Estimation and inference in partially functional linear regression with multiple functional covariates
Journal of Statistical Planning and Inference(2020)
摘要
In this paper, we consider the estimation and inference in partially functional linear regression with multiple functional covariates. We estimate the parameters and the slope functions by using functional principal component analysis (FPCA) approach to each functional covariate; establish the asymptotic distribution for the proposed estimators and investigate the semiparametric efficiency. We derive the rates of convergence for the estimators of the slope functions, and prove that the rates are optimal. We also develop a linear hypothesis test for the parametric component, and construct confidence bands centered at FPCA-based estimator for the slope functions and verify its asymptotic validity. The performance of the proposed procedures is illustrated via simulation studies and an analysis of a diffusion tensor imaging data application.
更多查看译文
关键词
Asymptotic normality,Confidence band,Functional linear regression,Functional principal component analysis,Hypothesis testing,Semiparametric efficiency
AI 理解论文
溯源树
样例
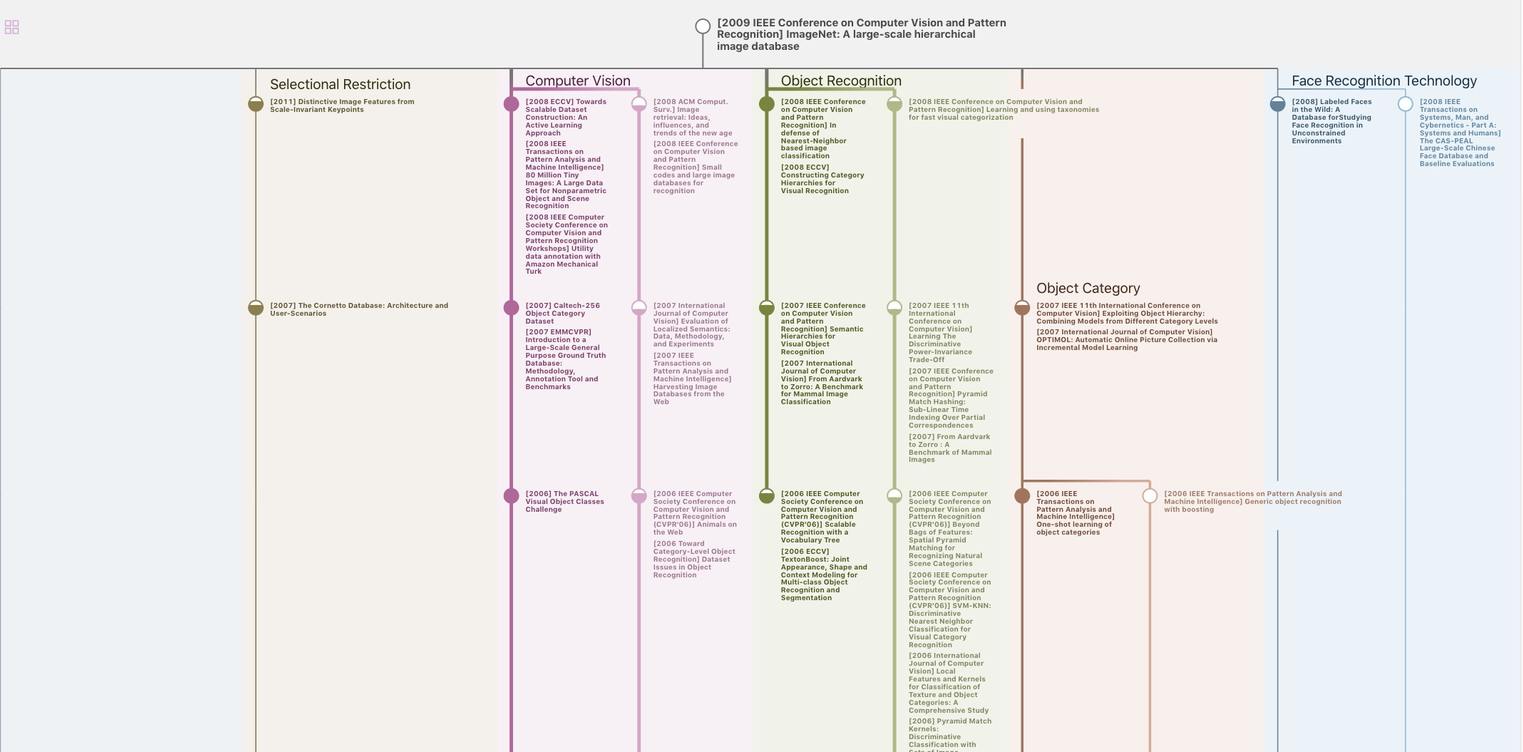
生成溯源树,研究论文发展脉络
Chat Paper
正在生成论文摘要