A Joint Neural Network for Session-aware Recommendation
IEEE ACCESS(2020)
摘要
Session-aware recommendation is a special form of sequential recommendation, where users & x2019; previous interactions before current session are available. Recently, Recurrent Neural Network (RNN) based models are widely used in sequential recommendation tasks with great success. Previous works mainly focus on the interaction sequences of the current session without analyzing a user & x2019;s long-term preferences. In this paper, we propose a joint neural network (JNN) for session-aware recommendation, which employs a Convolutional Neural Network(CNN) and a RNN to process the long-term historical interactions and the short-term sequential interactions respectively. Then, we apply a fully-connected neural network to study the complex relationship between these two types of features, which aims to generate a unified representation of the current session. Finally, a recommendation score for given items is generated by a bi-linear scheme upon the session representation. We conduct our experiments on three public datasets, showing that JNN outperforms the state-of-the-art baselines on all datasets in terms of Recall and Mean Reciprocal Rank (MRR). The outperforming results indicate that proper handling of historical interactions can improve the effectiveness of recommendation. The experimental results show that JNN is more prominent in samples with short current session or long historical interactions.
更多查看译文
关键词
Session-aware recommendation,sequential recommendation,recurrent neural networks,convolutional neural networks
AI 理解论文
溯源树
样例
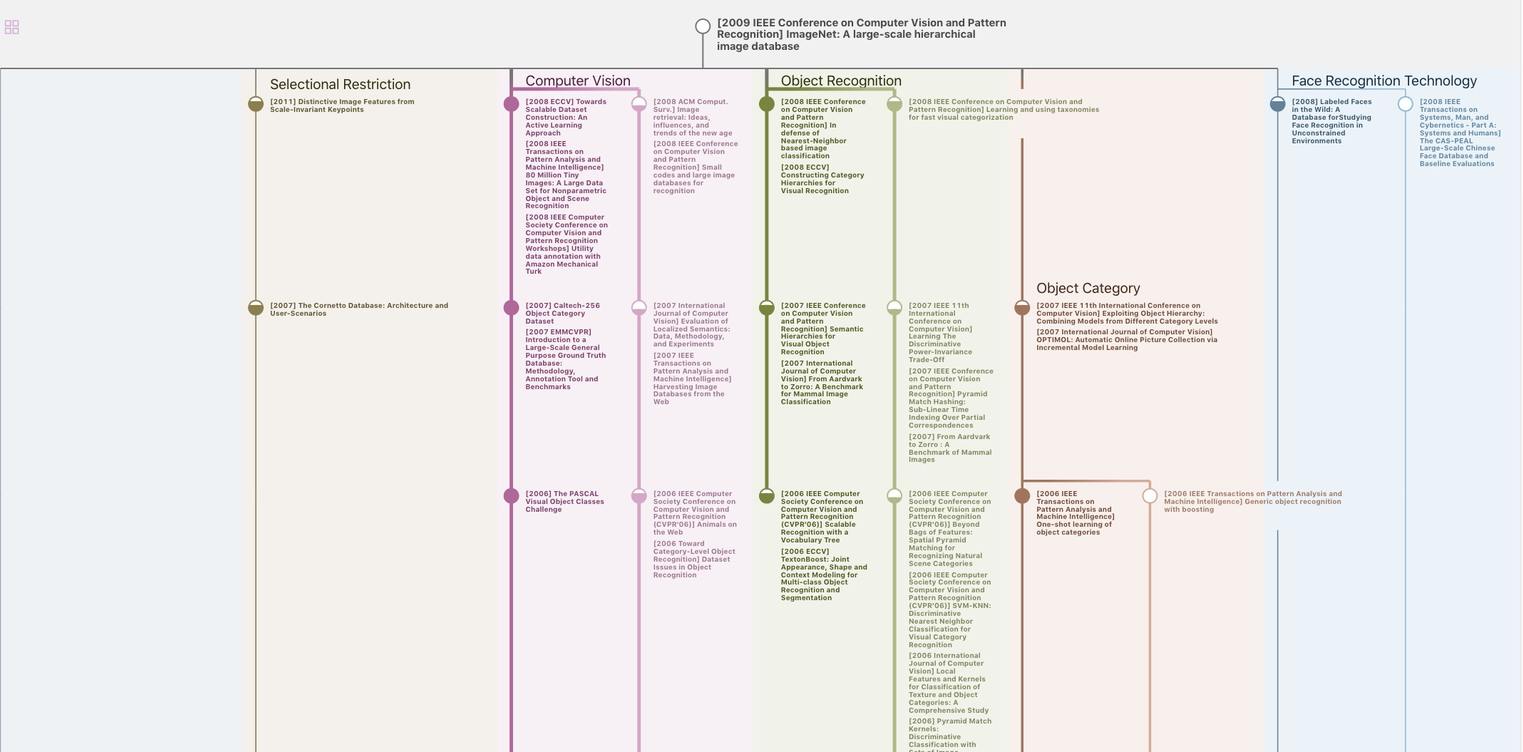
生成溯源树,研究论文发展脉络
Chat Paper
正在生成论文摘要