Principal Neighbourhood Aggregation for Graph Nets
NIPS 2020(2020)
摘要
Graph Neural Networks (GNNs) have been shown to be effective models for different predictive tasks on graph-structured data. Recent work on their expressive power has focused on isomorphism tasks and countable feature spaces. We extend this theoretical framework to include continuous features - which occur regularly in real-world input domains and within the hidden layers of GNNs - and we demonstrate the requirement for multiple aggregation functions in this setting. Accordingly, we propose Principal Neighbourhood Aggregation (PNA), a novel architecture combining multiple aggregators with degree-scalers (which generalize the sum aggregator). Finally, we compare the capacity of different models to capture and exploit the graph structure via a benchmark containing multiple tasks taken from classical graph theory, which demonstrates the capacity of our model.
更多查看译文
关键词
aggregation
AI 理解论文
溯源树
样例
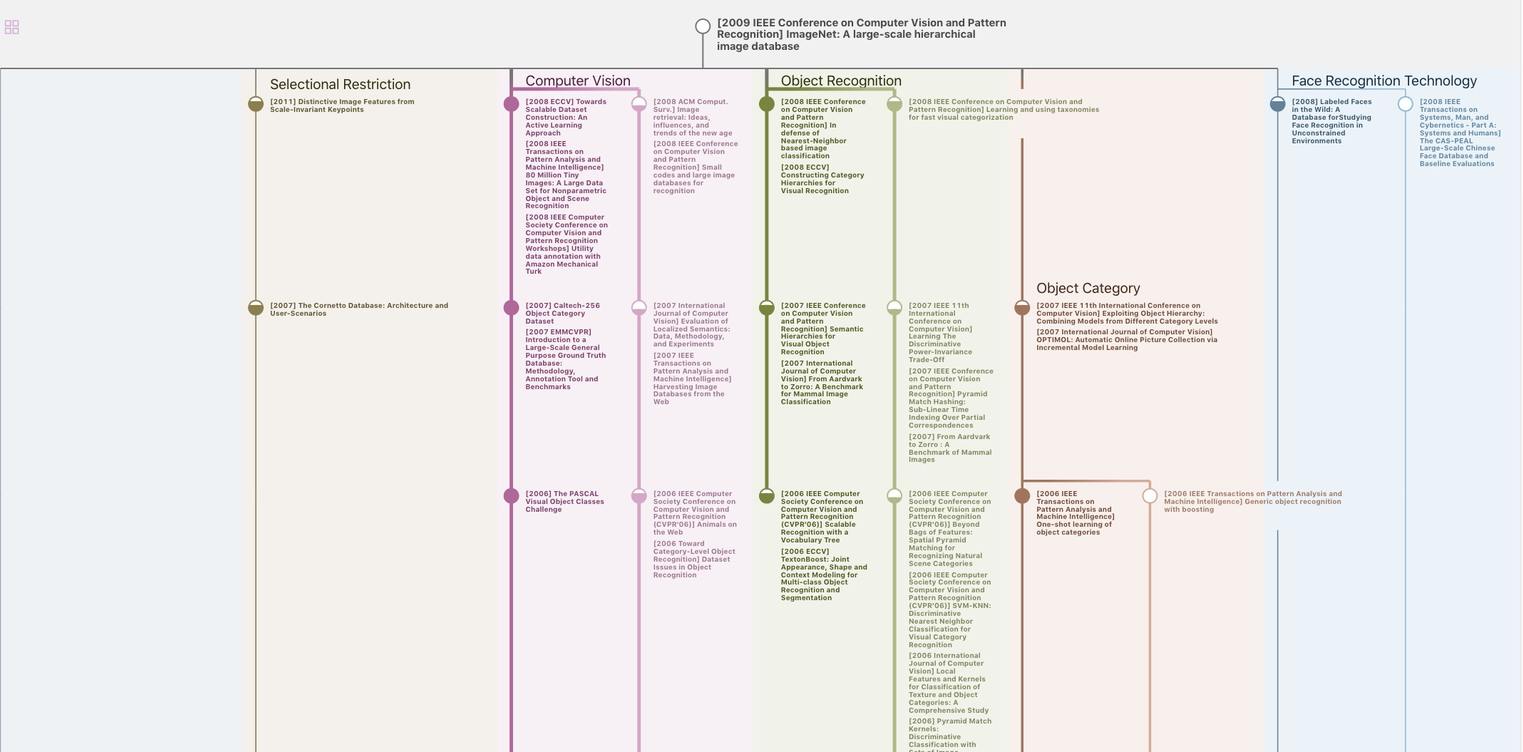
生成溯源树,研究论文发展脉络
Chat Paper
正在生成论文摘要