An Effective Model For Sms Spam Detection Using Content-Based Features And Averaged Neural Network
INTERNATIONAL JOURNAL OF ENGINEERING(2020)
摘要
In recent years, there has been considerable interest among people to use short message service (SMS) as one of the essential and straightforward communications services on mobile devices. The increased popularity of this service also increased the number of mobile devices attacks such as SMS spam messages. SMS spam messages constitute a real problem to mobile subscribers; this worries telecommunication service providers as it disturbs their customers and causes them to lose business. Therefore, in this paper, we proposed a novel machine learning method for detection of SMS spam messages. The proposed model contains two main stages: feature extraction and decision making. In the first stage, we have extracted relevant features from the dataset based on the characteristics of spam and legitimate messages to reduce the complexity and improve performance of the model. Then, an averaged neural network model was applied on extracted features to classify messages into either spam or legitimate classes. The method is evaluated in terms of accuracy and F-measure metrics on a real-world SMS dataset with over 5000 messages. Moreover, the achieved results were compared against three recently published works. Our results show that the proposed approach achieved successfully high detection rates in terms of F-measure and classification accuracy, compared with other considered researches.
更多查看译文
关键词
Spam, Mobile Spam, SMS Spam, Anti-Spam, Machine Learning
AI 理解论文
溯源树
样例
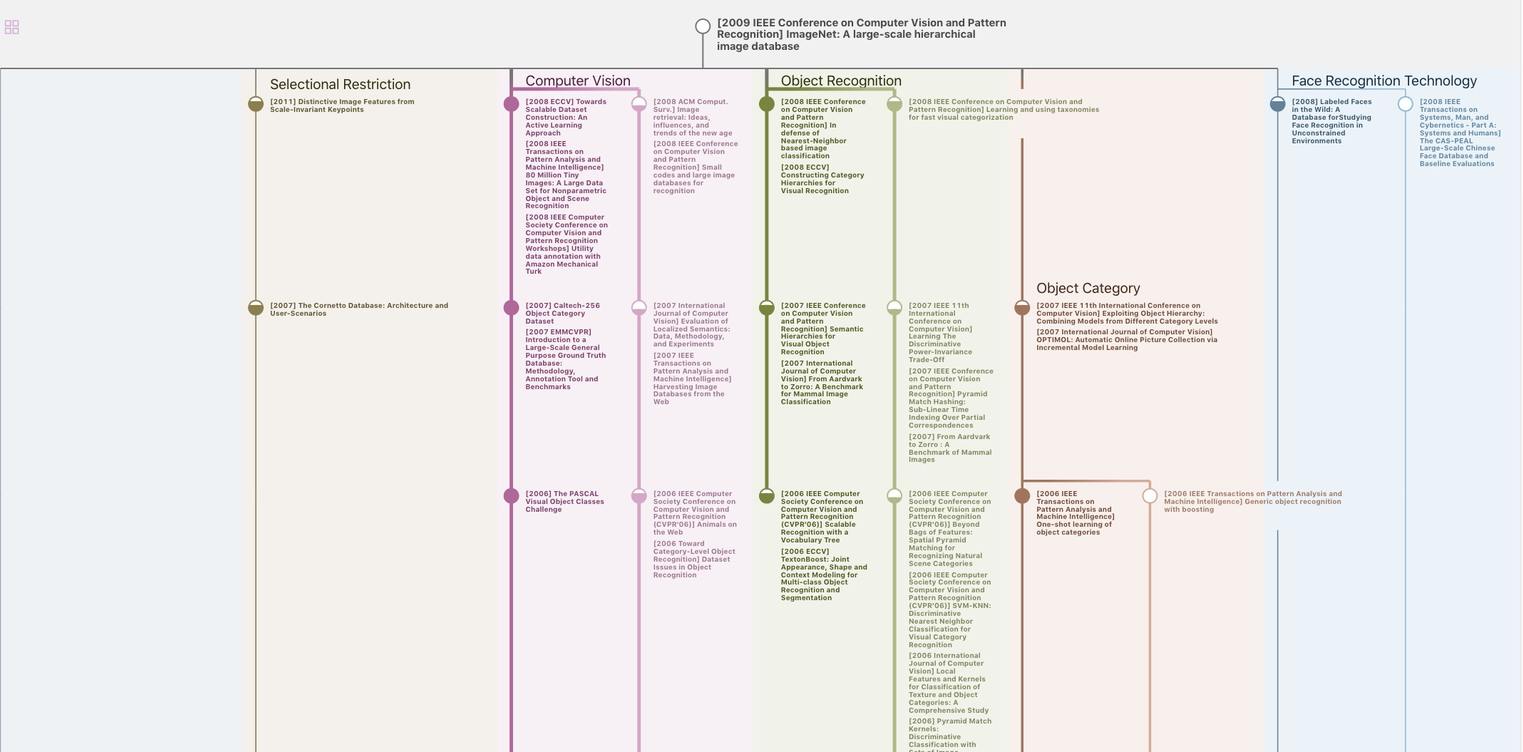
生成溯源树,研究论文发展脉络
Chat Paper
正在生成论文摘要