Google Landmarks Dataset V2 A Large-Scale Benchmark For Instance-Level Recognition And Retrieval
2020 IEEE/CVF CONFERENCE ON COMPUTER VISION AND PATTERN RECOGNITION (CVPR)(2020)
摘要
While image retrieval and instance recognition techniques are progressing rapidly, there is a need for challenging datasets to accurately measure their performance - while posing novel challenges that are relevant for practical applications. We introduce the Google Landmarks Dataset v2 (GLDv2), a new benchmark for large-scale, fine-grained instance recognition and image retrieval in the domain of human-made and natural landmarks. GLDv2 is the largest such dataset to date by a large margin, including over 5M images and 200k distinct instance labels. Its test set consists of 118k images with ground truth annotations for both the retrieval and recognition tasks. The ground truth construction involved over 800 hours of human annotator work. Our new dataset has several challenging properties inspired by realworld applications that previous datasets did not consider: An extremely long-tailed class distribution, a large fraction of out-of-domain test photos and large intra-class variability. The dataset is sourced from Wikimedia Commons, the world's largest crowdsourced collection of landmark photos. We provide baseline results for both recognition and retrieval tasks based on state-of-the-art methods as well as competitive results from a public challenge. We further demonstrate the suitability of the dataset for transfer learning by showing that image embeddings trained on it achieve competitive retrieval performance on independent datasets. The dataset images, ground-truth and metric scoring code are available at https://github.com/cvdfoundation/google-landmark.
更多查看译文
关键词
Google landmarks dataset,distinct instance labels,dataset images,independent datasets,competitive retrieval performance,image embeddings,public challenge,retrieval tasks,landmark photos,out-of-domain test photos,challenging properties,human annotator work,ground truth construction,ground truth annotations,natural landmarks,fine-grained instance recognition,GLDv2,instance recognition techniques,image retrieval,large-scale benchmark
AI 理解论文
溯源树
样例
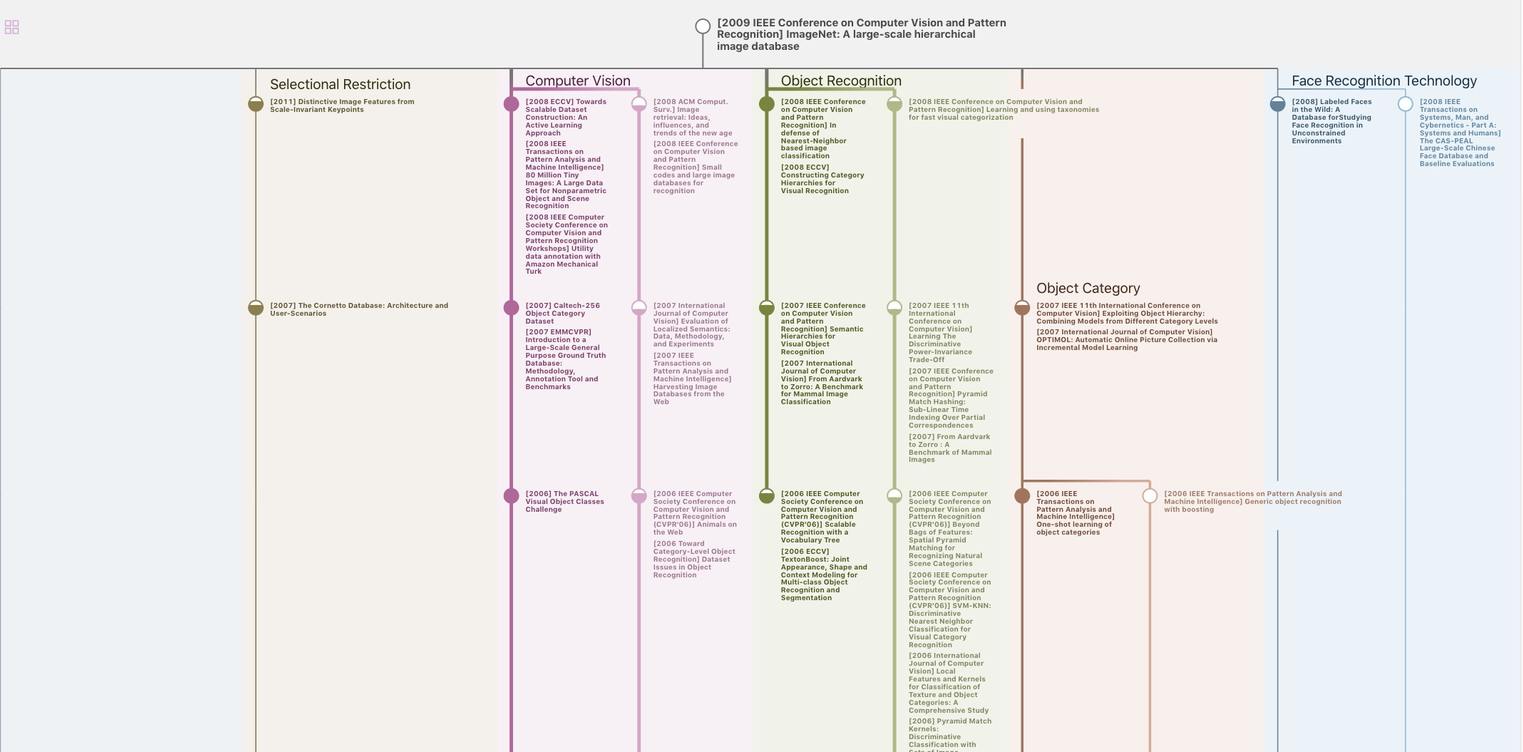
生成溯源树,研究论文发展脉络
Chat Paper
正在生成论文摘要