Machine-learning approaches to substance-abuse research: emerging trends and their implications.
CURRENT OPINION IN PSYCHIATRY(2020)
摘要
PURPOSE OF REVIEW:To provide an accessible overview of some of the most recent trends in the application of machine learning to the field of substance use disorders and their implications for future research and practice.
RECENT FINDINGS:Machine-learning (ML) techniques have recently been applied to substance use disorder (SUD) data for multiple predictive applications including detecting current abuse, assessing future risk and predicting treatment success. These models cover a wide range of machine-learning techniques and data types including physiological measures, longitudinal surveys, treatment outcomes, national surveys, medical records and social media.
SUMMARY:The application of machine-learning models to substance use disorder data shows significant promise, with some use cases and data types showing high predictive accuracy, particularly for models of physiological and behavioral measures for predicting current substance use, portending potential clinical diagnostic applications; however, these results are uneven, with some models performing poorly or at chance, a limitation likely reflecting insufficient data and/or weak validation methods. The field will likely benefit from larger and more multimodal datasets, greater standardization of data recording and rigorous testing protocols as well as greater use of modern deep neural network models applied to multimodal unstructured datasets.
更多查看译文
关键词
detection, diagnosis, machine learning, predictive modelling, substance use disorders, treatment outcomes
AI 理解论文
溯源树
样例
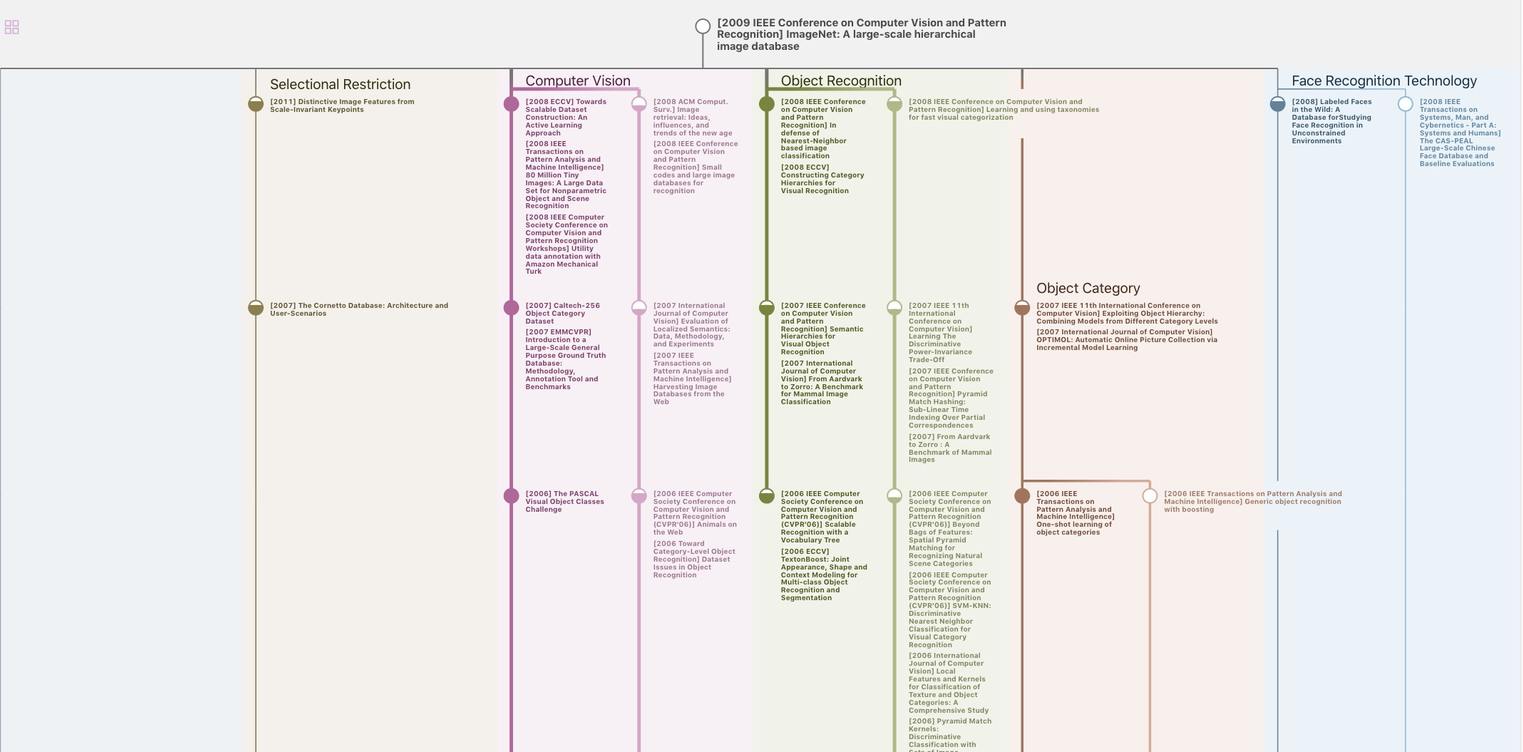
生成溯源树,研究论文发展脉络
Chat Paper
正在生成论文摘要