Learned optical flow for intra-operative tracking of the retinal fundus
International Journal of Computer Assisted Radiology and Surgery(2020)
摘要
Purpose Sustained delivery of regenerative retinal therapies by robotic systems requires intra-operative tracking of the retinal fundus. We propose a supervised deep convolutional neural network to densely predict semantic segmentation and optical flow of the retina as mutually supportive tasks, implicitly inpainting retinal flow information missing due to occlusion by surgical tools. Methods As manual annotation of optical flow is infeasible, we propose a flexible algorithm for generation of large synthetic training datasets on the basis of given intra-operative retinal images. We evaluate optical flow estimation by tracking a grid and sparsely annotated ground truth points on a benchmark of challenging real intra-operative clips obtained from an extensive internally acquired dataset encompassing representative vitreoretinal surgical cases. Results The U-Net-based network trained on the synthetic dataset is shown to generalise well to the benchmark of real surgical videos. When used to track retinal points of interest, our flow estimation outperforms variational baseline methods on clips containing tool motions which occlude the points of interest, as is routinely observed in intra-operatively recorded surgery videos. Conclusions The results indicate that complex synthetic training datasets can be used to specifically guide optical flow estimation. Our proposed algorithm therefore lays the foundation for a robust system which can assist with intra-operative tracking of moving surgical targets even when occluded.
更多查看译文
关键词
Synthetic data, Optical flow, Retinal tracking, Deep learning
AI 理解论文
溯源树
样例
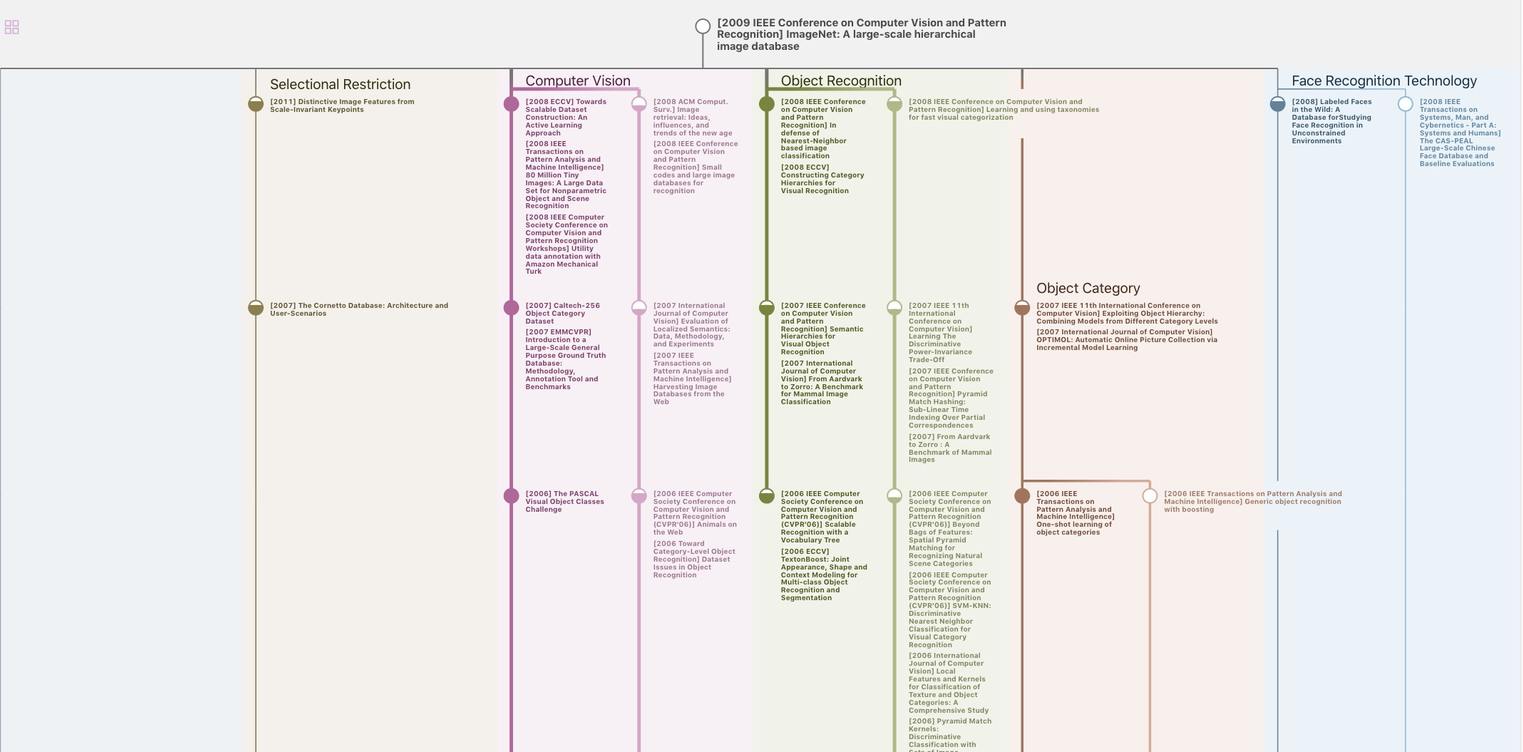
生成溯源树,研究论文发展脉络
Chat Paper
正在生成论文摘要