Learning Over Dirty Data Without Cleaning
SIGMOD/PODS '20: International Conference on Management of Data Portland OR USA June, 2020(2020)
摘要
Real-world datasets are dirty and contain many errors, such as violations of integrity constraints and entity duplicates. Learning over dirty databases may result in inaccurate models. Data scientists spend most of their time on preparing and repairing data errors to create clean databases for learning. Moreover, as the information required to repair these errors is not often available, there may be numerous possible clean versions for a dirty database. We propose Dirty Learn, DLearn, a novel learning system that learns directly over dirty databases effectively and efficiently without any preprocessing. DLearn leverages database constraints to learn accurate relational models over inconsistent and heterogeneous data. Its learned models represent patterns over all possible clean versions of the data in a usable form. Our empirical study indicates that DLearn learns accurate models over large real-world databases efficiently.
更多查看译文
关键词
dirty data,cleaning,learning
AI 理解论文
溯源树
样例
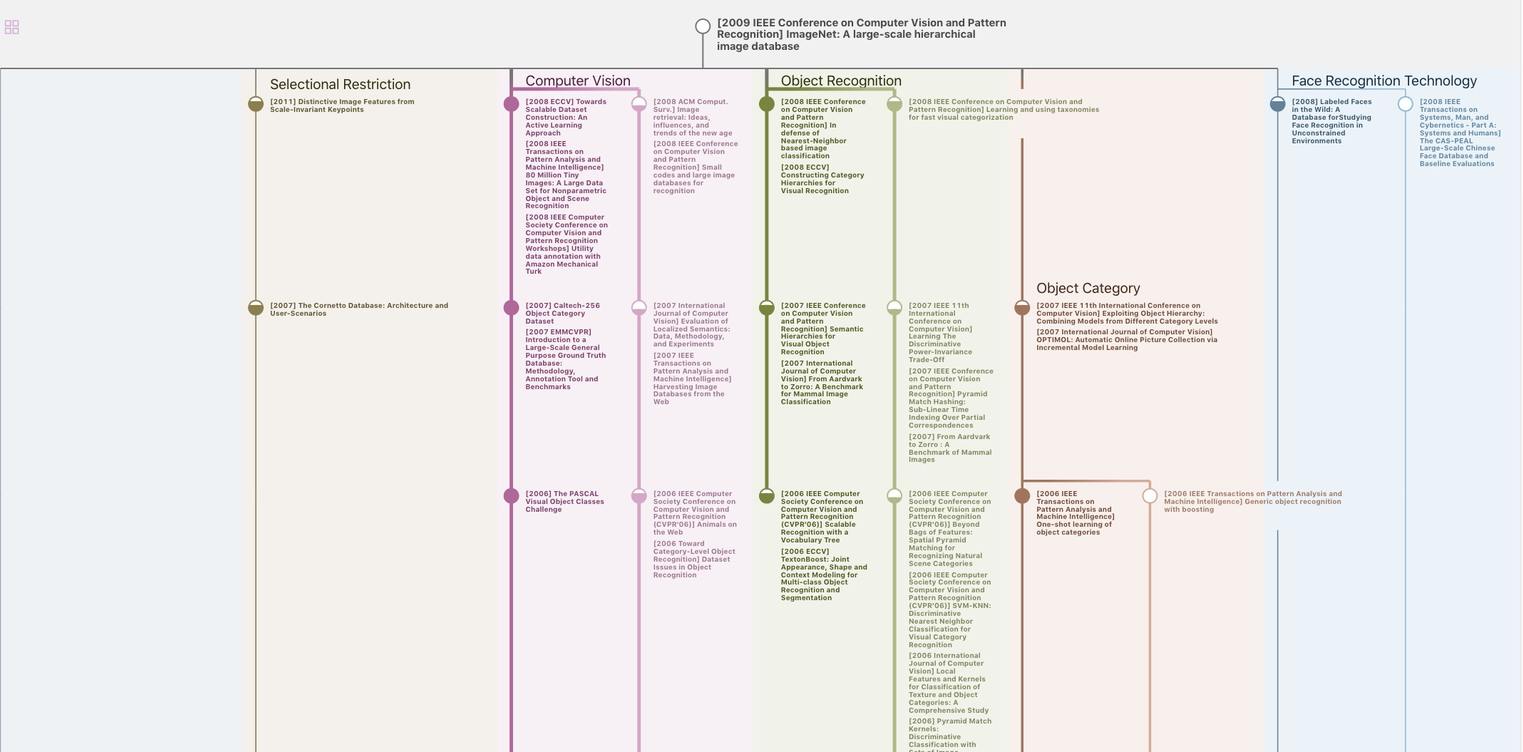
生成溯源树,研究论文发展脉络
Chat Paper
正在生成论文摘要