Metaplasticity in Multistate Memristor Synaptic Networks
ISCAS(2020)
摘要
Recent studies have shown that metaplastic synapses can retain information longer than simple binary synapses and are beneficial for continual learning. In this paper, we explore the multistate metaplastic synapse characteristics in the context of high retention and reception of information. Inherent behavior of a memristor emulating the multistate synapse is employed to capture the metaplastic behavior. An integrated neural network study for learning and memory retention is performed by integrating the synapse in a $5\times3$ crossbar at the circuit level and $128\times128$ network at the architectural level. An on-device training circuitry ensures the dynamic learning in the network. In the $128\times128$ network, it is observed that the number of input patterns the multistate synapse can classify is $\simeq$ 2.1x that of a simple binary synapse model, at a mean accuracy of $\geq$ 75% .
更多查看译文
关键词
multistate memristor synaptic networks
AI 理解论文
溯源树
样例
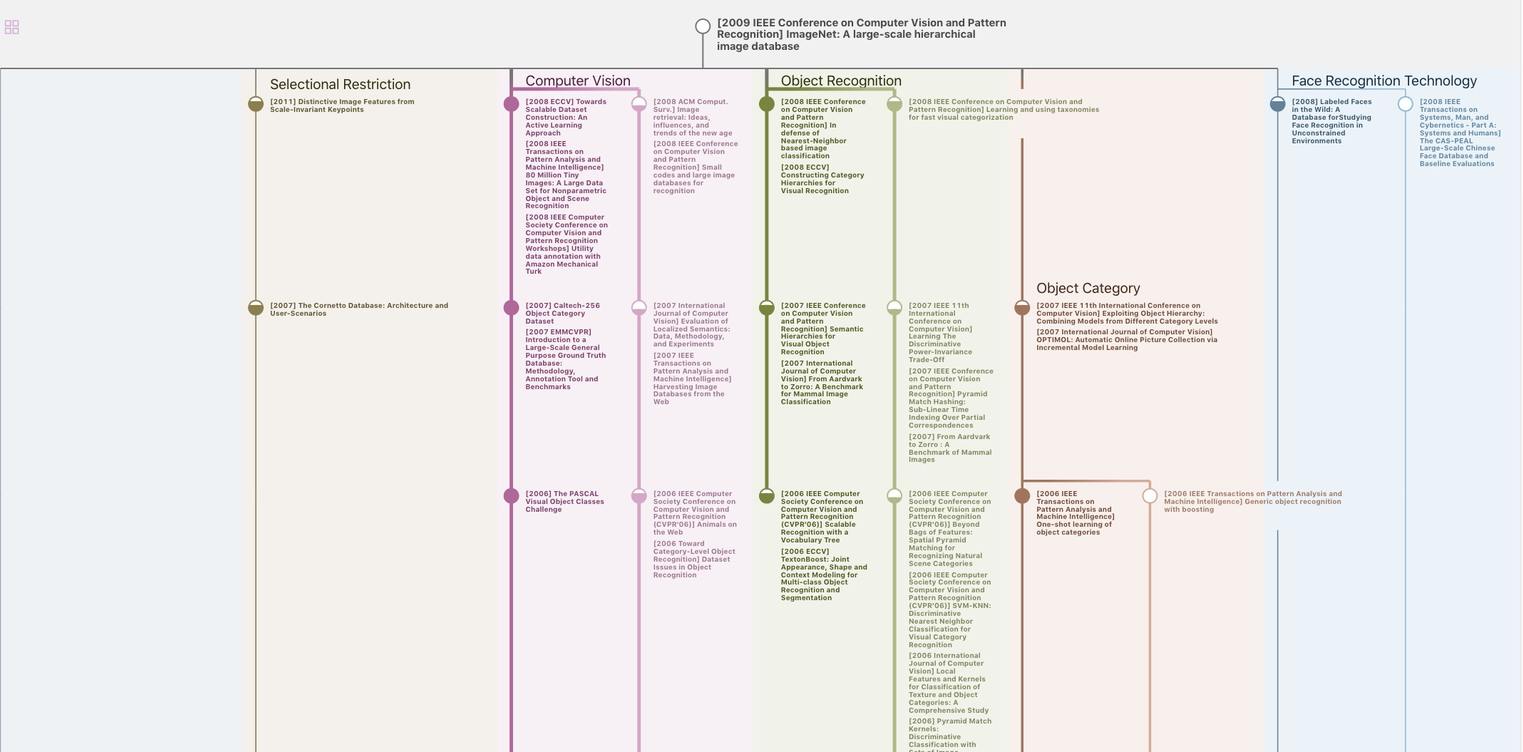
生成溯源树,研究论文发展脉络
Chat Paper
正在生成论文摘要