FDHelper: Assist Unsupervised Fraud Detection Experts with Interactive Feature Selection and Evaluation
CHI '20: CHI Conference on Human Factors in Computing Systems Honolulu HI USA April, 2020(2020)
摘要
Online fraud is the well-known dark side of the modern Internet. Unsupervised fraud detection algorithms are widely used to address this problem. However, selecting features, adjusting hyperparameters, evaluating the algorithms, and eliminating false positives all require human expert involvement. In this work, we design and implement an end-to-end interactive visualization system, FDHelper, based on the deep understanding of the mechanism of the black market and fraud detection algorithms. We identify a workflow based on experience from both fraud detection algorithm experts and domain experts. Using a multi-granularity three-layer visualization map embedding an entropy-based distance metric ColDis, analysts can interactively select different feature sets, refine fraud detection algorithms, tune parameters and evaluate the detection result in near real-time. We demonstrate the effectiveness and significance of FDHelper through two case studies with state-of-the-art fraud detection algorithms, interviews with domain experts and algorithm experts, and a user study with eight first-time end users.
更多查看译文
关键词
Human Computer Interaction, Fraud Detection, Visualization
AI 理解论文
溯源树
样例
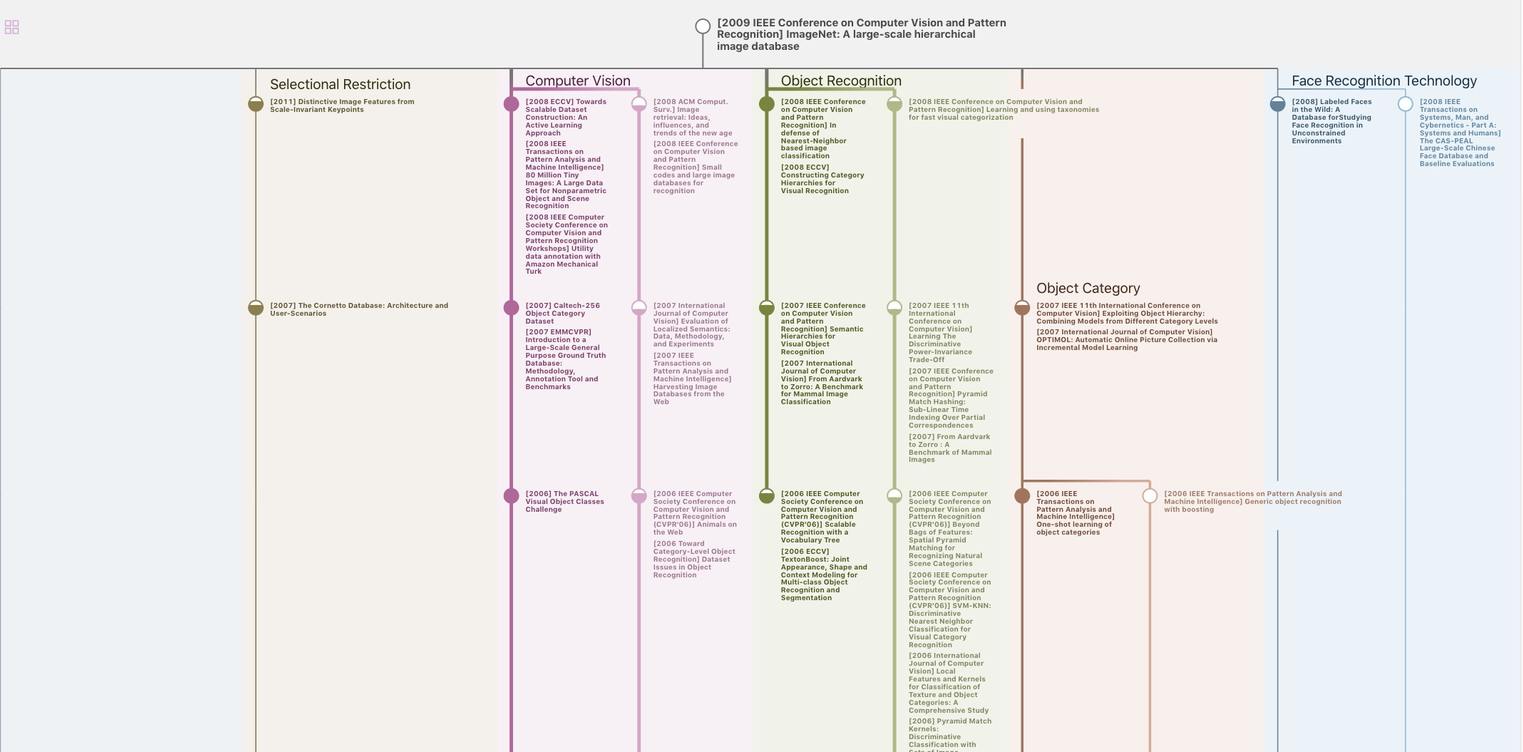
生成溯源树,研究论文发展脉络
Chat Paper
正在生成论文摘要