A Key-Phrase Aware End2end Neural Response Generation Model.
NLPCC (2)(2019)
摘要
Previous Seq2Seq models for chitchat assume that each word in the target sequence has direct corresponding relationship with words in the source sequence, and all the target words are equally important. However, it is invalid since sometimes only parts of the response are relevant to the message. For models with the above mentioned assumption, irrelevant response words might have a negative impact on the performance in semantic association modeling that is a core task for open-domain dialogue modeling. In this work, to address the challenge of semantic association modeling, we automatically recognize key-phrases from responses in training data, and then feed this supervision information into an enhanced key-phrase aware seq2seq model for better capability in semantic association modeling. This model consists of an encoder and a two-layer decoder, where the encoder and the first layer sub-decoder is mainly for learning semantic association and the second layer sub-decoder is for responses generation. Experimental results show that this model can effectively utilize the key phrase information for semantic association modeling, and it can significantly outperform baseline models in terms of response appropriateness and informativeness
.
更多查看译文
关键词
Key-phrase, End2end, Neural dialog model
AI 理解论文
溯源树
样例
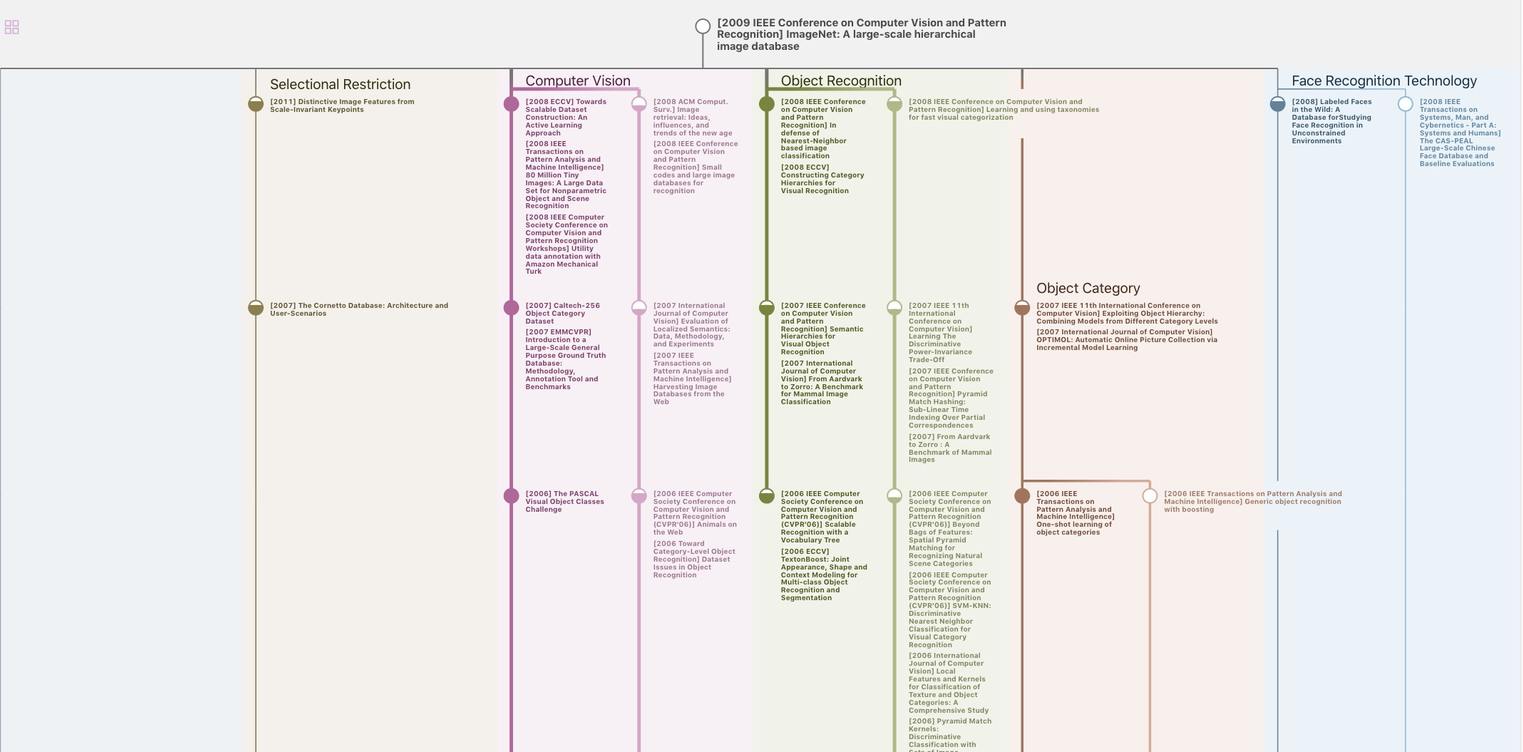
生成溯源树,研究论文发展脉络
Chat Paper
正在生成论文摘要