Robust classification of 3D objects using discrete orthogonal moments and deep neural networks
MULTIMEDIA TOOLS AND APPLICATIONS(2020)
摘要
In this paper, we propose a new model based on 3D discrete orthogonal moments and deep neural networks (DNN) to improve the classification accuracy of 3D objects under geometric transformations and noise. However, the utilization of moment invariants presents some drawbacks: They can’t describe the object efficiently, and their computation process is time consuming. Discrete orthogonal moments have the property to extract pertinent features from an image even in lower orders and are robust to noise. The main goal of this work is to investigate the robustness of the proposed model to geometric transformations like translation, scale and rotation and noisy conditions. The experiment simulations are conducted on datasets formed by applying some geometric transformations and noise on selected objects from McGill database. The obtained results indicate that the proposed model achieves high performance classification rates, robust to geometric transformations and noise degradation than other methods based on moment invariants.
更多查看译文
关键词
3D discrete orthogonal moments,3D object classification,Geometric transformations,Noisy conditions,Deep neural networks
AI 理解论文
溯源树
样例
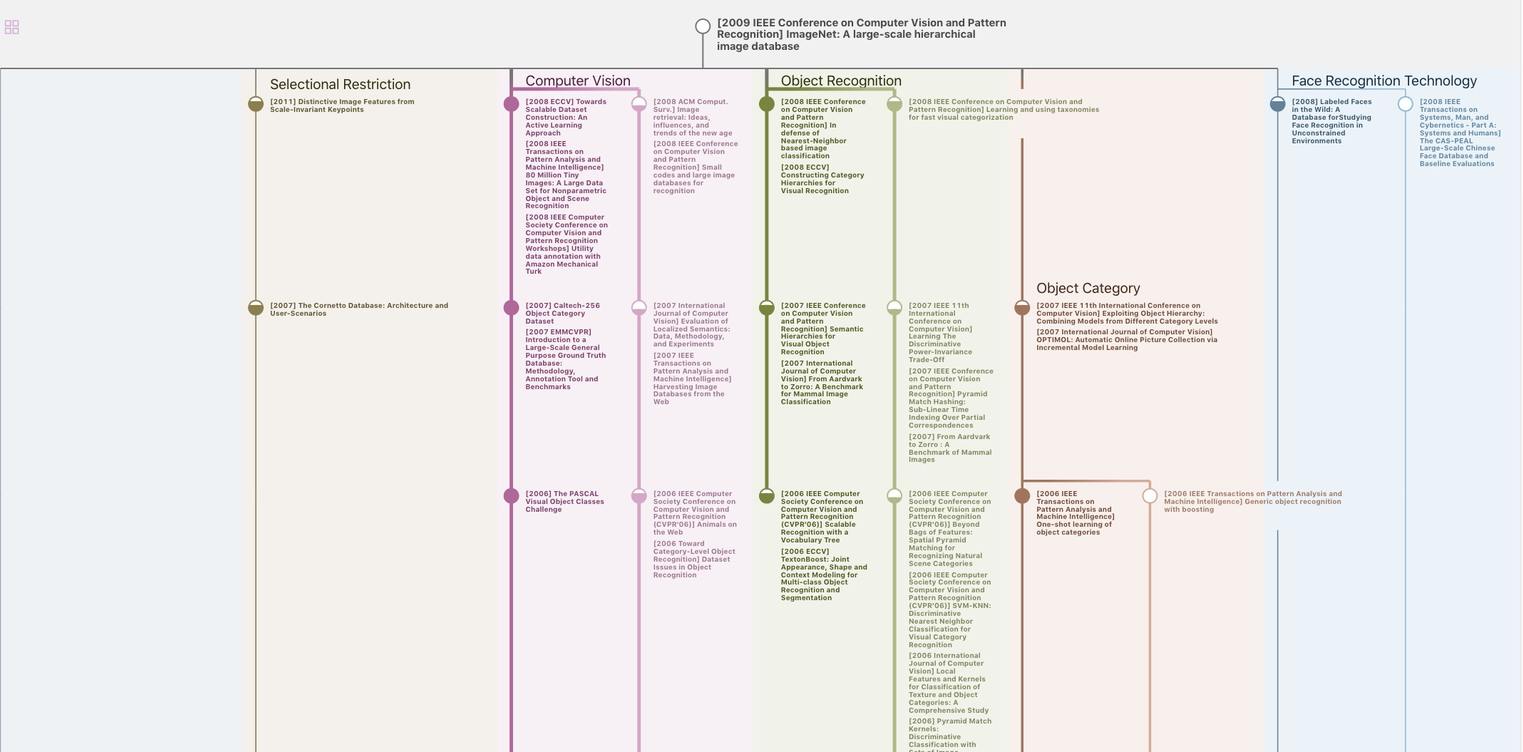
生成溯源树,研究论文发展脉络
Chat Paper
正在生成论文摘要