Dependability‐based cluster weighting in clustering ensemble
Periodicals(2020)
摘要
AbstractAbstractAfter observing the ensemble success in supervised learning (such as classification), it was extended into unsupervised learning. Therefore, cluster ensemble, which merges multiple basic data partitions or clusters (called as ensemble pool) into an ordinarily better clustering solution usually named as consensus partition, emerged. Any cluster ensemble method tries to optimize a particular criterion during extracting the consensus partition out of the ensemble pool. But traditional cluster ensembles consider all the pool members with the equal importance in making the consensus partition; that is to say that each basic partition or cluster participates in the cluster ensemble algorithm equivalently. Indeed, they ignore to consider any ensemble member according to its importance. But it is obvious that some clusters with more quality deserve more emphasis and some clusters with less quality deserve less emphasis during generating consensus partition. This paper proposes (a) a metric to evaluate quality of any arbitrary cluster, (b) a mechanism to project the computed quality of a cluster into a meaningful weight value, and (c) an approach to apply the weight values of the basic clusters in the cluster ensemble process. Experimental results conducted on a number of real‐world standard datasets indicate that the proposed method outperforms the state of the art methods.
更多查看译文
关键词
cluster dependability,clustering ensemble,consensus partition,entropy
AI 理解论文
溯源树
样例
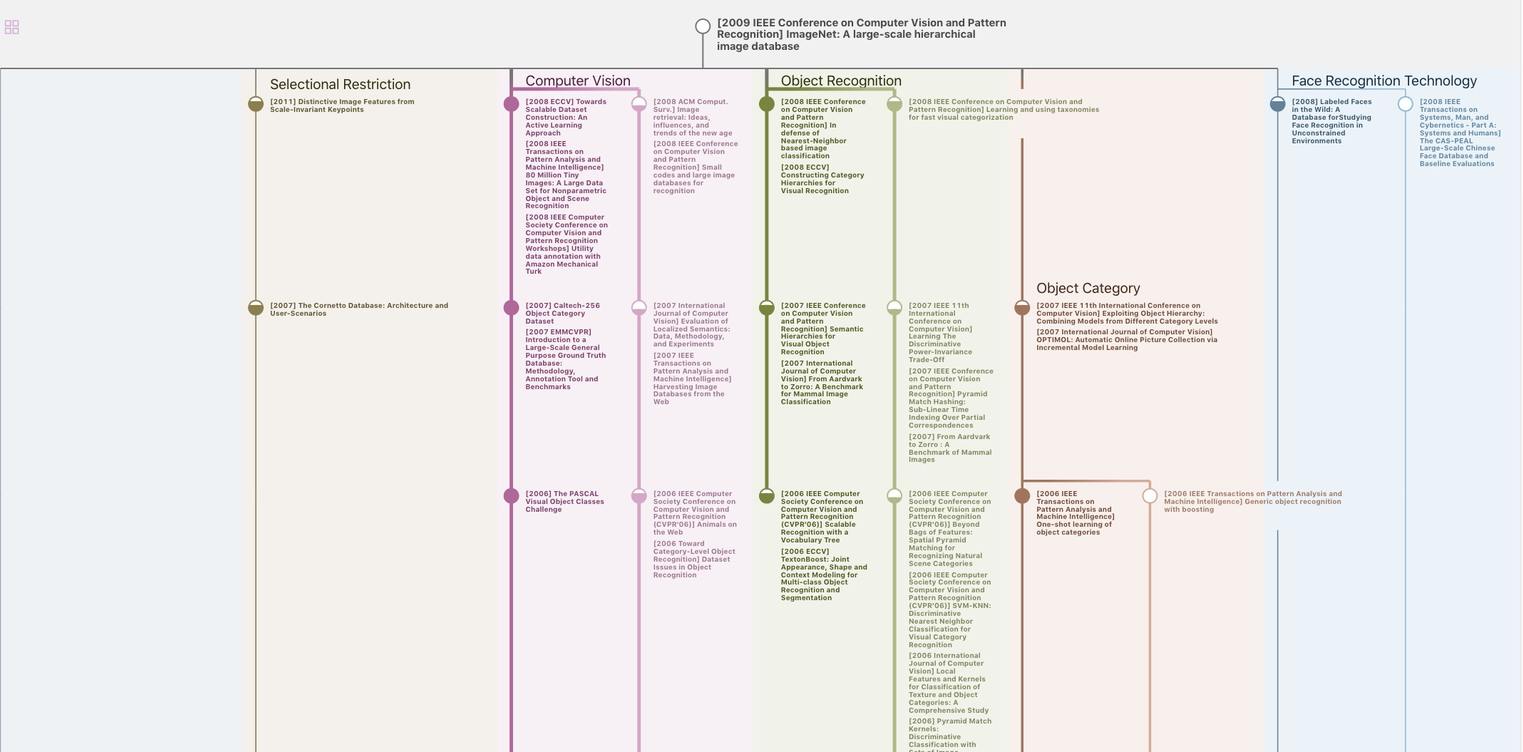
生成溯源树,研究论文发展脉络
Chat Paper
正在生成论文摘要