Workload-Aware Scheduling for Data Analytics upon Heterogeneous Storage
2019 IEEE Intl Conf on Parallel & Distributed Processing with Applications, Big Data & Cloud Computing, Sustainable Computing & Communications, Social Computing & Networking (ISPA/BDCloud/SocialCom/SustainCom)(2019)
摘要
A trend in nowadays data centers is that equipped with SSD, HDD, etc., heterogeneous storage devices are widely deployed to meet diverse demands of various big data workloads. Since the reading performance of various storage devices are quite different, traditional concurrent data fetching easily incurs unbalanced use among devices. As a result, the straggler in terms of the data fetching, derived from the unbalanced use, directly increases the overall latency of data analytics. To avoid such unbalanced use on fetching large volume of data concurrently from storage devices, we formulate Workload-Aware Scheduling problem for Heterogeneous storage devices (WASH), the goal of which is to minimize the maximum data fetching time for parallel data analytical tasks. We design a randomized algorithm (rWASH) to select a proper source device for each task based on delicate calculated probabilities, which can be proved concentrated on its optimum with high probability. Extensive experiments show that rWASH reduces the average data fetching time for tasks by up to 55% over the state-of-the-art algorithms.
更多查看译文
关键词
big data analytics, heterogeneous storage devices, workload aware scheduling
AI 理解论文
溯源树
样例
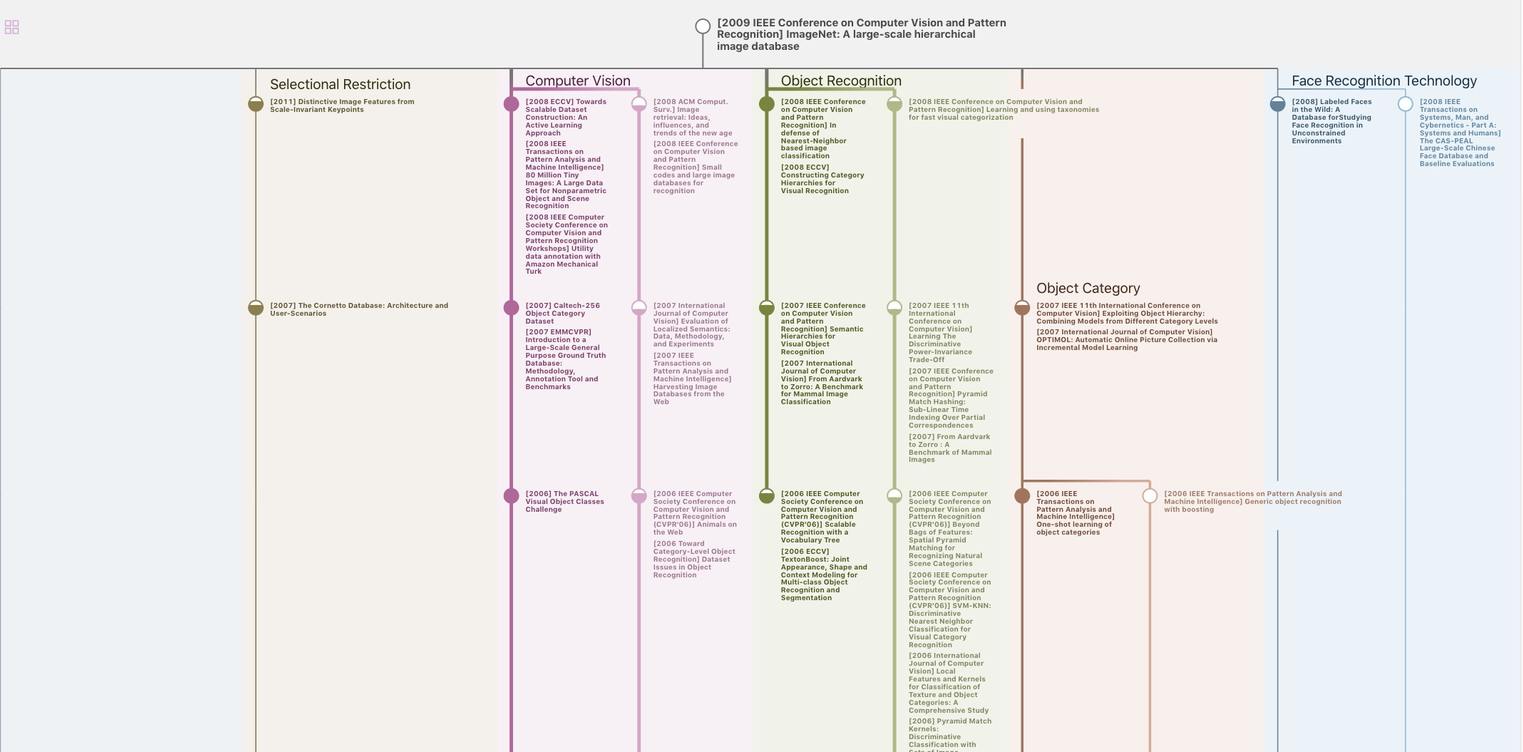
生成溯源树,研究论文发展脉络
Chat Paper
正在生成论文摘要