Toward semantic data imputation for a dengue dataset
Knowledge-Based Systems(2020)
摘要
Missing data are a major problem that affects data analysis techniques for forecasting. Traditional methods suffer from poor performance in predicting missing values using simple techniques, e.g., mean and mode. In this paper, we present and discuss a novel method of imputing missing values semantically with the use of an ontology model. We make three new contributions to the field: first, an improvement in the efficiency of predicting missing data utilizing Particle Swarm Optimization (PSO), which is applied to the numerical data cleansing problem, with the performance of PSO being enhanced using K-means to help determine the fitness value. Second, the incorporation of an ontology with PSO for the purpose of narrowing the search space, to make PSO provide greater accuracy in predicting numerical missing values while quickly converging on the answer. Third, the facilitation of a framework to substitute nominal data that are lost from the dataset using the relationships of concepts and a reasoning mechanism concerning the knowledge-based model. The experimental results indicated that the proposed method could estimate missing data more efficiently and with less chance of error than conventional methods, as measured by the root mean square error.
更多查看译文
关键词
Missing data,Ontology,Data imputation,Data cleansing,Semantic
AI 理解论文
溯源树
样例
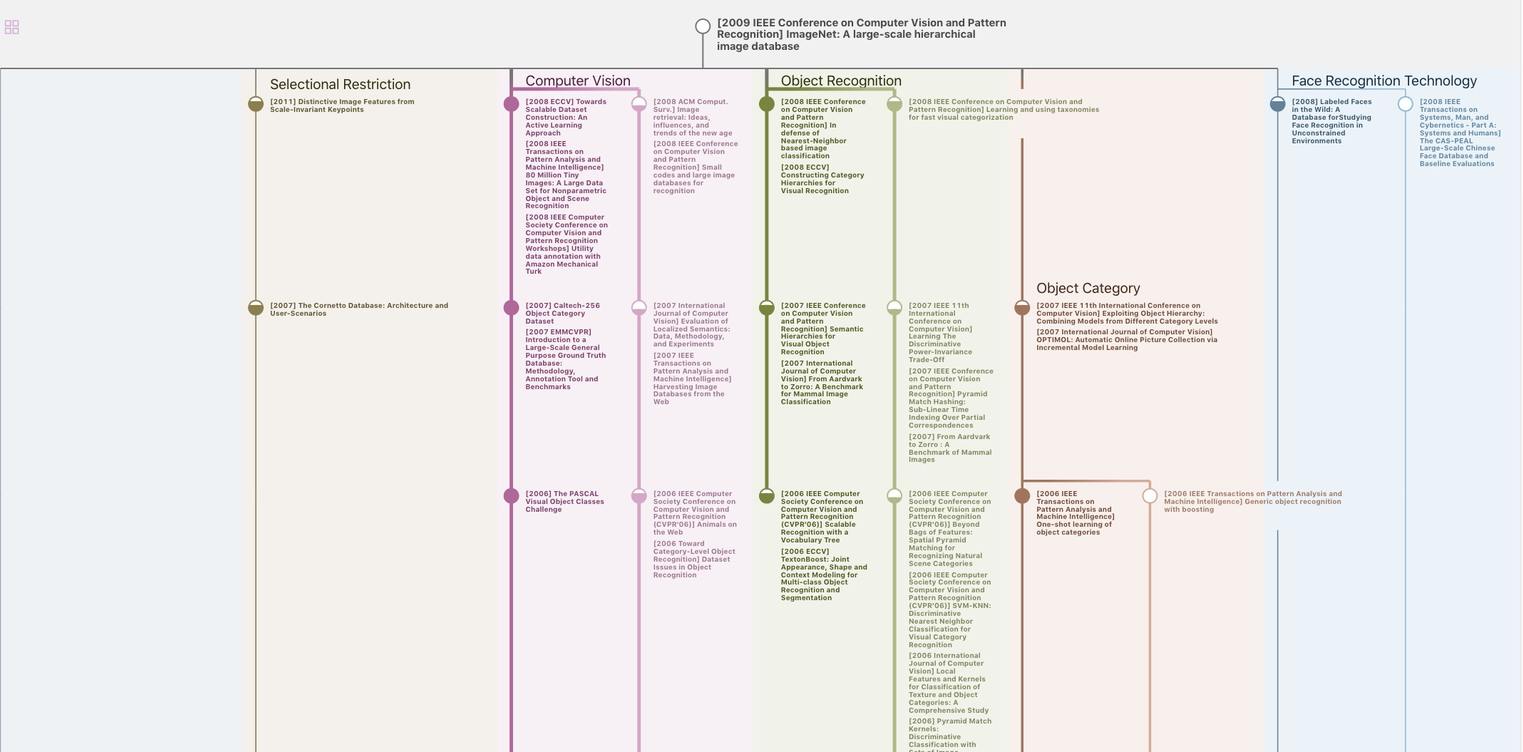
生成溯源树,研究论文发展脉络
Chat Paper
正在生成论文摘要