LOVBench: Ontology Ranking Benchmark
WWW '20: The Web Conference 2020 Taipei Taiwan April, 2020(2020)
摘要
Ontology search and ranking are key building blocks to establish and reuse shared conceptualizations of domain knowledge on the Web. However, the effectiveness of proposed ontology ranking models is difficult to compare since these are often evaluated on diverse datasets that are limited by their static nature and scale. In this paper, we first introduce the LOVBench dataset as a benchmark for ontology term ranking. With inferred relevance judgments for more than 7000 queries, LOVBench is large enough to perform a comparison study using learning to rank (LTR) with complex ontology ranking models. Instead of relying on relevance judgments from a few experts, we consider implicit feedback from many actual users collected from the Linked Open Vocabularies (LOV) platform. Our approach further enables continuous updates of the benchmark, capturing the evolution of ontologies’ relevance in an ever-changing data community. Second, we compare the performance of several feature configurations from the literature using LOVBench in LTR settings and discuss the results in the context of the observed real-world user behavior. Our experimental results show that feature configurations which are (i) well-suited to the user behavior, (ii) cover all features types, and (iii) consider decomposition of features can significantly improve the ranking performance.
更多查看译文
关键词
ontology search, ground truth mining, ontology reuse, semantic interoperability, learning to rank
AI 理解论文
溯源树
样例
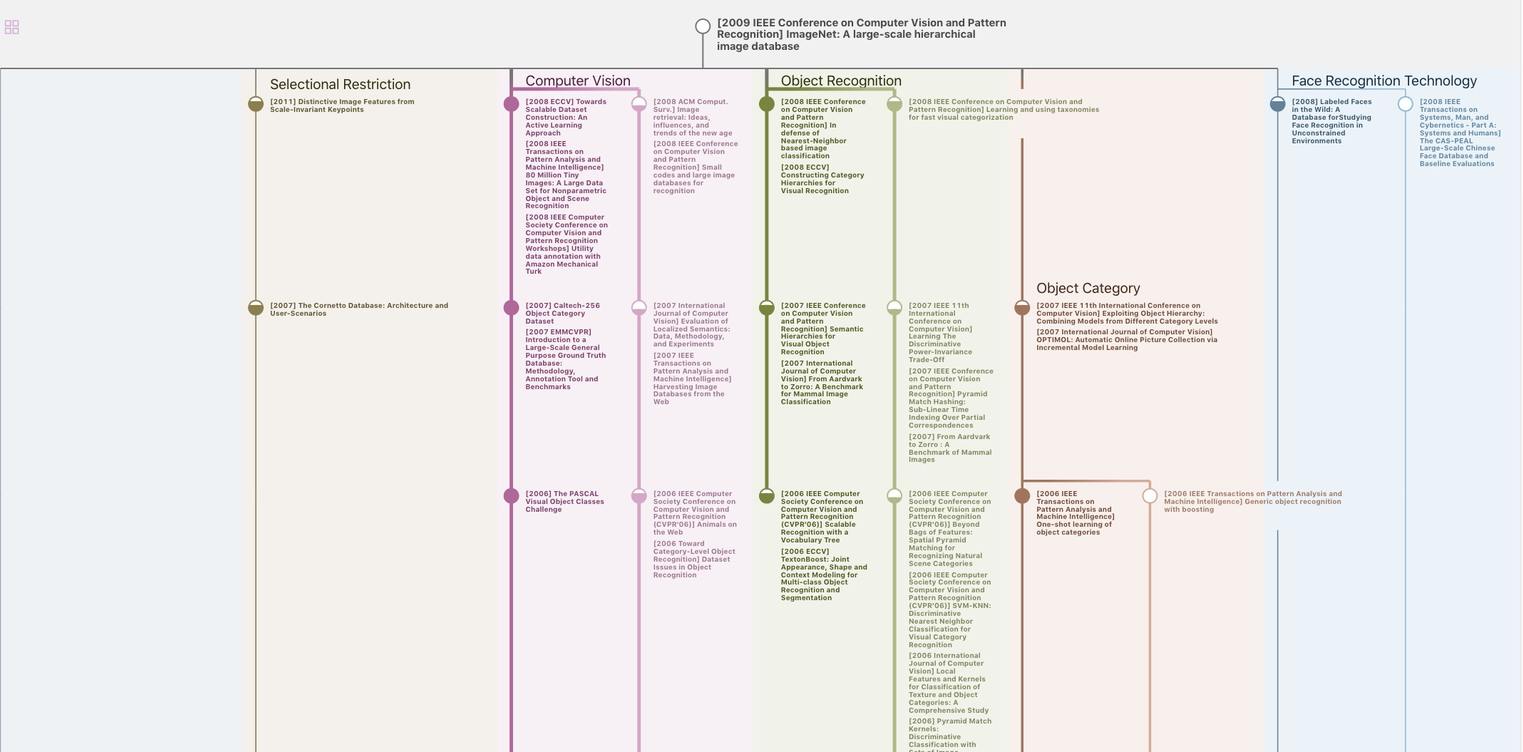
生成溯源树,研究论文发展脉络
Chat Paper
正在生成论文摘要