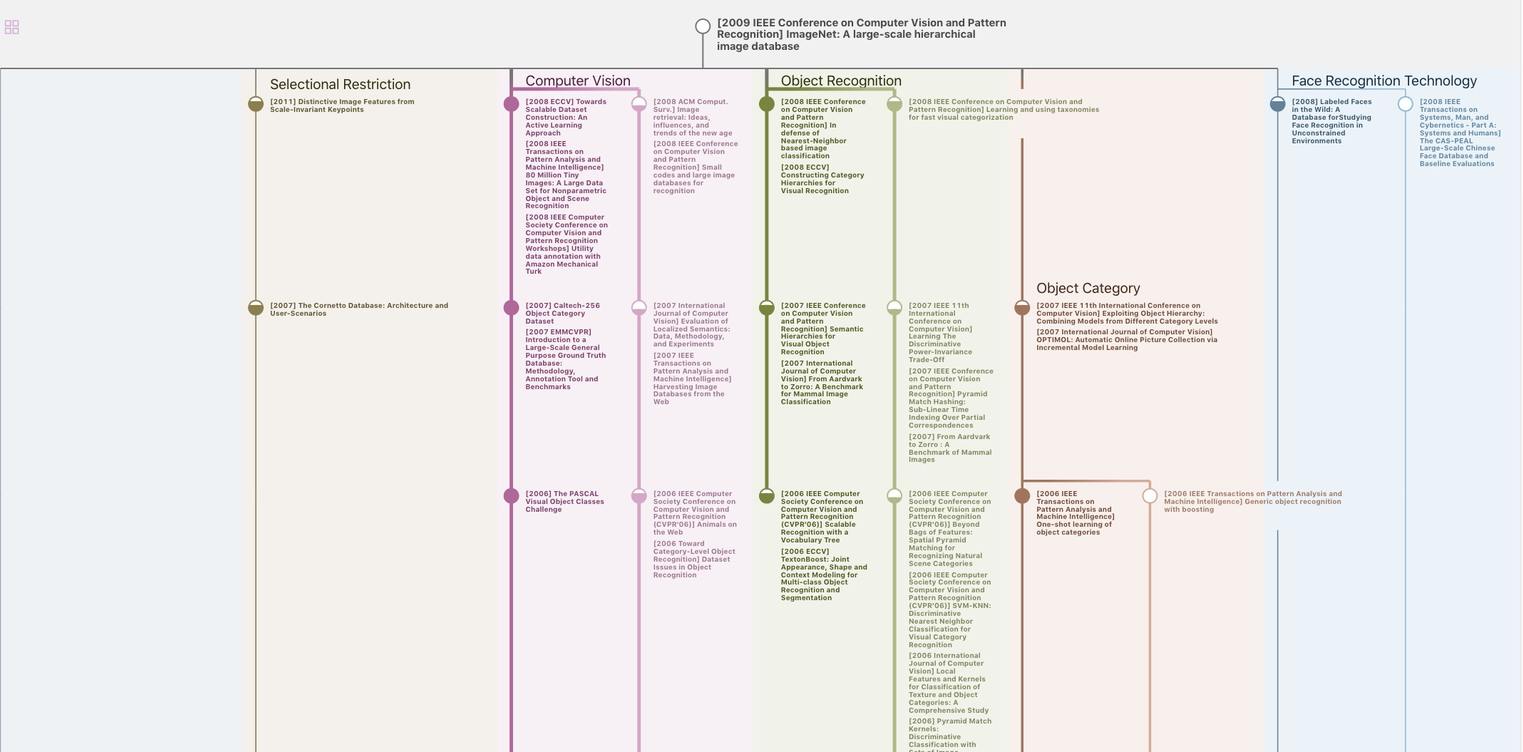
生成溯源树,研究论文发展脉络
Chat Paper
正在生成论文摘要