S2DNet: Learning Accurate Correspondences for Sparse-to-Dense Feature Matching
arxiv(2020)
摘要
Establishing robust and accurate correspondences is a fundamental backbone to many computer vision algorithms. While recent learning-based feature matching methods have shown promising results in providing robust correspondences under challenging conditions, they are often limited in terms of precision. In this paper, we introduce S2DNet, a novel feature matching pipeline, designed and trained to efficiently establish both robust and accurate correspondences. By leveraging a sparse-to-dense matching paradigm, we cast the correspondence learning problem as a supervised classification task to learn to output highly peaked correspondence maps. We show that S2DNet achieves state-of-the-art results on the HPatches benchmark, as well as on several long-term visual localization datasets.
更多查看译文
关键词
accurate correspondences,feature
AI 理解论文
溯源树
样例
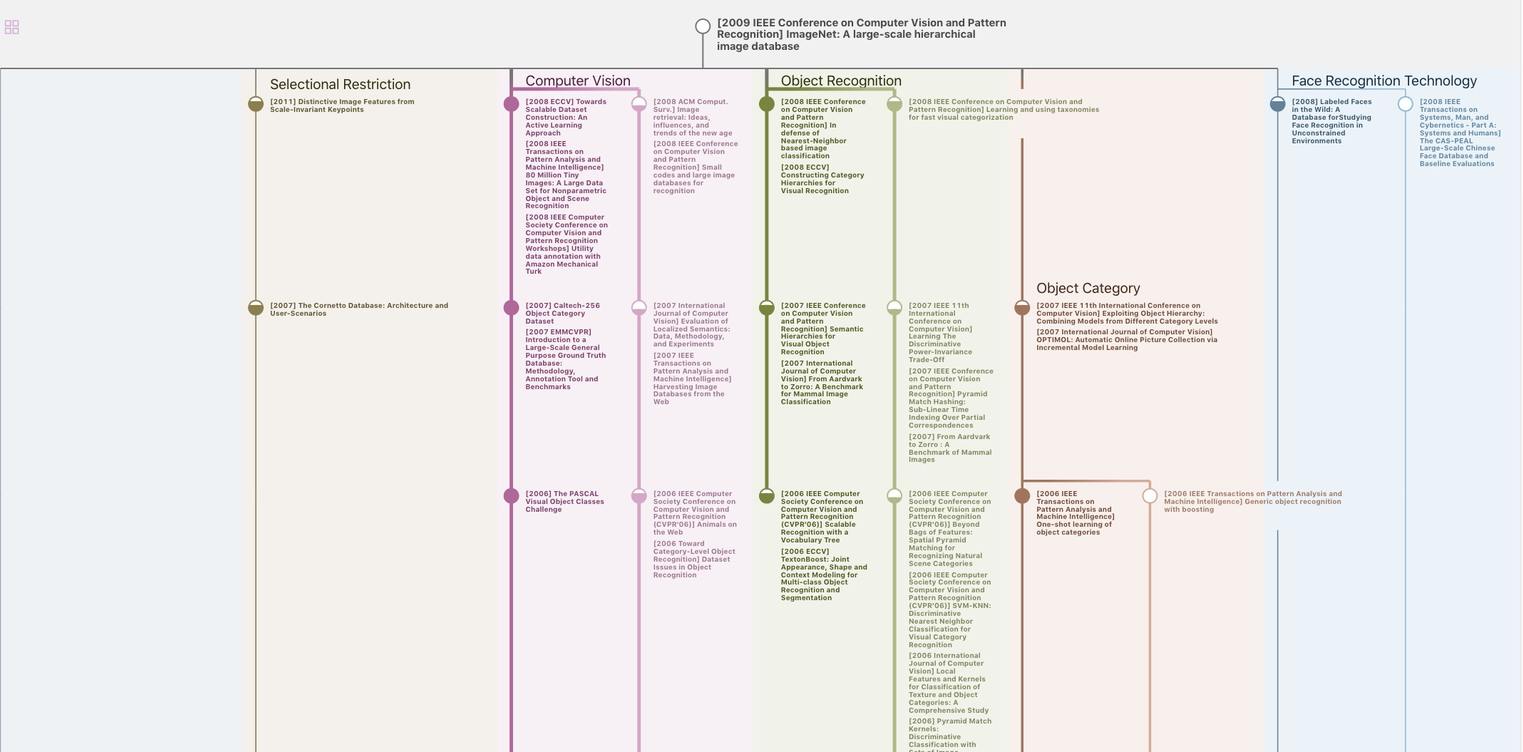
生成溯源树,研究论文发展脉络
Chat Paper
正在生成论文摘要