Friend Recommendation Based on Multi-Social Graph Convolutional Network.
IEEE ACCESS(2020)
摘要
Friend recommendations based on social relationships have attracted thousands of research under the rapid development of social networks. However, most of the existing friend recommendation methods use user attributes or a single social network, while rarely integrating multiple social relationships to enhance the representation. This paper focuses on integrating various social relationships to guide the representation learning, and further generating personalized friend recommendations. We design an end-to-end framework based on multiple social networks to learn the potential features of users and construct a friend recommendation model named Multi-Social Graph Convolutional Network (MSGCN). It learns the features of higher-order neighbors from multiple social networks to enrich the representation of the target user based on the improved graph convolution neural network. In particular, some graph fusion strategies by adjusting and fusing the Laplace matrix of the graph are designed to integrate social relationships. Finally, we use Bayesian theory to transform friend recommendation into a sorting problem for personalized recommendation. The experimental results show that the proposed model outperforms the state-of-the-art methods.
更多查看译文
关键词
Social network services,Machine learning,Bayes methods,Neural networks,Task analysis,Transforms,Learning systems,Friend recommendation,multi-social graph convolutional network,social network
AI 理解论文
溯源树
样例
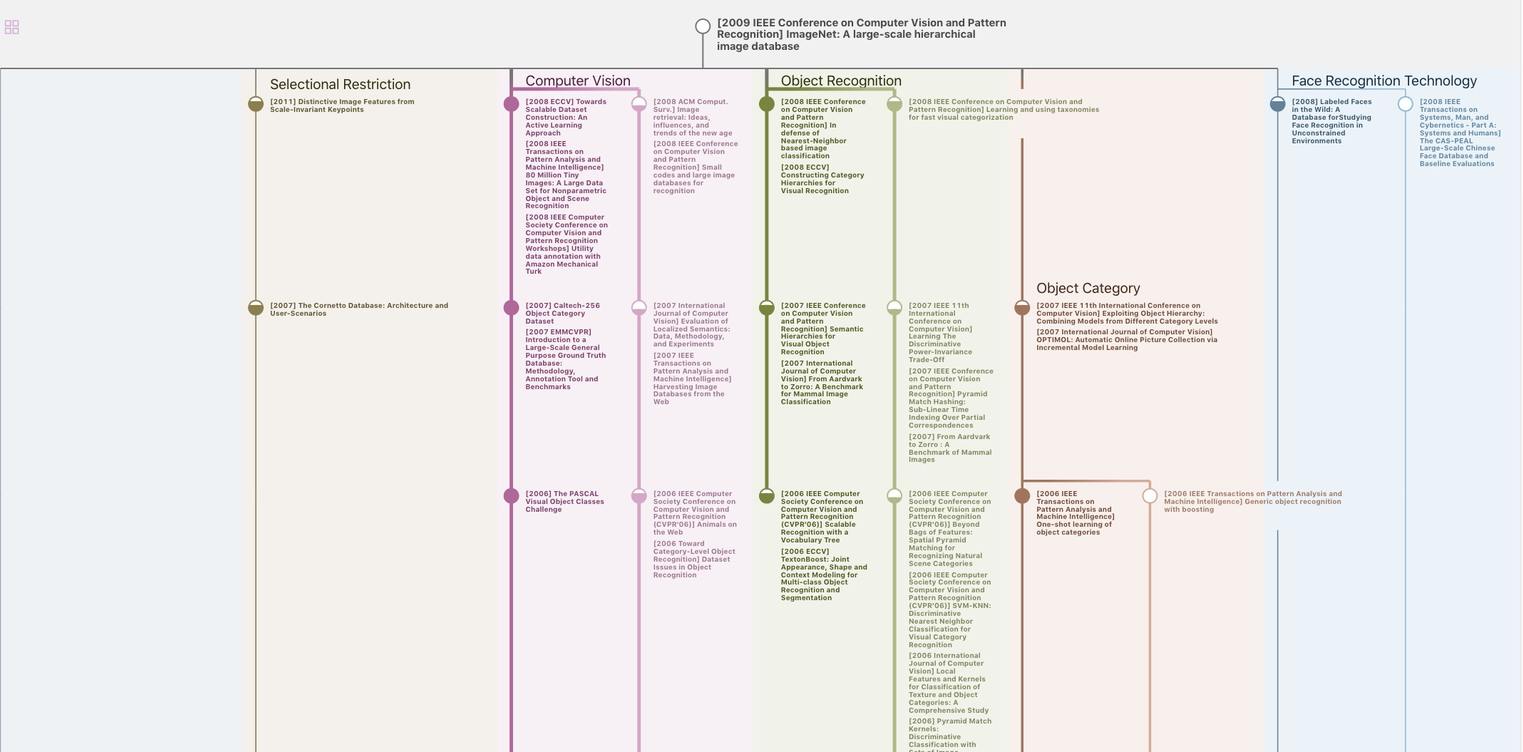
生成溯源树,研究论文发展脉络
Chat Paper
正在生成论文摘要