Deep Learning for RF Fingerprinting: A Massive Experimental Study
IEEE Internet of Things Magazine(2020)
摘要
RF fingerprinting is a key security mechanism that allows device identification by learning unchanging, hardware-based characteristics of the transmitter. In this article, we demonstrate how machine learning techniques impact RF fingerprinting by analyzing a dataset of 400 GB of in-phase (I) and quadrature (Q) signal data transmitted by 10,000 radios. Our deep convolutional neural network architectures take raw and processed IQ samples as input to identify devices under a variety of practical conditions, including changing channels, noise levels, training data sizes, and computational overheads, among others. The contributions of the article are as follows: (i) This is the first work, to the best of our knowledge, reporting on RF fingerprinting and scalability issues on very large device populations, in the range of 50-10,000 devices. (ii) We investigate how to mitigate the effect of the wireless channel through a feature engineering step that we refer to as partial equalization. (iii) We provide a comprehensive performance evaluation of both a custom-designed and a modified ResNet architecture, with insights on which one may be preferred under specific environmental conditions.
更多查看译文
关键词
Radio frequency,Computer architecture,Training,Wireless fidelity,Fingerprint recognition,Performance evaluation,Wireless communication
AI 理解论文
溯源树
样例
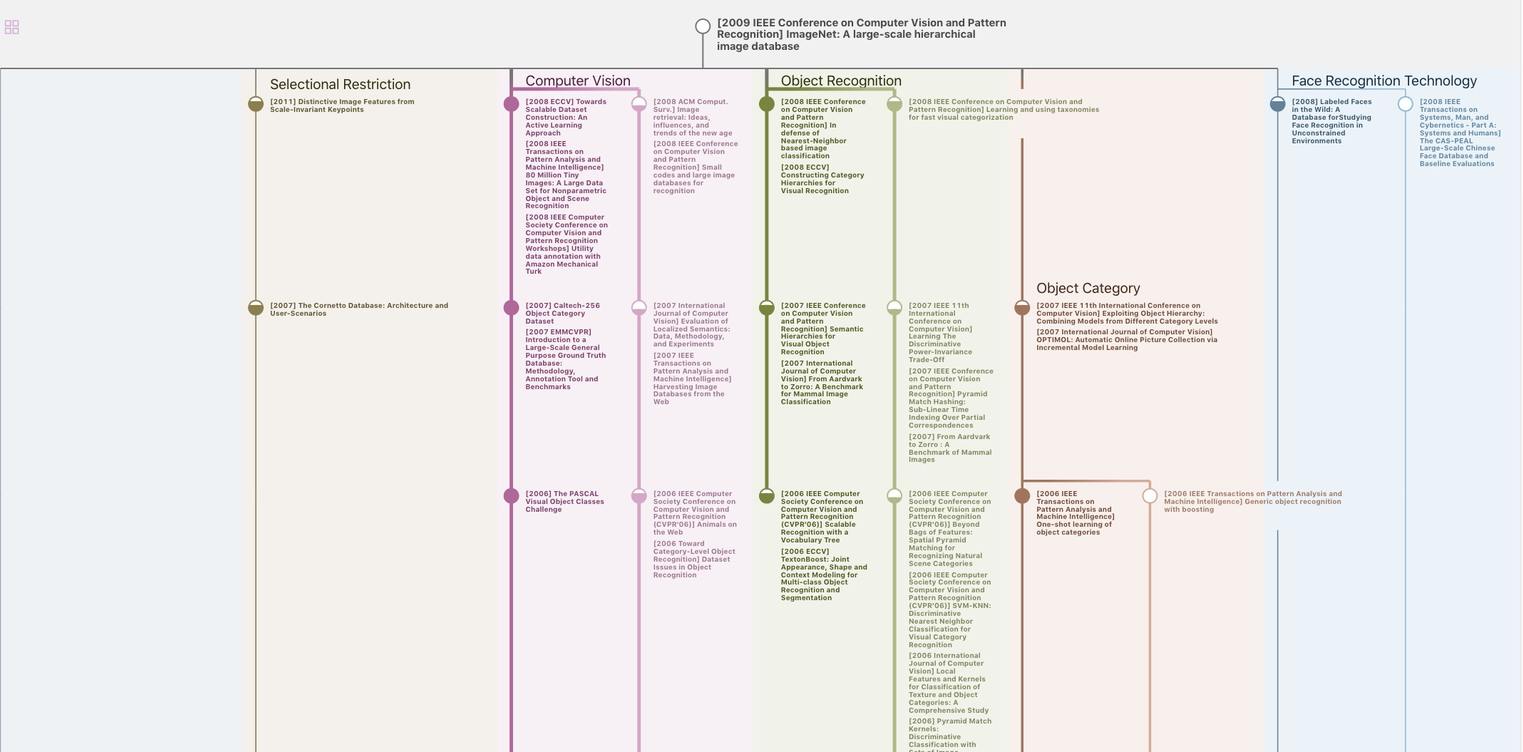
生成溯源树,研究论文发展脉络
Chat Paper
正在生成论文摘要