Evaluation of a Deep Neural Network for Automated Classification of Colorectal Polyps on Histopathologic Slides.
JAMA NETWORK OPEN(2020)
摘要
Question Are deep neural networks trained on data from a single institution for classification of colorectal polyps on digitized histopathologic slides generalizable across multiple external institutions? Findings In this prognostic study of a deep neural network to classify the 4 most common polyp types on digitized histopathologic slides from a single institution (internal test set) and 24 US institutions (external test set), the mean accuracy was 93.5% on the internal test set and 87.0% on the external test set. Meaning Deep neural networks may provide a generalizable approach for the classification of colorectal polyps on digitized histopathologic slides. This prognostic study evaluates the performance and generalizability of a deep neural network trained on data from a single institution for classification of colorectal polyps on histopathologic slide images. Importance Histologic classification of colorectal polyps plays a critical role in screening for colorectal cancer and care of affected patients. An accurate and automated algorithm for the classification of colorectal polyps on digitized histopathologic slides could benefit practitioners and patients. Objective To evaluate the performance and generalizability of a deep neural network for colorectal polyp classification on histopathologic slide images using a multi-institutional data set. Design, Setting, and Participants This prognostic study used histopathologic slides collected from January 1, 2016, to June 31, 2016, from Dartmouth-Hitchcock Medical Center, Lebanon, New Hampshire, with 326 slides used for training, 157 slides for an internal data set, and 25 for a validation set. For the external data set, 238 slides for 179 distinct patients were obtained from 24 institutions across 13 US states. Data analysis was performed from April 9 to November 23, 2019. Main Outcomes and Measures Accuracy, sensitivity, and specificity of the model to classify 4 major colorectal polyp types: tubular adenoma, tubulovillous or villous adenoma, hyperplastic polyp, and sessile serrated adenoma. Performance was compared with that of local pathologists' at the point of care identified from corresponding pathology laboratories. Results For the internal evaluation on the 157 slides with ground truth labels from 5 pathologists, the deep neural network had a mean accuracy of 93.5% (95% CI, 89.6%-97.4%) compared with local pathologists' accuracy of 91.4% (95% CI, 87.0%-95.8%). On the external test set of 238 slides with ground truth labels from 5 pathologists, the deep neural network achieved an accuracy of 87.0% (95% CI, 82.7%-91.3%), which was comparable with local pathologists' accuracy of 86.6% (95% CI, 82.3%-90.9%). Conclusions and Relevance The findings suggest that this model may assist pathologists by improving the diagnostic efficiency, reproducibility, and accuracy of colorectal cancer screenings.
更多查看译文
关键词
colorectal polyps,deep neural network,automated classification
AI 理解论文
溯源树
样例
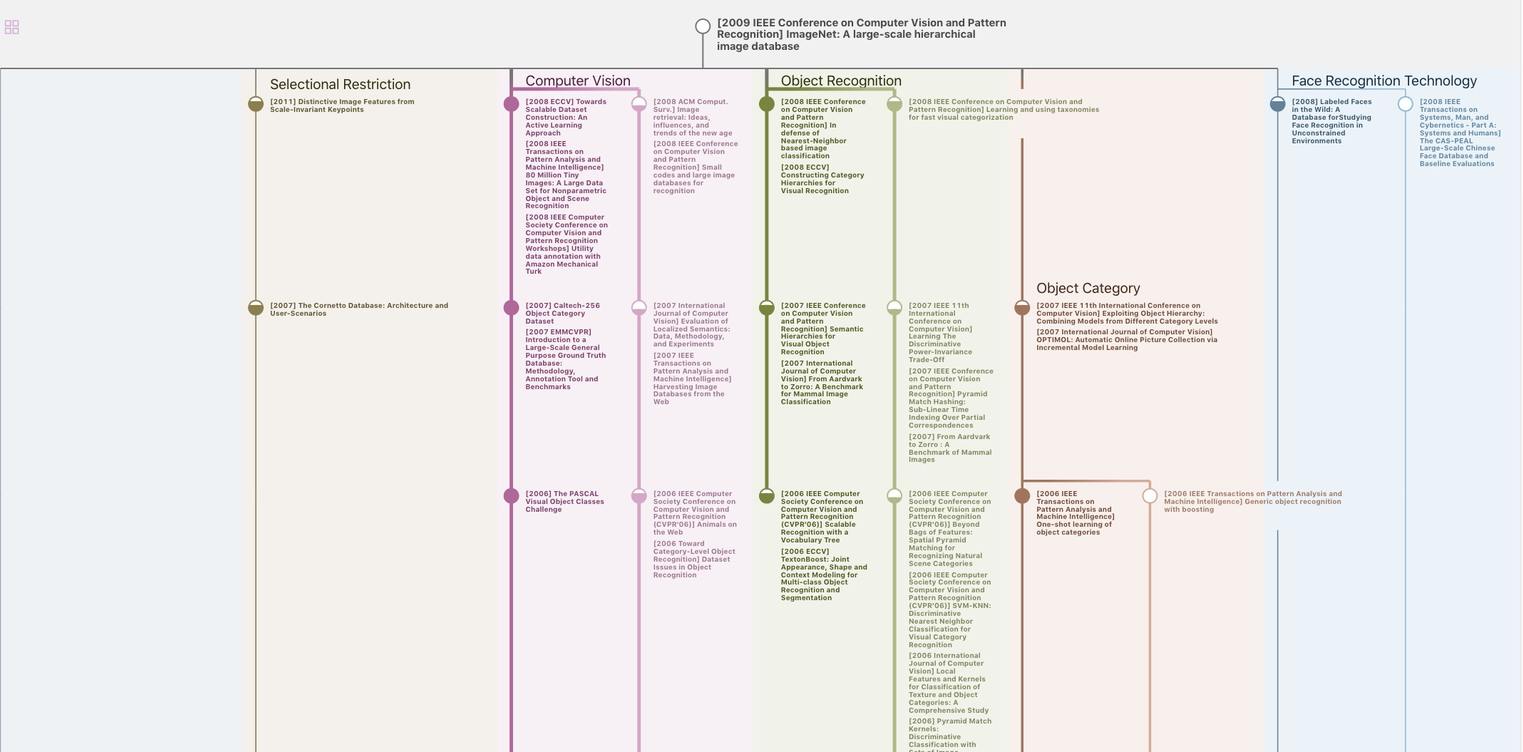
生成溯源树,研究论文发展脉络
Chat Paper
正在生成论文摘要