Robust Kernelized Multiview Self-Representation for Subspace Clustering
IEEE Transactions on Neural Networks and Learning Systems(2021)
摘要
In this article, we propose a multiview self-representation model for nonlinear subspaces clustering. By assuming that the heterogeneous features lie within the union of multiple linear subspaces, the recent multiview subspace learning methods aim to capture the complementary and consensus from multiple views to boost the performance. However, in real-world applications, data feature usually resides in multiple nonlinear subspaces, leading to undesirable results. To this end, we propose a kernelized version of tensor-based multiview subspace clustering, which is referred to as Kt-SVD-MSC, to jointly learn self-representation coefficients in mapped high-dimensional spaces and multiple views correlation in unified tensor space. In view-specific feature space, a kernel-induced mapping is introduced for each view to ensure the separability of self-representation coefficients. In unified tensor space, a new kind of tensor low-rank regularizer is employed on the rotated self-representation coefficient tensor to preserve the global consistency across different views. We also derive an algorithm to efficiently solve the optimization problem with all the subproblems having closed-form solutions. Furthermore, by incorporating the nonnegative and sparsity constraints, the proposed method can be easily extended to a useful variant, meaning that several useful variants can be easily constructed in a similar way. Extensive experiments of the proposed method are tested on eight challenging data sets, in which a significant (even a breakthrough) advance over state-of-the-art multiview clustering is achieved.
更多查看译文
关键词
Kernelization,multiview subspace learning,nonlinear subspace clustering,tensor singular value decomposition (t-SVD)
AI 理解论文
溯源树
样例
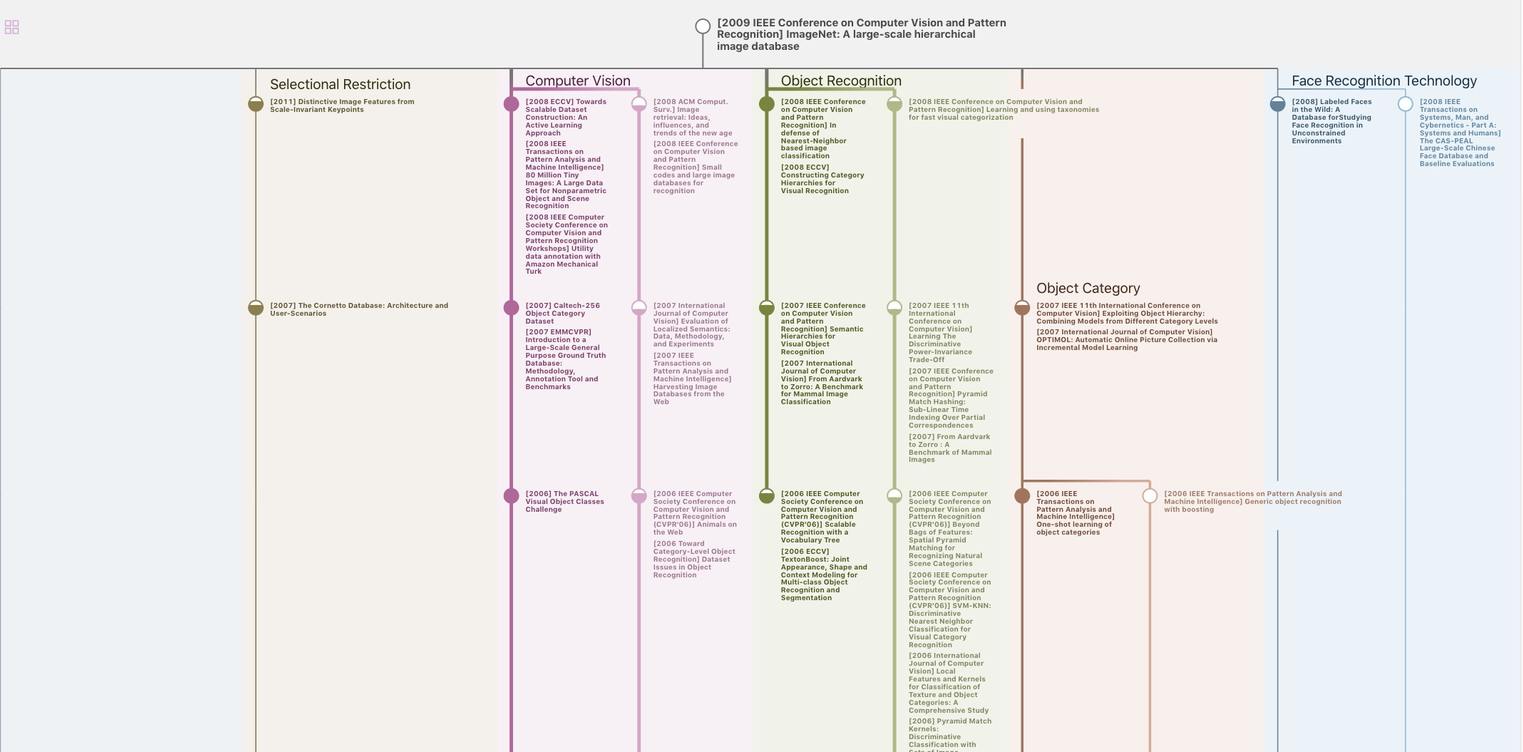
生成溯源树,研究论文发展脉络
Chat Paper
正在生成论文摘要