Lr3m: Robust Low-Light Enhancement Via Low-Rank Regularized Retinex Model
IEEE TRANSACTIONS ON IMAGE PROCESSING(2020)
摘要
Noise causes unpleasant visual effects in low-light image/video enhancement. In this paper, we aim to make the enhancement model and method aware of noise in the whole process. To deal with heavy noise which is not handled in previous methods, we introduce a robust low-light enhancement approach, aiming at well enhancing low-light images/videos and suppressing intensive noise jointly. Our method is based on the proposed Low-Rank Regularized Retinex Model (LR3M), which is the first to inject low-rank prior into a Retinex decomposition process to suppress noise in the reflectance map. Our method estimates a piece-wise smoothed illumination and a noise-suppressed reflectance sequentially, avoiding remaining noise in the illumination and reflectance maps which are usually presented in alternative decomposition methods. After getting the estimated illumination and reflectance, we adjust the illumination layer and generate our enhancement result. Furthermore, we apply our LR3M to video low-light enhancement. We consider inter-frame coherence of illumination maps and find similar patches through reflectance maps of successive frames to form the low-rank prior to make use of temporal correspondence. Our method performs well for a wide variety of images and videos, and achieves better quality both in enhancing and denoising, compared with the state-of-the-art methods.
更多查看译文
关键词
Lighting, Robustness, Noise reduction, Histograms, Minimization, Visualization, Estimation, Low-light enhancement, denoising, retinex model, low-rank decomposition
AI 理解论文
溯源树
样例
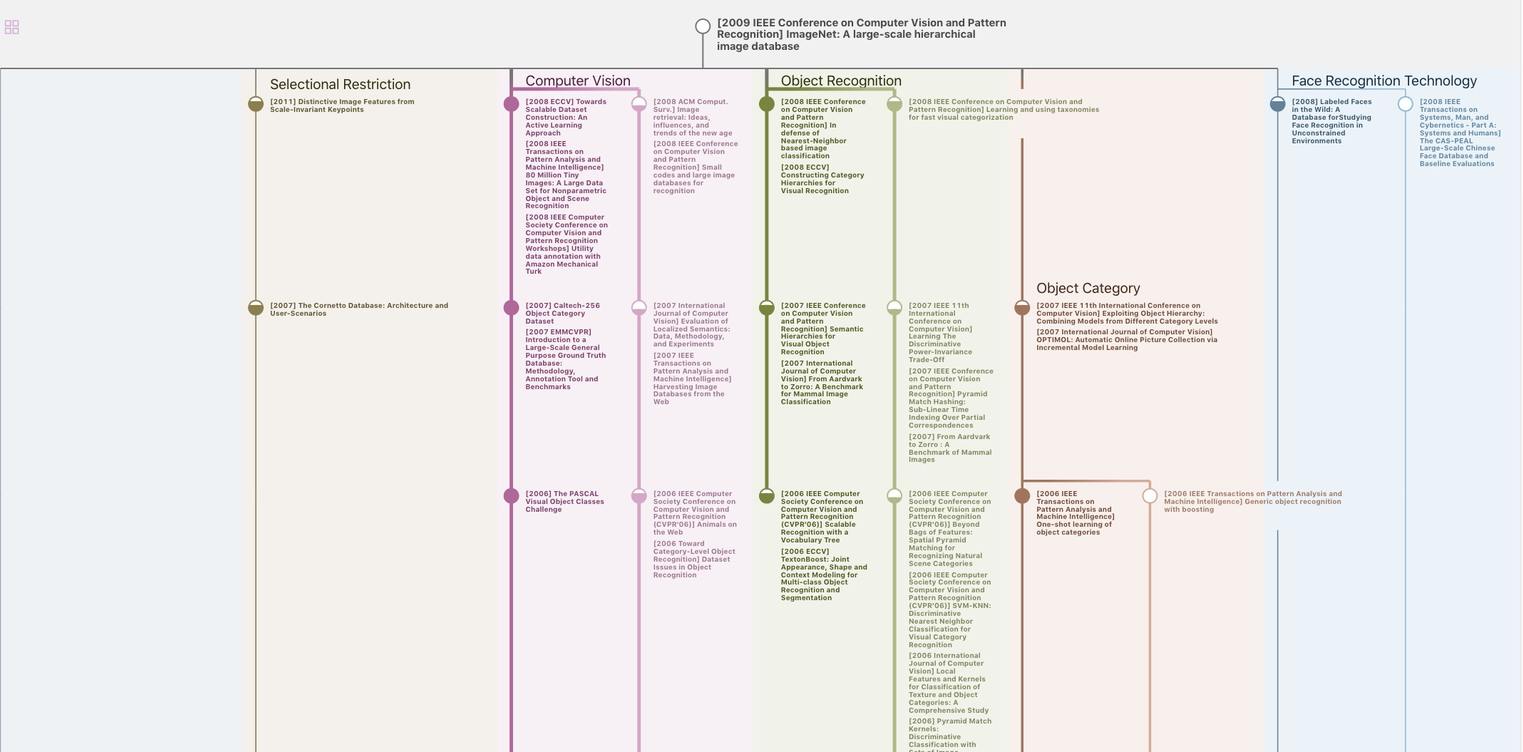
生成溯源树,研究论文发展脉络
Chat Paper
正在生成论文摘要