Forecast of streamflows to the Arctic Ocean by a Bayesian neural network model with snowcover and climate inputs
HYDROLOGY RESEARCH(2020)
摘要
Increasing water flowing into the Arctic Ocean affects oceanic freshwater balance, which may lead to the thermohaline circulation collapse and unpredictable climatic conditions if freshwater inputs continue to increase. Despite the crucial role of ocean inflow in the climate system, less is known about its predictability, variability, and connectivity to cryospheric and climatic patterns on different time scales. In this study, multi-scale variation modes were decomposed from observed daily and monthly snowcover and river flows to improve the predictability of Arctic Ocean inflows from the Mackenzie River Basin in Canada. Two multi-linear regression and Bayesian neural network models were used with different combinations of remotely sensed snowcover, in-situ inflow observations, and climatic teleconnection patterns as predictors. The results showed that daily and monthly ocean inflows are associated positively with decadal snowcover fluctuations and negatively with interannual snowcover fluctuations. Interannual snowcover and antecedent flow oscillations have a more important role in describing the variability of ocean inflows than seasonal snowmelt and large-scale climatic teleconnection. Both models forecasted inflows seven months in advance with a Nash-Sutcliffe efficiency score of approximate to 0.8. The proposed methodology can be used to assess the variability of the freshwater input to northern oceans, affecting thermohaline and atmospheric circulations.
更多查看译文
关键词
Arctic ocean,Bayesian neural network,climate teleconnections,Mackenzie River Basin,snowcover extent,streamflow forecast
AI 理解论文
溯源树
样例
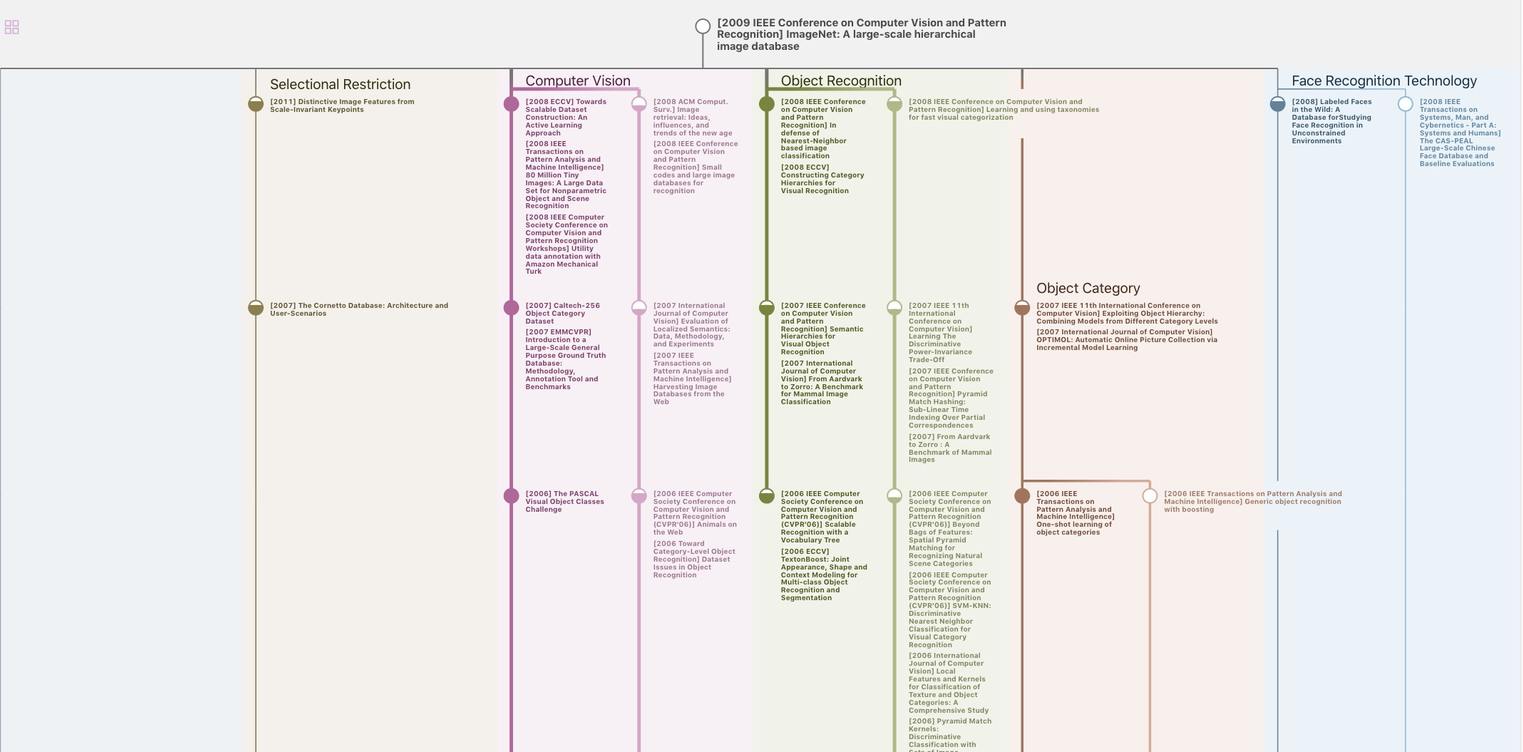
生成溯源树,研究论文发展脉络
Chat Paper
正在生成论文摘要